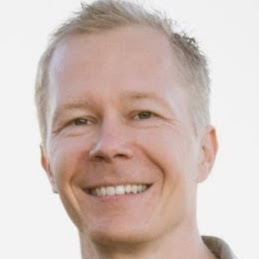
Janne Kontkanen
Janne Kontkanen works on machine learning, computer vision and graphics algorithms in Google’s augmented perception team. Previously he worked on Google Earth rendering technology and in the film industry. He has a PhD in computer graphics from the Aalto University (former Helsinki University of Technology) and received an academy award for his deep compositing work in DreamWorks Animation.
Research Areas
Authored Publications
Sort By
Google
Generative Powers of Ten
Xiaojuan Wang
Steve Seitz
Ben Mildenhall
Pratul Srinivasan
Dor Verbin
Aleksander Hołyński
2024
3D Moments from Near Duplicate Photos
Qianqian Wang
Zhengqi Li
Conference on Computer Vision and Pattern Recognition (CVPR) (2022)
FILM: Frame Interpolation for Large Motion
Fitsum Reda
Eric Tabellion
Proceedings of the European conference on computer vision (ECCV) (2022)
Removing an object and its shadows from a photograph
Edward Zhang
Ricardo Martin-Brualla
IEEE/CVF Conference on Computer Vision and Pattern Recognition (CVPR) (2021)
Multi-view Image Fusion
Marc Comino Trinidad
Ricardo Martin Brualla
Florian Kainz
International Conference on Computer Vision (ICCV) (2019) (to appear)
Jump: Virtual Reality Video
Robert Anderson
Carlos Hernandez Esteban
Steven M. Seitz
SIGGRAPH Asia (2016)