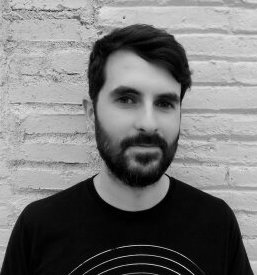
Eduardo Fonseca
I am currently a Research Scientist at Google Research, working in the Sound Understanding Group on machine learning for audio processing. Before joining Google, I received my PhD at the Music Technology Group of Universitat Pompeu Fabra in Barcelona. My PhD thesis focused on sound event classification using different types of supervision. Some of my thesis’ highlights include the Best Audio Representation Learning Paper Award at WASPAA21, and the FSD50K paper and dataset. My research explores learning algorithms for audio processing with different types of supervision, including self-supervised learning, learning with noisy labels, and multimodal learning. I have also been involved in DCASE as Challenge Task Organizer and Technical Program Co-Chair. See my personal website or my Google Scholar profile for a full list of publications.
Research Areas
Authored Publications
Sort By
Google
Self-Supervised Learning from Automatically Separated Sound Scenes
Marco Tagliasacchi
Xavier Serra
WASPAA 2021 (2021)
The Benefit of Temporally-Strong Labels in Audio Event Classification
Caroline Liu
Proceedings of ICASSP 2021 (2021)
Learning Sound Event Classifiers From Web Audio With Noisy Labels
Frederic Font
Xavier Favory
Xavier Serra
Proceedings of ICASSP 2019 (to appear)
Audio Tagging with Noisy Labels and Minimal Supervision
Frederic Font
Xavier Serra
Proceedings of the Detection and Classification of Acoustic Scenes and Events 2019 Workshop (DCASE2019) (to appear)