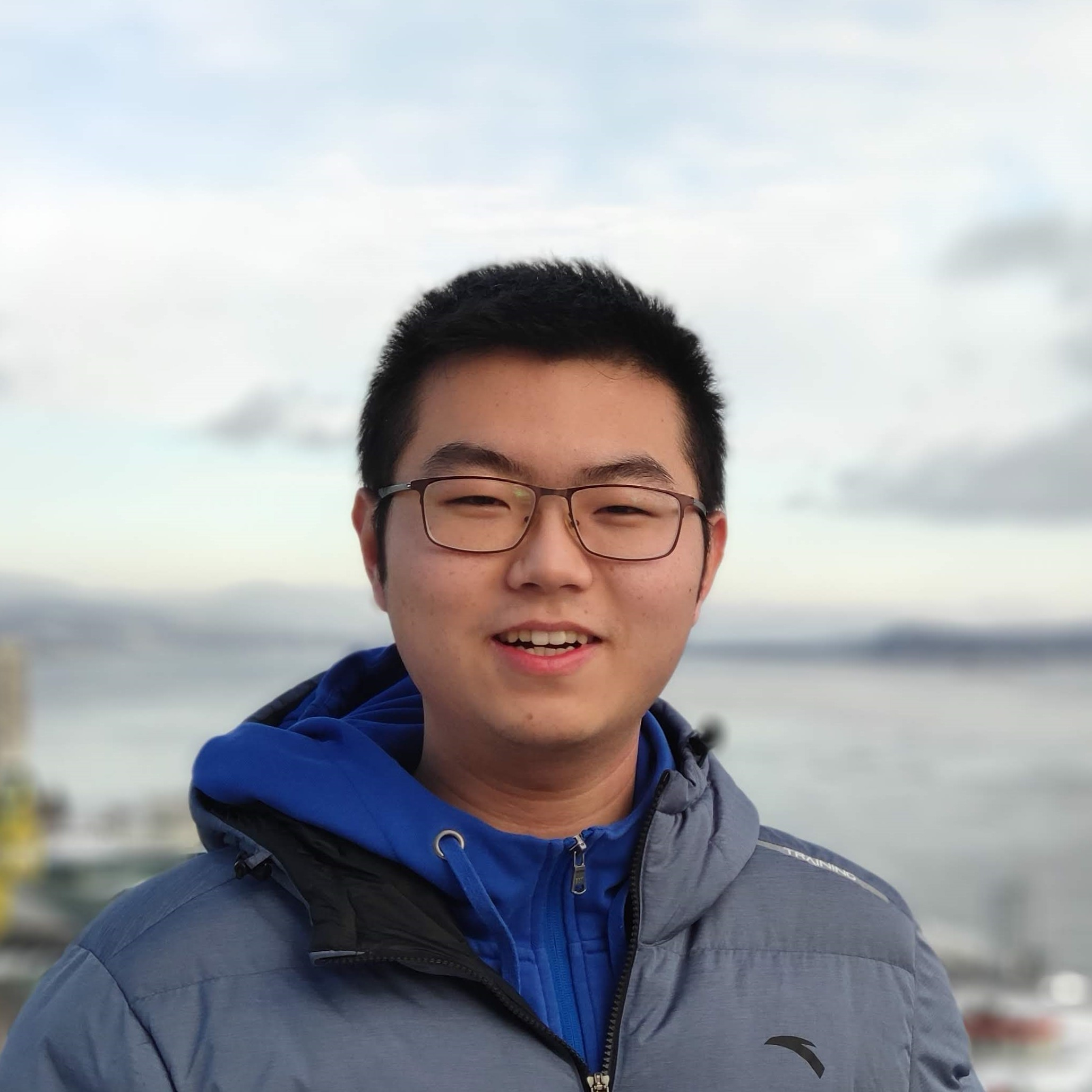
Chen Qu
I am Software Engineer at Google. I have broad research interests in information retrieval, natural language processing, machine learning, and deep learning. Before joining Google, I received my PhD in Computer Science from the Center for Intelligent Information Retrieval (CIIR), College of Information and Computer Sciences, University of Massachusetts Amherst. For a full list of my publications see my personal page.
Authored Publications
Sort By
Large Dual Encoders Are Generalizable Retrievers
Jianmo Ni
Zhuyun Dai
Vincent Zhao
Yi Luan
Keith B. Hall
Ming-Wei Chang
Yinfei Yang
(2022)
Preview abstract
It has been shown that dual encoders trained on one domain often fail to generalize to other domains for retrieval tasks. One widespread belief is that the bottleneck layer of a dual encoder, where the final score is simply a dot-product between a query vector and a passage vector, is too limited to make dual encoders an effective retrieval model for out-ofdomain generalization. In this paper, we challenge this belief by scaling up the size of the dual encoder model while keeping the bottleneck embedding size fixed. With multi-stage training, surprisingly, scaling up the model size brings significant improvement on a variety of retrieval tasks, especially for out-of-domain generalization. Experimental results show that our dual encoders, Generalizable T5-based dense Retrievers (GTR), outperform existing sparse and dense retrievers on the BEIR dataset (Thakur et al., 2021) significantly. Most surprisingly, our ablation study finds that GTR is very data efficient, as it only needs 10% of MS Marco supervised data to
achieve the best out-of-domain performance.
View details
Natural Language Understanding with Privacy-Preserving BERT
Proceedings of the 30th ACM International Conference on Information and Knowledge Management, ACM (2021)
Preview abstract
Privacy preservation remains a key challenge in data mining and Natural Language Understanding (NLU). Previous research shows that the input text or even text embeddings can leak private information. This concern motivates our research on effective privacy preservation approaches for pretrained Language Models (LMs). We investigate the privacy and utility implications of applying dχ-privacy, a variant of Local Differential Privacy, to BERT fine-tuning in NLU applications. More importantly, we further propose privacy-adaptive LM pretraining methods and show that our approach can boost the utility of BERT dramatically while retaining the same level of privacy protection. We also quantify the level of privacy preservation and provide guidance on privacy configuration. Our experiments and findings lay the groundwork for future explorations of privacy-preserving NLU with pretrained LMs.
View details