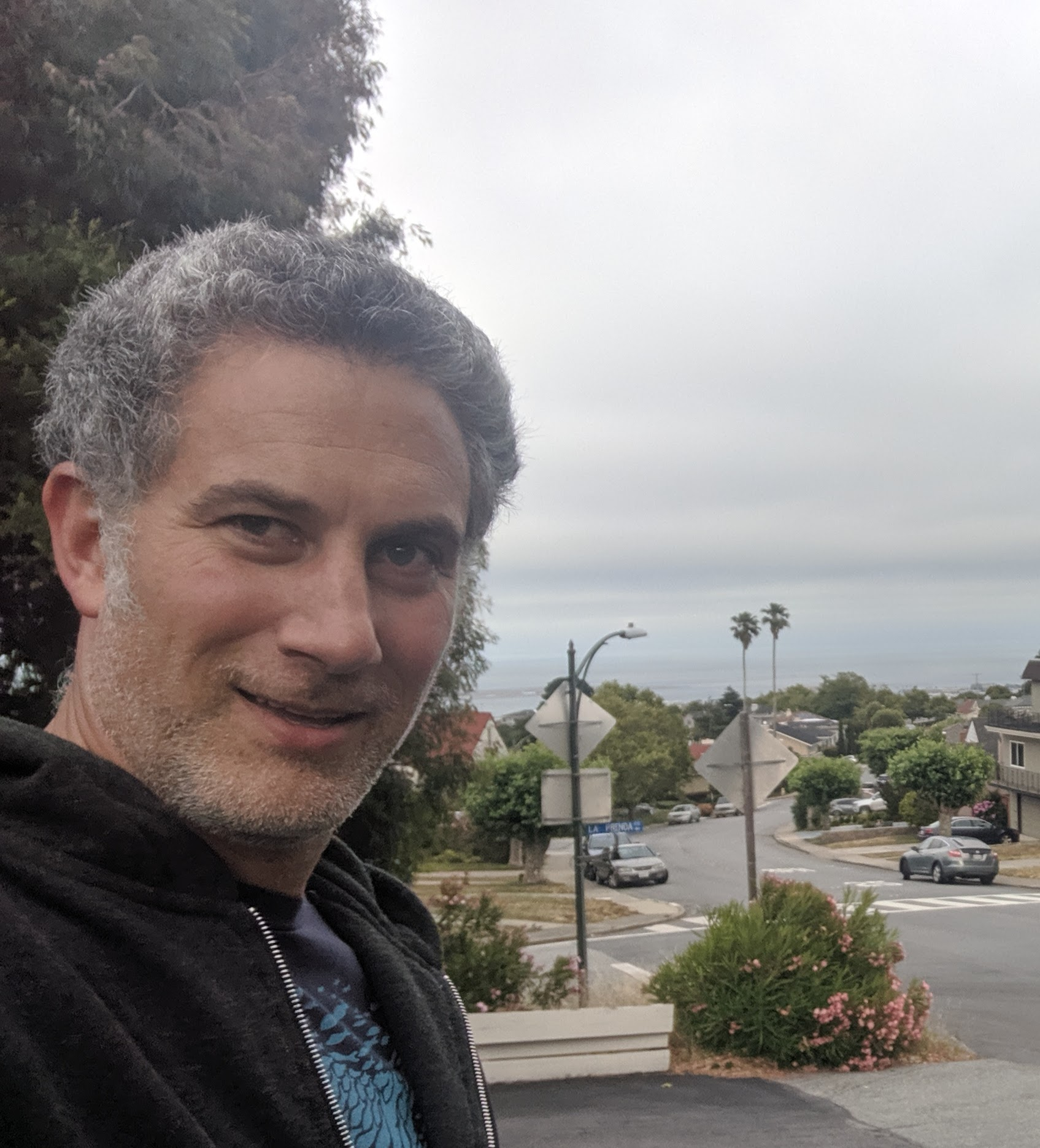
Ian Langmore
At Google, Ian first worked on Bayesian modeling for local search algorithms before moving on to probabilistic methods in fusion plasma reconstruction. As of 2023, he works on probabilistic weather prediction using differentiable computing. This places him at the intersection of applied probability, software engineering, and physics.
Research Areas
Authored Publications
Sort By
A scalable system to measure contrail formation on a per-flight basis
Erica Brand
Sebastian Eastham
Carl Elkin
Thomas Dean
Zebediah Engberg
Ulrike Hager
Joe Ng
Dinesh Sanekommu
Marc Shapiro
Environmental Research Communications (2024)
Preview abstract
In this work we describe a scalable, automated system to determine from satellite data whether a given flight has made a persistent contrail.
