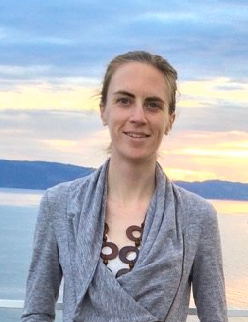
Emily Fertig
I joined the residency after working as a technical consultant and data scientist in the electricity industry, where I supported power grid operators and policymakers on decisions related to the integration of renewable energy and emerging technologies. Prior to working in industry, I completed my Ph.D. in Engineering and Public Policy at CMU, where I researched quantitative methods for decision-making under uncertainty in climate and energy policy. My work in energy increasingly used methods from machine learning, which led me to have a greater interest in AI and its broader implications for society. The residency gave me an exciting opportunity to switch research fields while pursuing my core interests of decision-making under uncertainty and technology policy, and I am currently working on quantifying uncertainty in the predictions of deep neural networks. I hope to build a research program that better characterizes and systematizes uncertainty in deep learning, and develops methods that help enable safer, more reliable AI systems. The residency has been a great experience -- I’ve learned so much in the short time that I’ve been here, I really value the freedom to direct my own research, and it’s a privilege to collaborate with and learn from some of the best researchers in the field.
Research Areas
Authored Publications
Sort By
Automatic Structured Variational Inference
Luca Ambrogioni
Max Hinne
Dave Moore
Marcel van Gerven
AISTATS (2021)
Preview abstract
Probabilistic programming is concerned with the symbolic specification of probabilistic models for which inference can be performed automatically. Gradient-based automatic differentiation stochastic variational inference offers an attractive option as the default method for (differentiable) probabilistic programming. However, the performance of any (parametric) variational approach depends on the choice of an appropriate variational family. Here, we introduce automated structured variational inference (ASVI), a fully automated method for constructing structured variational families, inspired by the closed-form update in conjugate Bayesian models. These pseudo-conjugate families incorporate the forward pass of the input probabilistic program and can therefore capture complex statistical dependencies. Pseudo-conjugate families have the same space and time complexity of the input probabilistic program and are therefore tractable for a very large family of models including both continuous and discrete variables. We provide a fully automatic implementation in TensorFlow Probability. We validate our automatic variational method on a wide range of both low- and high-dimensional inference problems including deep learning components.
View details
Likelihood Ratios for Out-of-Distribution Detection
Peter J. Liu
Mark DePristo
Josh Dillon
arXiv preprint arXiv:1906.02845 (2019)
Preview abstract
Discriminative neural networks offer little or no performance guarantees when deployed on data not generated by the same process as the training distribution. On such out-of-distribution (OOD) inputs, the prediction may not only be erroneous, but confidently so, limiting the safe deployment of classifiers in real-world applications. One such challenging application is bacteria identification based on genomic sequences, which holds the promise of early detection of diseases, but requires a model that can output low confidence predictions on OOD genomic sequences from new bacteria that were not present in the training data. We introduce a genomics dataset for OOD detection that allows other researchers to benchmark progress on this important problem. We investigate deep generative model based approaches for OOD detection and observe that the likelihood score is heavily affected by population level background statistics. We propose a likelihood ratio method for deep generative models which effectively corrects for these confounding background statistics. We benchmark the OOD detection performance of the proposed method against existing approaches on the genomics dataset and show that our method achieves state-of-the-art performance. We demonstrate the generality of the proposed method by showing that it significantly improves OOD detection when applied to deep generative models of images.
View details
Can You Trust Your Model’s Uncertainty? Evaluating Predictive Uncertainty Under Dataset Shift
Yaniv Ovadia
D. Sculley
Sebastian Nowozin
Josh Dillon
Advances in Neural Information Processing Systems (2019)
Preview abstract
Modern machine learning methods including deep learning have achieved great success in predictive accuracy for supervised learning tasks, but may still fall short in giving useful estimates of their predictive {\em uncertainty}. Quantifying uncertainty is especially critical in real-world settings, which often involve distributions that are skewed from the training distribution due to a variety of factors including sample bias and non-stationarity. In such settings, well calibrated uncertainty estimates convey information about when a model's output should (or should not) be trusted. Many probabilistic deep learning methods, including Bayesian-and non-Bayesian methods, have been proposed in the literature for quantifying predictive uncertainty, but to our knowledge there has not previously been a rigorous large-scale empirical comparison of these methods under conditions of distributional skew. We present a large-scale benchmark of existing state-of-the-art methods on classification problems and investigate the effect of distributional skew on accuracy and calibration. We find that traditional post-hoc calibration falls short and some Bayesian methods are intractable for very large data. However, methods that marginalize over models give surprisingly strong results across a broad spectrum.
View details
Preview abstract
In this paper, we investigate the degree to which the encoding of a β-VAE captures label information across multiple architectures on Binary Static MNIST and Omniglot. Even though they are trained in a completely unsupervised manner, we demonstrate that a β-VAE can retain a large amount of label information, even when asked to learn a highly compressed representation.
View details