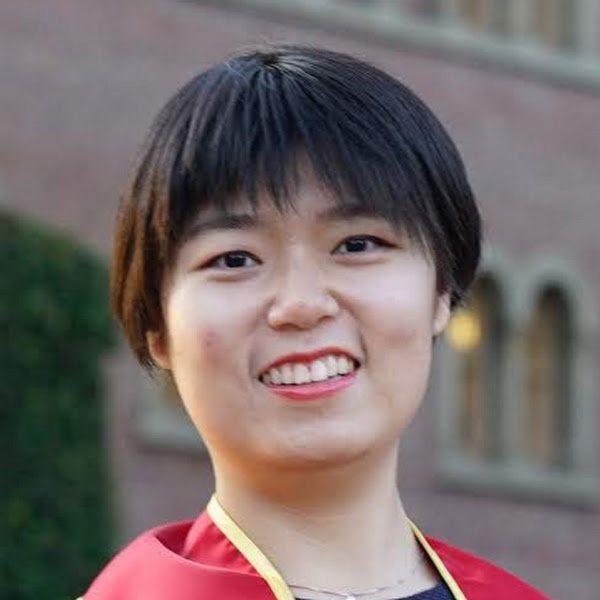
Jie Ren
I am a research scientist in Google Brain. My recent research interest is robust and reliable deep learning, with a focus on uncertainty quantification and out-of-distribution detection. I received my PhD in Computational Biology and Bioinformatics and a MSc in Statistics from the University of Southern California, 2017. I was a post-doctoral fellow at USC from 2017-2018, and after that I joined Google Brain as an AI resident for one year. Please see my webpage for more information https://jessieren.github.io/.
Authored Publications
Sort By
Building One-class Detector for Anything: Open-vocabulary Zero-shot OOD Detection Using Text-image Models
Yunhao Ge
Jiaping Zhao
Laurent Itti
Knowledge and Logical Reasoning workshop @ ICML(2023)
Preview abstract
We focus on the challenge of out-of-distribution (OOD) detection in deep learning models, a crucial aspect in ensuring reliability. Despite considerable effort, the problem remains significantly challenging in deep learning models due to their propensity to output over-confident predictions for OOD inputs. We propose a novel one-class open-set OOD detector that leverages text-image pre-trained models in a zero-shot fashion and incorporates various descriptions of in-domain and OOD. Our approach is designed to detect anything not in-domain and offers the flexibility to detect a wide variety of OOD, defined via fine- or coarse-grained labels, or even in natural language. We evaluate our approach on challenging benchmarks including large-scale datasets containing fine-grained, semantically similar classes, distributionally shifted images, and multi-object images containing a mixture of in-domain and OOD objects. Our method shows superior performance over previous methods on all benchmarks.
View details
Morse Neural Networks for Uncertainty Quantification
Clara Huiyi Hu
ICML 2023 Workshop on Structured Probabilistic Inference & Generative Modeling(2023)
Preview abstract
We introduce a new deep generative model useful for uncertainty quantification: the Morse neural network, which generalizes the unnormalized Gaussian densities to have modes of high-dimensional submanifolds instead of just discrete points. Fitting the Morse neural network via a KL-divergence loss yields 1) a (unnormalized) generative density, 2) an OOD detector, 3) a calibration temperature, 4) a generative sampler, along with in the supervised case 6) a distance aware-classifier. The Morse network can be used on top of a pre-trained network to bring distance-aware calibration w.r.t the training data. Because of its versatility, the Morse neural networks unifies many techniques: e.g., the Entropic Out-of-Distribution Detector of (Macêdo et al., 2021) inOOD detection, the one class Deep Support Vector Description method of (Ruff et al., 2018) in anomaly detection, or the Contrastive One Class classifier in continuous learning (Sun et al., 2021).The Morse neural network has connections to sup-port vector machines, kernel methods, and Morse theory in topology.
View details
A Simple Approach to Improve Single-Model Deep Uncertainty via Distance-Awareness
Shreyas Padhy
Zi Lin
Yeming Wen
Ghassen Jerfel
Journal of Machine Learning Research(2022)
Preview abstract
Accurate uncertainty quantification is a major challenge in deep learning, as neural networks can make overconfident errors and assign high confidence predictions to out-of-distribution (OOD) inputs. The most popular approaches to estimate predictive uncertainty in deep learning are methods that combine predictions from multiple neural networks, such as Bayesian neural networks (BNNs) and deep ensembles. However their practicality in real-time, industrial-scale applications are limited due to the high memory and computational cost. Furthermore, ensembles and BNNs do not necessarily fix all the issues with the underlying member networks. In this work, we study principled approaches to improve uncertainty property of a single network, based on a single, deterministic representation. By formalizing the uncertainty quantification as a minimax learning problem, we first identify distance awareness, i.e., the model's ability to quantify the distance of a testing example from the training data, as a necessary condition for a DNN to achieve high-quality (i.e., minimax optimal) uncertainty estimation. We then propose Spectral-normalized Neural Gaussian Process (SNGP), a simple method that improves the distance-awareness ability of modern DNNs with two simple changes: (1) applying spectral normalization to hidden weights to enforce bi-Lipschitz smoothness in representations and (2) replacing the last output layer with a Gaussian process layer. On a suite of vision and language understanding benchmarks, SNGP outperforms other single-model approaches in prediction, calibration and out-of-domain detection. Furthermore, SNGP provides complementary benefits to popular techniques such as deep ensembles and data augmentation, making it a simple and scalable building block for probabilistic deep learning. Code is open-sourced at https://github.com/google/uncertainty-baselines.