The system works by comparing flight segments to contrails detected by a computer vision algorithm running on images from the GOES-16 Advanced Baseline Imager. We develop a `flight matching' algorithm and use it to label each flight segment as a `match' or `non-match'. We perform this analysis on 1.6 million flight segments and compare these labels to existing contrail prediction methods based on weather forecast data. The result is an analysis of which flights make persistent contrails several orders of magnitude larger than any previous work. We find that current contrail prediction models fail to correctly predict whether we will match a contrail in many cases.
View details
Neural general circulation models for weather and climate
Dmitrii Kochkov
Janni Yuval
Jamie Smith
Griffin Mooers
Milan Kloewer
James Lottes
Peter Dueben
Samuel Hatfield
Peter Battaglia
Alvaro Sanchez
Matthew Willson
Nature, 632 (2024), pp. 1060-1066
Preview abstract
General circulation models (GCMs) are the foundation of weather and climate prediction. GCMs are physics-based simulators that combine a numerical solver for large-scale dynamics with tuned representations for small-scale processes such as cloud formation. Recently, machine-learning models trained on reanalysis data have achieved comparable or better skill than GCMs for deterministic weather forecasting. However, these models have not demonstrated improved ensemble forecasts, or shown sufficient stability for long-term weather and climate simulations. Here we present a GCM that combines a differentiable solver for atmospheric dynamics with machine-learning components and show that it can generate forecasts of deterministic weather, ensemble weather and climate on par with the best machine-learning and physics-based methods. NeuralGCM is competitive with machine-learning models for one- to ten-day forecasts, and with the European Centre for Medium-Range Weather Forecasts ensemble prediction for one- to fifteen-day forecasts. With prescribed sea surface temperature, NeuralGCM can accurately track climate metrics for multiple decades, and climate forecasts with 140-kilometre resolution show emergent phenomena such as realistic frequency and trajectories of tropical cyclones. For both weather and climate, our approach offers orders of magnitude computational savings over conventional GCMs, although our model does not extrapolate to substantially different future climates. Our results show that end-to-end deep learning is compatible with tasks performed by conventional GCMs and can enhance the large-scale physical simulations that are essential for understanding and predicting the Earth system.
View details
WeatherBench 2: A benchmark for the next generation of data-driven global weather models
Alex Merose
Peter Battaglia
Tyler Russell
Alvaro Sanchez
Vivian Yang
Matthew Chantry
Zied Ben Bouallegue
Peter Dueben
Carla Bromberg
Jared Sisk
Luke Barrington
Aaron Bell
arXiv (2023) (to appear)
Preview abstract
WeatherBench 2 is an update to the global, medium-range (1-14 day) weather forecasting benchmark proposed by Rasp et al. (2020), designed with the aim to accelerate progress in data-driven weather modeling. WeatherBench 2 consists of an open-source evaluation framework, publicly available training, ground truth and baseline data as well as a continuously updated website with the latest metrics and state-of-the-art models: https://sites.research.google/weatherbench. This paper describes the design principles of the evaluation framework and presents results for current state-of-the-art physical and data-driven weather models. The metrics are based on established practices for evaluating weather forecasts at leading operational weather centers. We define a set of headline scores to provide an overview of model performance. In addition, we also discuss caveats in the current evaluation setup and challenges for the future of data-driven weather forecasting.
View details
Preview abstract
Hamiltonian Monte Carlo is discussed in the context of a fusion plasma reconstruction. Ill conditioned covariance and multi-modality are discussed in depth.
View details
Preview abstract
The Hamiltonian Monte Carlo (HMC) method allows sampling from continuous densities. Favorable scaling with dimension has led to wide adoption of HMC by the statistics community. Modern auto-differentiating software should allow more widespread usage in Bayesian inverse problems. This paper analyzes the two major difficulties encountered using HMC for inverse problems: poor conditioning and multi-modality. Novel results on preconditioning and replica exchange Monte Carlo parameter selection are presented in the context of spectroscopy. Recommendations are analyzed rigorously in the Gaussian case, and shown to generalize in a fusion plasma reconstruction.
