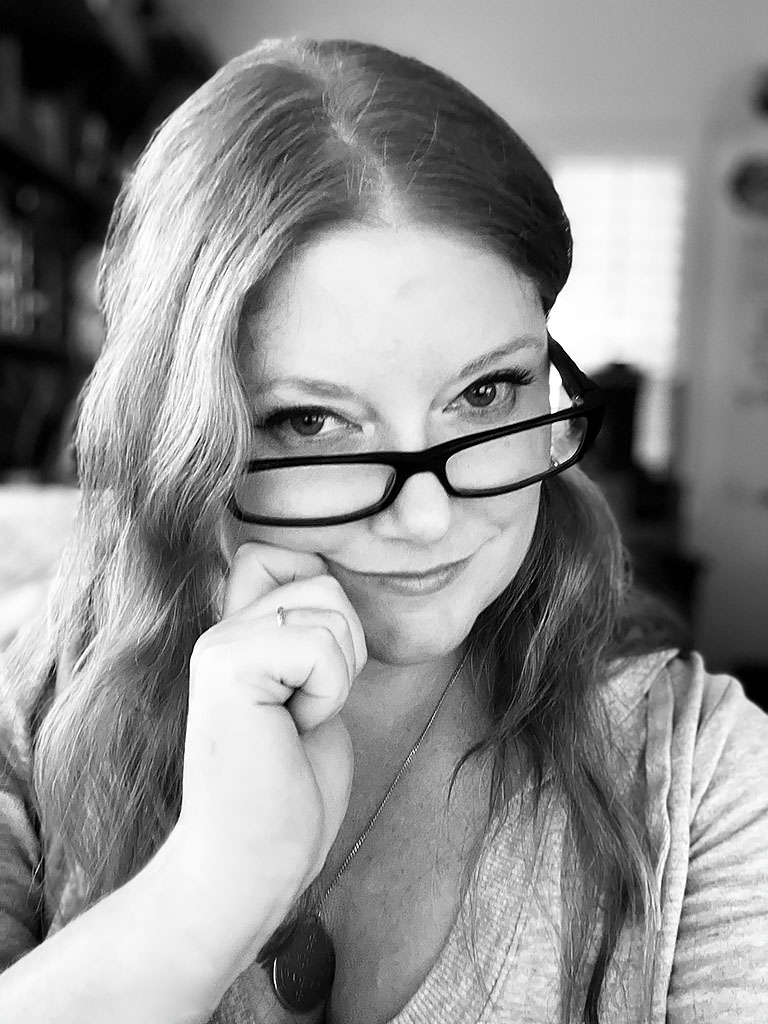
Jay Busch
Jay Busch has been working in the field of digital humans and computer graphics for over 10 years. A large portion of her work is in the capture and creation of digital doubles for movies, video games and simulation at USC Institute for Creative Technologies. Graduating from the Art Institute of Santa Monica with a B.S. in Media Arts and Animation, she often enjoys exploring the convergence of art and technology in the areas of creative and educational fields. She currently works for Google Daydream VR as a Technical Program Manager in the areas of hardware and technical art.
Authored Publications
Sort By
Learning Illumination from Diverse Portraits
Wan-Chun Alex Ma
Christoph Rhemann
Jason Dourgarian
Paul Debevec
SIGGRAPH Asia 2020 Technical Communications (2020)
Preview abstract
We present a learning-based technique for estimating high dynamic range (HDR), omnidirectional illumination from a single low dynamic range (LDR) portrait image captured under arbitrary indoor or outdoor lighting conditions. We train our model using portrait photos paired with their ground truth illumination. We generate a rich set of such photos by using a light stage to record the reflectance field and alpha matte of 70 diverse subjects in various expressions. We then relight the subjects using image-based relighting with a database of one million HDR lighting environments, compositing them onto paired high-resolution background imagery recorded during the lighting acquisition. We train the lighting estimation model using rendering-based loss functions and add a multi-scale adversarial loss to estimate plausible high frequency lighting detail. We show that our technique outperforms the state-of-the-art technique for portrait-based lighting estimation, and we also show that our method reliably handles the inherent ambiguity between overall lighting strength and surface albedo, recovering a similar scale of illumination for subjects with diverse skin tones. Our method allows virtual objects and digital characters to be added to a portrait photograph with consistent illumination. As our inference runs in real-time on a smartphone, we enable realistic rendering and compositing of virtual objects into live video for augmented reality.
View details
DeepLight: Learning Illumination for Unconstrained Mobile Mixed Reality
Graham Fyffe
John Flynn
Laurent Charbonnel
Paul Debevec
Wan-Chun Alex Ma
Proceedings of the IEEE/CVF Conference on Computer Vision and Pattern Recognition (2019), pp. 5918-5928
Preview abstract
We present a learning-based method to infer plausible high dynamic range (HDR), omnidirectional illumination given an unconstrained, low dynamic range (LDR) image from a mobile phone camera with a limited field-of-view (FOV). For training data, we collect videos of various reflective spheres placed within the camera's FOV, but with most of the background unoccluded, leveraging that materials with diverse reflectance functions will reveal different lighting cues in a single exposure. We train a deep neural network to regress from the unoccluded part of the LDR background image to its HDR lighting by matching the LDR ground truth sphere images to those rendered with the predicted illumination using image-based relighting, which is differentiable. Our inference runs in real-time on a mobile device, enabling realistic rendering of virtual objects into real scenes for mobile mixed reality. Training on automatically exposed and white-balanced videos, we improve the realism of rendered objects compared to the state-of-the art methods for both indoor and outdoor scenes.