View details
Plex: Towards Reliability using Pretrained Large Model Extensions
Du Phan
Mark Patrick Collier
Zi Wang
Zelda Mariet
Clara Huiyi Hu
Neil Band
Tim G. J. Rudner
Karan Singhal
Joost van Amersfoort
Andreas Christian Kirsch
Rodolphe Jenatton
Honglin Yuan
Kelly Buchanan
Yarin Gal
ICML 2022 Pre-training Workshop(2022)
Preview abstract
A recent trend in artificial intelligence (AI) is the use of pretrained models for language and vision tasks, which has achieved extraordinary performance but also puzzling failures. Examining tasks that probe the model’s abilities in diverse ways is therefore critical to the field. In this paper, we explore the \emph{reliability} of models, where we define a reliable model as one that not only achieves strong predictive performance but also performs well consistently over many decision-making tasks such as uncertainty (e.g., selective prediction, open set recognition), robust generalization (e.g., accuracy and scoring rules such as log-likelihood on in- and out-of-distribution datasets), and adaptation (e.g., active learning, few-shot learning). We devise 11 types of tasks over 36 datasets in order to evaluate different aspects of reliability on both vision and language domains. To improve reliability, we developed ViT-Plex and T5-Plex, \emph{p}retrained \emph{l}arge-model \emph{ex}tensions (henceforth abbreviated as \emph{plex}) for vision and language modalities. Plex greatly improves the state-of-the-art across tasks, and as a pretrained model Plex unifies the traditional protocol of designing and tuning one model for each reliability task. We demonstrate scaling effects over model sizes and pretraining dataset sizes up to 4 billion examples. We also demonstrate Plex’s capabilities on new tasks including zero-shot open set recognition, few-shot uncertainty, and uncertainty in conversational language understanding.
View details
Does Your Dermatology Classifier Know What It Doesn't Know? Detecting the Long-Tail of Unseen Conditions
Aaron Loh
Basil Mustafa
Nick Pawlowski
Jan Freyberg
Zach William Beaver
Nam Vo
Peggy Bui
Samantha Winter
Patricia MacWilliams
Umesh Telang
Taylan Cemgil
Medical Imaging Analysis(2021)
Preview abstract
Supervised deep learning models have proven to be highly effective in classification of dermatological conditions. These models rely on the availability of abundant labeled training examples. However, in the real world, many dermatological conditions are individually too infrequent for per-condition classification with supervised learning. Although individually infrequent, these conditions may collectively be common and therefore are clinically significant in aggregate. To avoid models generating erroneous outputs on such examples, there remains a considerable unmet need for deep learning systems that can better detect such infrequent conditions. These infrequent `outlier' conditions are seen very rarely (or not at all) during training. In this paper, we frame this task as an out-of-distribution (OOD) detection problem. We set up a benchmark ensuring that outlier conditions are disjoint between model train, validation, and test sets. Unlike most traditional OOD benchmarks which detect dataset distribution shift, we aim at detecting semantic differences, often referred to as near-OOD detection which is a more difficult task. We propose a novel hierarchical outlier detection (HOD) approach, which assigns multiple abstention classes for each training outlier class and jointly performs a coarse classification of inliers \vs{} outliers, along with fine-grained classification of the individual classes. We demonstrate that the proposed HOD outperforms existing techniques for outlier exposure based OOD detection. We also use different state-of-the-art representation learning approaches (BiT-JFT, SimCLR, MICLe) to improve OOD performance and demonstrate the effectiveness of HOD loss for them.
Further, we explore different ensembling strategies for OOD detection and propose a diverse ensemble selection process for the best result. We also performed a subgroup analysis over conditions of varying risk levels and different skin types to investigate how OOD performance changes over each subgroup and demonstrated the gains of our framework in comparison to baselines. Furthermore, we go beyond traditional performance metrics and introduce a cost metric to approximate downstream clinical impact. We used this cost metric to compare the proposed method against the baseline, thereby making a stronger case for its effectiveness in real-world deployment scenarios.
