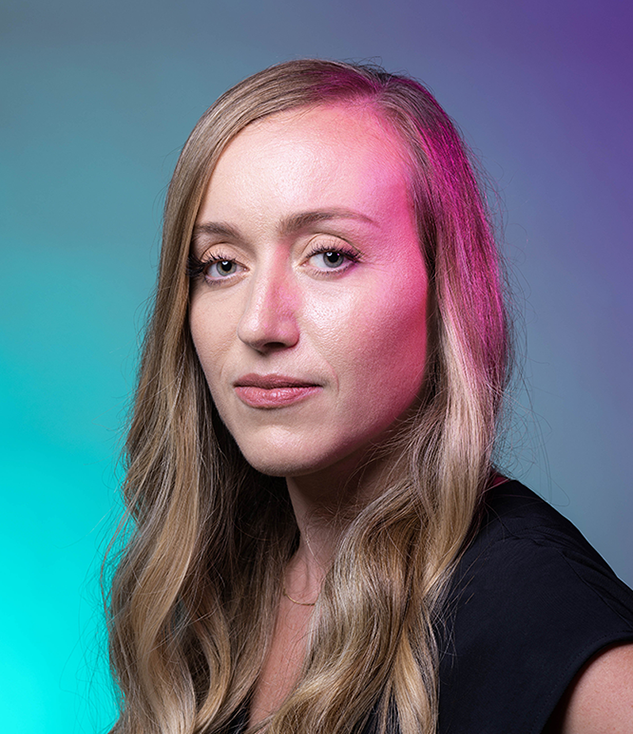
Chloe LeGendre
Chloe LeGendre is a senior software engineer in Google Research, currently working on computational photography, with interests in machine learning applied to problems in computer graphics, photography, imaging, and computer vision. Her research interests include scene lighting measurement and estimation, color science, and portrait photography manipulation. She graduated with a PhD in Computer Science in 2019 from the University of Southern California as a member of USC's Institute for Creative Technologies in the Vision and Graphics Lab.
Research Areas
Authored Publications
Sort By
Google
Total Relighting: Learning to Relight Portraits for Background Replacement
Christian Haene
Sofien Bouaziz
Christoph Rhemann
Paul Debevec
SIGGRAPH and TOG (2021)
Deep Relightable Textures: Volumetric Performance Capture with Neural Rendering
Abhi Meka
Christian Haene
Peter Barnum
Philip Davidson
Daniel Erickson
Jonathan Taylor
Sofien Bouaziz
Wan-Chun Alex Ma
Ryan Overbeck
Thabo Beeler
Paul Debevec
Shahram Izadi
Christian Theobalt
Christoph Rhemann
SIGGRAPH Asia and TOG (2020)
Learning Illumination from Diverse Portraits
Wan-Chun Alex Ma
Christoph Rhemann
Jason Dourgarian
Paul Debevec
SIGGRAPH Asia 2020 Technical Communications (2020)
DeepLight: Learning Illumination for Unconstrained Mobile Mixed Reality
Graham Fyffe
John Flynn
Laurent Charbonnel
Paul Debevec
Wan-Chun Alex Ma
Proceedings of the IEEE/CVF Conference on Computer Vision and Pattern Recognition (2019), pp. 5918-5928