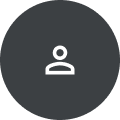
Congcong Li
http://chenlab.ece.cornell.edu/people/congcong/
Research Areas
Authored Publications
Sort By
Google
Multi-modal 3D Human Pose Estimation with 2D Weak Supervision in Autonomous Driving
Jingxiao Zheng
Xinwei Shi
Alexander Gorban
Junhua Mao
Charles Qi
Visesh Chari
Andre Cornman
Yin Zhou
Dragomir Anguelov
CVPR'2022, Workshop on Autonomous Driving, IEEE
Improving 3D Object Detection through Progressive Population Based Augmentation
Shuyang Cheng
Zhaoqi Leng
Barret Richard Zoph
Chunyan Bai
Jiquan Ngiam
Vijay Vasudevan
Jon Shlens
Drago Anguelov
ECCV'2020
Learning semantic relationships for better action retrieval in images
Vignesh Ramanathan
Jia Deng
Wei Han
Zhen Li
Kunlong Gu
Samy Bengio
Chuck Rosenberg
Li Fei-Fei
CVPR (2015)