View details
Preview abstract
Near out-of-distribution detection (OOD) is a major challenge for deep neural networks. We demonstrate that large-scale pre-training can significantly improve the state-of-the-art (SOTA) on a range of near OOD tasks across different data modalities. For instance, on CIFAR-100 vs CIFAR-10 OOD detection, we improve the AUROC from 85% (current SOTA) to more than 96% using Vision Transformers pre-trained on ImageNet21k. On a challenging genomics OOD detection benchmark, we improve the AUROC from 66% (current SOTA) to 77%. To further improve performance, we explore the few-shot outlier exposure setting where a few examples from outlier classes may be available; we show that pre-trained models are well-suited to outlier exposure, and that the AUROC of OOD detection on CIFAR-100 vs CIFAR-10 can be improved to 98.7% with just 1 image per OOD class, and 99.46% with 10 images per OOD class. We observe similar trends on genomics, achieving 85% with just 1 example per OOD class. For multi-modal image-text pre-trained models such as CLIP, we explore a new way of using just the names of outlier classes as a sole source of information (without any accompanying images) and show that this outperforms previous SOTA on several standard OOD benchmark tasks.
View details
Revisiting One-vs-All Classifiers for Predictive Uncertainty and Out-of-Distribution Detection in Neural Networks
Shreyas Padhy
(2020)
Preview abstract
Accurate estimation of predictive uncertainty in modern neural networks is critical to achieve well calibrated predictions and detect out-of-distribution inputs. The most promising approaches have been predominantly focused on improving model uncertainty (e.g. deep ensembles and Bayesian neural networks) and post-processing techniques for out-of-distribution detection (e.g. ODIN and Mahalanobis distance). However, there has been relatively little investigation into how the parametrization of the probabilities in discriminative classifiers affects the uncertainty estimates, and the dominant method, softmax cross-entropy, results in misleadingly high confidences on out-of-distribution data and under covariate shift. We investigate alternative ways of formulating probabilities using (1) a one-vs-all formulation to capture the notion of “none of the above”, and (2) a distance-based logit representation to encode uncertainty as a function of distance to the training manifold. We show that one-vs-all formulations can match the predictive performance of softmax without incurring any additional training or test-time complexity, and improve calibration on image classification tasks.
View details
Likelihood Ratios for Out-of-Distribution Detection
Peter J. Liu
Mark DePristo
Josh Dillon
arXiv preprint arXiv:1906.02845(2019)
Preview abstract
Discriminative neural networks offer little or no performance guarantees when deployed on data not generated by the same process as the training distribution. On such out-of-distribution (OOD) inputs, the prediction may not only be erroneous, but confidently so, limiting the safe deployment of classifiers in real-world applications. One such challenging application is bacteria identification based on genomic sequences, which holds the promise of early detection of diseases, but requires a model that can output low confidence predictions on OOD genomic sequences from new bacteria that were not present in the training data. We introduce a genomics dataset for OOD detection that allows other researchers to benchmark progress on this important problem. We investigate deep generative model based approaches for OOD detection and observe that the likelihood score is heavily affected by population level background statistics. We propose a likelihood ratio method for deep generative models which effectively corrects for these confounding background statistics. We benchmark the OOD detection performance of the proposed method against existing approaches on the genomics dataset and show that our method achieves state-of-the-art performance. We demonstrate the generality of the proposed method by showing that it significantly improves OOD detection when applied to deep generative models of images.
View details
Preview abstract
We propose an end-to-end neural model for zero-shot abstractive text summarization of paragraphs, and introduce a benchmark task, ROCSumm, based on ROCStories, a subset for which we collected human summaries. In this task, five-sentence stories (paragraphs) are summarized with one sentence, using human summaries only for evaluation. We show results for extractive and human baselines to demonstrate a large abstractive gap in performance. Our model, SummAE, consists of a denoising auto-encoder that embeds sentences and paragraphs in a common space, from which either can be decoded. Summaries for paragraphs are generated by decoding a sentence from the paragraph representations. We find that traditional sequence-to-sequence auto-encoders fail to produce good summaries and describe how specific architectural choices and pre-training techniques can significantly improve performance, outperforming extractive baselines. The data, training, evaluation code, and best model weights are open-sourced.
View details
Can You Trust Your Model’s Uncertainty? Evaluating Predictive Uncertainty Under Dataset Shift
Yaniv Ovadia
Sebastian Nowozin
Josh Dillon
Advances in Neural Information Processing Systems(2019)
Preview abstract
Modern machine learning methods including deep learning have achieved great success in predictive accuracy for supervised learning tasks, but may still fall short in giving useful estimates of their predictive {\em uncertainty}. Quantifying uncertainty is especially critical in real-world settings, which often involve distributions that are skewed from the training distribution due to a variety of factors including sample bias and non-stationarity. In such settings, well calibrated uncertainty estimates convey information about when a model's output should (or should not) be trusted. Many probabilistic deep learning methods, including Bayesian-and non-Bayesian methods, have been proposed in the literature for quantifying predictive uncertainty, but to our knowledge there has not previously been a rigorous large-scale empirical comparison of these methods under conditions of distributional skew. We present a large-scale benchmark of existing state-of-the-art methods on classification problems and investigate the effect of distributional skew on accuracy and calibration. We find that traditional post-hoc calibration falls short and some Bayesian methods are intractable for very large data. However, methods that marginalize over models give surprisingly strong results across a broad spectrum.
View details