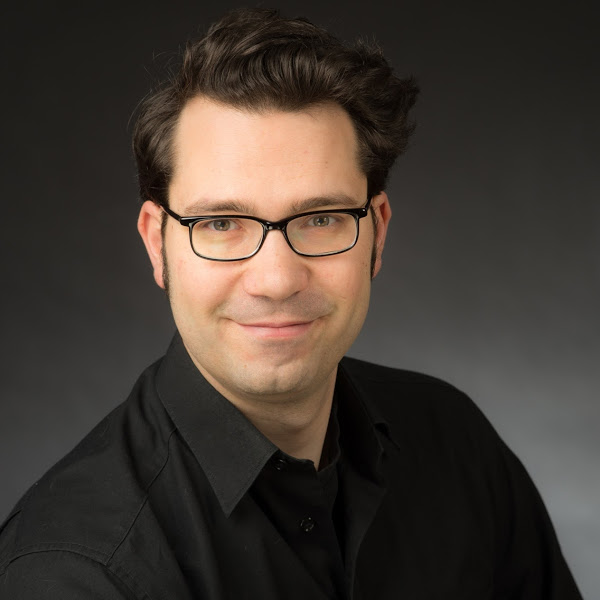
Dirk Holz
Research Areas
Authored Publications
Sort By
Preview abstract
This book includes the post-conference proceedings of the 22nd RoboCup International Symposium, held in Montreal, QC, Canada, in June 2018.
The 32 full revised papers and 11 papers from the winning teams presented were carefully reviewed and selected from 51 submissions.
This book highlights the approaches of champion teams from the competitions and documents the proceedings of the 22nd annual RoboCup International Symposium. Due to the complex research challenges set by the RoboCup initiative, the RoboCup International Symposium offers a unique perspective for exploring scientific and engineering principles underlying advanced robotic and AI systems.
View details
Preview abstract
Example implementations may relate methods and systems for detecting, recognizing, and localizing pallets. For instance, a computing system may receive sensor data representing aspects of an environment, and identify a set of edge points in the sensor data. The computing system may further determine a set of line segments from the set of edge points where each line segment may fit to a subset of the set of edge points. Additionally, the computing system may also filter the set of line segments to exclude line segments that have a length outside a height range and a width range associated with dimensions of a pallet template, and identify, from the filtered set of line segments, a subset of line segments that align with the pallet template. Based on the identified subset of line segments, the computing system may determine a pose of a pallet in the environment.
View details
Preview abstract
Example implementations relate to map generation and alignment. For instance, a computing system may receive and use sensor data indicative of positions of multiple markers positioned relative to a sensor within an environment to determine a pose of the sensor and also create a map that indicates the markers positions. The computing system may also receive and use subsequent sensor data indicative of distances from the sensor to surfaces in the environment and the determined pose of the sensor to determine an occupancy grid map that represents the surfaces within the environment. The computing system may then determine a transformation between the map of the markers and a design model of the environment that relates occupied cells in the occupancy grid map to sampled points from the design model, and provide the transformation between the map of the plurality of markers and the design model.
View details
A Standard for Map Data Representation: IEEE 1873-2015 Facilitates Interoperability Between Robots
Francesco Amigoni
Wonpil Yu
Torsten Andre
Martin Magnusson
Matteo Matteucci
Hyungpil Moon
Masashi Yokotsuka
Geoffrey Biggs
Raj Madhavan
IEEE Robotics & Automation Magazine, 25(2018), pp. 65-76
Preview abstract
The availability of environment maps for autonomous robots enables them to complete several tasks. A new IEEE standard, IEEE 1873-2015, Robot Map Data Representation for Navigation (MDR) [15], sponsored by the IEEE Robotics and Automation Society (RAS) and approved by the IEEE Standards Association Standards Board in September 2015, defines a common representation for two-dimensional (2-D) robot maps and is intended to facilitate interoperability among navigating robots. The standard defines an extensible markup language (XML) data format for exchanging maps between different systems. This article illustrates how metric maps, topological maps, and their combinations can be represented according to the standard.
View details
Localization of Robotic Vehicles
Patent(2018)
Preview abstract
An example method includes determining locations of a plurality of candidate landmarks in relation to a robot based on sensor data from at least one sensor on the robot. The method further includes determining a plurality of sample sets, wherein each sample set comprises a subset of the plurality of candidate landmarks and a plurality of corresponding mapped landmarks. The method also includes determining a transformation for each sample set that relates the candidate landmarks from the subset to the corresponding mapped landmarks. The method additionally includes applying the determined transformation for each sample set to the plurality of candidate landmarks to determine a number of inliers associated with each sample set based on distances between the transformed plurality of candidate landmarks and a plurality of neighbouring mapped landmarks. The method further includes selecting a sample set from the plurality based on the number of inliers associated with each sample set. The method still further includes estimating a pose of the robot based on the selected sample set.
View details
Preview abstract
Examples relate to simultaneous localization and calibration. An example implementation may involve receiving sensor data indicative of markers detected by a sensor on a vehicle located at vehicle poses within an environment, and determining a pose graph representing the vehicle poses and the markers. For instance, the pose graph may include edges associated with a cost function representing a distance measurement between matching marker detections at different vehicle poses. The distance measurement may incorporate the different vehicle poses and a sensor pose on the vehicle. The implementation may further involve determining a sensor pose transform representing the sensor pose on the vehicle that optimizes the cost function associated with the edges in the pose graph, and providing the sensor pose transform. In further examples, motion model parameters of the vehicle may be optimized as part of a graph-based system as well or instead of sensor calibration.
View details
Localization with Negative Mapping
Patent(2018)
Preview abstract
Example embodiments include determining a map of an environment of a robotic vehicle. The map includes locations of a plurality of mapped landmarks within the environment and a false detection source region within the environment. The embodiments further include detecting a plurality of candidate landmarks, and determining which of the detected candidate landmarks correspond to one of the plurality of mapped landmarks and which correspond to false detections. The embodiments additionally include estimating a pose of the robotic vehicle within the environment. The embodiments further include determining which of the detected candidate landmarks determined to correspond to false detections fall within the false detection source region. The embodiments still further include determining a confidence level of the pose estimate based on which of the detected candidate landmarks determined to correspond to false detections fall within the false detection source region.
View details
Landmark Placement for Localization
Patent(2018)
Preview abstract
Embodiments are provided that include receiving sensor data from a sensor positioned at a plurality of positions in an environment. The environment includes a plurality of landmarks. The embodiments also include determining, based on the sensor data, a subset of the plurality of landmarks detected at each of the plurality of positions. The embodiments further include determining, based on the subset of the plurality of landmarks detected at each of the plurality of positions, a detection frequency of each landmark. The embodiments additionally include determining, based on the determined detection frequency of each landmark, a localization viability metric associated with each landmark. The embodiments still further include providing for display, via a user interface, a map of the environment. The map includes an indication of the localization viability metric associated with each landmark.
View details
Preview abstract
Methods and systems for detecting sensor orientation characteristics using marker-based localization are disclosed herein. In one aspect, a robotic device can: receive a map of a horizontal marker plane that includes mapped positions of a first marker and a second marker arranged in the horizontal marker plane; receive, from a sensor configured to scan a two-dimensional sensor plane, sensor data indicative of positions of the first and second markers relative to the sensor; determine measured positions of the first and second markers based on the sensor data and a current position of the sensor; determine a difference vector between a first vector that connects the mapped positions of the first and second markers and a second vector that connects the measured positions of the first and second markers; and determine, based on the difference vector, an orientation of the two-dimensional sensor plane relative to the horizontal marker plane.
View details