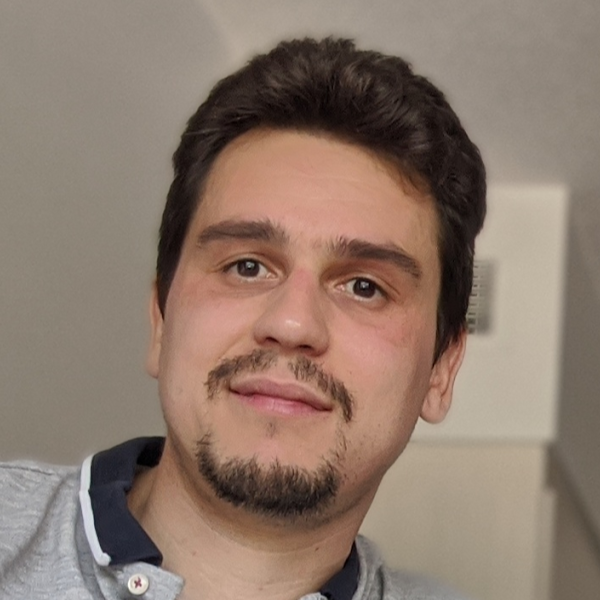
Fabian Pedregosa
I am a research scientist in the Brain group in Montreal. My main area of expertise is optimization. My previous work includes analysis and development of parallel stochastic methods, the development of more practical methods for optimizing non-smooth functions and new methods for smooth hyperparameter optimization. More broadly, I'm interested in optimization, machine learning and scientific software.
I am also one of the founders of the scikit-learn machine learning library, of which I have been core developer and maintainer from 2010 to 2012, and I have been an active contributor to many open source projects including the computer algebra system sympy and the python profiler memory-profiler.
My current editorial involvement includes being the managing editor of the journal of machine learning research (JMLR) and reviewer for the conferences NIPS and ICML and the journals Mathematical Programming, JMLR.
Finally, I maintain a blog about my research at http://fa.bianp.net.