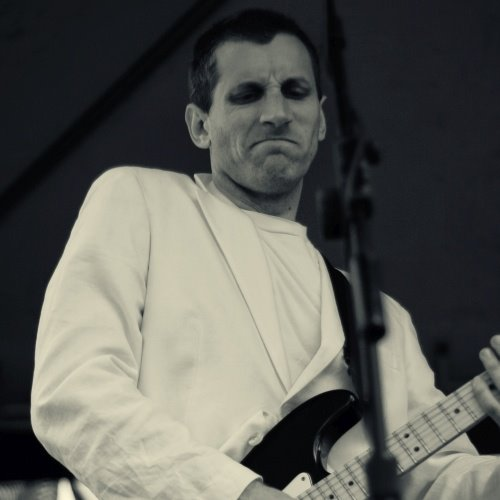
Dan Morris
I use AI to help conservation practitioners spend less time doing boring things. Also see my Google Scholar profile and my personal site.
Research Areas
Authored Publications
Sort By
Google
A versatile, semi-automated image analysis workflow for time-lapse camera trap image classification
Hanna Böhner
Olga Pokrovskaya
Desheng Liu
Natalia Sokolova
Olivier Gilg
Wenbo Zhou
Ivan Fufachev
Peter Ungar
Rolf Anker Ims
Alexsandr Sokolov
Dorothee Ehrich
Gerardo Celis
Ecological Informatics (2024)
To crop or not to crop: comparing whole-image and cropped classification on a large dataset of camera trap images
Jorge Ahumada
Sara Beery
Stefan Istrate
Clint Kim
Tanya Birch
Tomer Gadot
IET Computer Vision (2024)