View details
Multi-instrument Bayesian reconstruction of plasma shape evolution in C-2W experiment
Erik Trask
Hiroshi Gota
Jesus Romero
Rob von Behren
Tom Madams
Physics of Plasmas (2021)
Preview abstract
We determined the time-dependent geometry including high-frequency oscillations of the plasma density in TAE’s C2W experiment. This was done as a joint Bayesian reconstruction from a 14-chord FIR interferometer in the midplane, 32 Mirnov probes at the periphery, and 8 shine-through detectors at the targets of the neutral beams. For each point in time we recovered, with credibility intervals: the radial density profile of the plasma; bulk plasma displacement; amplitudes, frequencies and phases of the azimuthal modes n=1 to n=4. Also reconstructed were the radial profiles of the deformations associated with each of the azimuthal modes. Bayesian posterior sampling was done via Hamiltonian
Monte Carlo with custom preconditioning. This gave us a comprehensive uncertainty quantification of the reconstructed values, including correlations and some understanding of multimodal posteriors. This method was applied to thousands of experimental shots on C-2W, producing a rich data set for analysis of plasma performance.
View details
OVERVIEW OF C-2W: HIGH TEMPERATURE, STEADY-STATE BEAM-DRIVEN FIELD-REVERSED CONFIGURATION PLASMAS
Rob von Behren
TAE
Tom Madams
William D Heavlin
Nuclear Fusion (2021)
Preview abstract
TAE Technologies, Inc. (TAE) is pursuing an alternative approach to magnetically confined fusion, which relies on field-reversed configuration (FRC) plasmas composed of mostly energetic and well-confined particles by means of a state-of-the-art tunable energy neutral-beam (NB) injector system. TAE’s current experimental device, C-2W (also called “Norman”), is the world’s largest compact-toroid device and has made significant progress in FRC performance, producing record breaking, high temperature (electron temperature, Te >500 eV; total electron and ion temperature, Ttot >3 keV) advanced beam-driven FRC plasmas, dominated by injected fast particles and sustained in steady-state for up to 30 ms, which is limited by NB pulse duration. C-2W produces significantly better FRC performance than the preceding C-2U experiment, in part due to Google’s machine-learning framework for experimental optimization, which has contributed to the discovery of a new operational regime where novel settings for the formation sections yield consistently reproducible, hot, and stable plasmas. Active plasma control system has been developed and utilized in C-2W to produce consistent FRC performance as well as for reliable machine operations using magnets, electrodes, gas injection, and tunable NBs. The active control system has demonstrated a stabilization of FRC axial instability. Overall FRC performance is well correlated with NBs and edge-biasing system, where higher total plasma energy is obtained with increasing both NB injection power and applied-voltage on biasing electrodes. C-2W divertors have demonstrated a good electron heat confinement on open-field-lines using strong magnetic mirror fields as well as expanding the magnetic field in the divertors (expansion ratio >30); the electron energy lost per ion, ~6–8, is achieved, which is close to the ideal theoretical minimum.
View details
Fusion Plasma Reconstruction
Nathan Neibauer
Rob von Behren
(2019)
Preview abstract
Fusion Plasma Reconstruction work done at Google in partnership with TAE is presented.
View details
Preview abstract
Hamiltonian Monte Carlo is a popular sampling technique for smooth target densities. The scale lengths of the target have long been known to influence integration error and sampling efficiency. However, quantitative measures intrinsic to the target have been lacking. In this paper, we restrict attention to the multivariate Gaussian and the leapfrog integrator, and obtain a condition number corresponding to sampling efficiency. This number, based on the spectral and Schatten norms, quantifies the number of leapfrog steps needed to efficiently sample. We demonstrate its utility by using this condition number to analyze HMC preconditioning techniques. We also find the condition number of large inverse Wishart matrices, from which we derive burn-in heuristics.
View details
NeuTra-lizing Bad Geometry in Hamiltonian Monte Carlo Using Neural Transport
Matthew D. Hoffman
Josh Dillon
Srinivas Vasudevan
arXiv preprint (2019)
Preview abstract
Hamiltonian Monte Carlo is a powerful algorithm for sampling from difficult-to-normalize posterior distributions. However, when the geometry of the posterior is unfavorable, it may take many expensive evaluations of the target distribution and its gradient to converge and mix. We propose neural transport (NeuTra) HMC, a technique for learning to correct this sort of unfavorable geometry using inverse autoregressive flows (IAF), a powerful neural variational inference technique. The IAF is trained to minimize the KL divergence from an isotropic Gaussian to the warped posterior, and then HMC sampling is performed in the warped space. We evaluate NeuTra HMC on a variety of synthetic and real problems, and find that it significantly outperforms vanilla HMC both in time to reach the stationary distribution and asymptotic effective-sample-size rates.
View details