View details
The Relightables: Volumetric Performance Capture of Humans with Realistic Relighting
Kaiwen Guo
Peter Lincoln
Philip Davidson
Xueming Yu
Matt Whalen
Geoff Harvey
Jason Dourgarian
Danhang Tang
Anastasia Tkach
Emily Cooper
Mingsong Dou
Graham Fyffe
Christoph Rhemann
Jonathan Taylor
Paul Debevec
Shahram Izadi
SIGGRAPH Asia (2019) (to appear)
Preview abstract
We present ''The Relightables'', a volumetric capture system for photorealistic and high quality relightable full-body performance capture. While significant progress has been made on volumetric capture systems, focusing on 3D geometric reconstruction with high resolution textures, much less work has been done to recover photometric properties needed for relighting. Results from such systems lack high-frequency details and the subject's shading is prebaked into the texture. In contrast, a large body of work has addressed relightable acquisition for image-based approaches, which photograph the subject under a set of basis lighting conditions and recombine the images to show the subject as they would appear in a target lighting environment. However, to date, these approaches have not been adapted for use in the context of a high-resolution volumetric capture system. Our method combines this ability to realistically relight humans for arbitrary environments, with the benefits of free-viewpoint volumetric capture and new levels of geometric accuracy for dynamic performances. Our subjects are recorded inside a custom geodesic sphere outfitted with 331 custom color LED lights, an array of high-resolution cameras, and a set of custom high-resolution depth sensors. Our system innovates in multiple areas: First, we designed a novel active depth sensor to capture 12.4MP depth maps, which we describe in detail. Second, we show how to design a hybrid geometric and machine learning reconstruction pipeline to process the high resolution input and output a volumetric video. Third, we generate temporally consistent reflectance maps for dynamic performers by leveraging the information contained in two alternating color gradient illumination images acquired at 60Hz. Multiple experiments, comparisons, and applications show that The Relightables significantly improves upon the level of realism in placing volumetrically captured human performances into arbitrary CG scenes.
View details
Deep Reflectance Fields - High-Quality Facial Reflectance Field Inference from Color Gradient Illumination
Abhi Meka
Christian Haene
Michael Zollhöfer
Graham Fyffe
Xueming Yu
Jason Dourgarian
Peter Denny
Sofien Bouaziz
Peter Lincoln
Matt Whalen
Geoff Harvey
Jonathan Taylor
Shahram Izadi
Paul Debevec
Christian Theobalt
Julien Valentin
Christoph Rhemann
SIGGRAPH (2019)
Preview abstract
Photo-realistic relighting of human faces is a highly sought after feature with many applications ranging from visual effects to truly immersive virtual experiences. Despite tremendous technological advances in the field, humans are often capable of distinguishing real faces from synthetic renders. Photo-realistically relighting any human face is indeed a challenge with many difficulties going from modelling sub-surface scattering and blood flow to estimating the interaction between light and individual strands of hair. We introduce the first system that combines the ability to deal with dynamic performances to the realism of 4D reflectance fields, enabling photo-realistic relighting of non-static faces. The core of our method consists of a Deep Neural network that is able to predict full 4D reflectance fields from two images captured under spherical gradient illumination. Extensive experiments not only show that two images under spherical gradient illumination can be easily captured in real time, but also that these particular images contain all the information needed to estimate the full reflectance field, including specularities and high frequency details. Finally, side by side comparisons demonstrate that the proposed system outperforms the current state-of-the-art in terms of realism and speed.
View details
Single Image Portrait Relighting
Christoph Rhemann
Graham Fyffe
Paul Debevec
Ravi Ramamoorthi
Tiancheng Sun
Xueming Yu
Yun-Ta Tsai
Zexiang Xu
SIGGRAPH (2019)
Preview abstract
Lighting plays a central role in conveying the essence and depth of the subject in a 2D portrait photograph. Professional photographers will carefully control the lighting in their studio to manipulate the appearance of their subject, while consumer photographers are usually constrained to the illumination of their environment. Though prior works have explored techniques for relighting an image, their utility is usually limited due to requirements of specialized hardware, multiple images of the subject under controlled or known illuminations, or accurate models of geometry and reflectance. takes as input a single RGB image of a portrait taken with a standard cellphone camera in an unconstrained environment, and from that image produces a relit image of that subject as though it were illuminated according to any provided environment map. Our proposed technique produces quantitatively superior results on our dataset's validation set compared to prior work, and produces convincing qualitative relighting results on a dataset of hundreds of real-world cellphone portraits. Because our technique can produce a 640 x 640 image in only 160 milliseconds, it may enable interactive user-facing photographic applications in the future.
View details