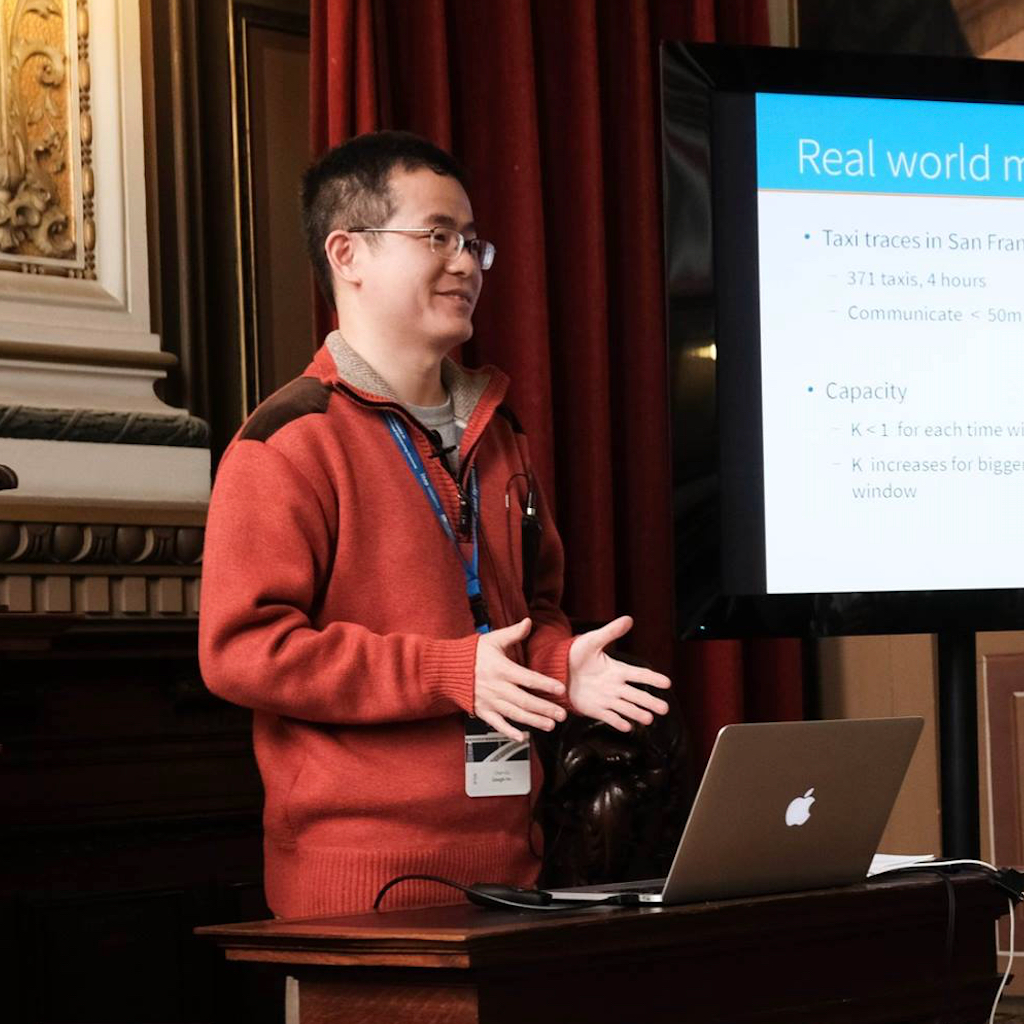
Chen Gu
顾晨
Google Research Scientist
Education
- Stanford University, Ph.D. in Computational and Mathematical Engineering, 2013.
- Stanford University, M.S. in Electrical Engineering, 2008.
- Tsinghua University, B.Eng. in Computer Science and Technology, 2005.
Olympics
- 32nd ACM International Collegiate Programming Contest (ICPC) World Finals, Silver Medal & 7th Place, Representing Stanford University. Banff, Canada, 2008.
Awards
- Google Android Quality Awards, Hall of Fame. More indoor accuracy for less compute resources, 2022.
Interests
- I am interested in anything related to mathematics.
- I am not interested in anything not related to mathematics.
Research Areas
Authored Publications
Sort By
Ubiquitous and Low-Cost Generation of Elevation Pseudo Ground Control Points
Etienne Le Grand
Moustafa Youssef
14th International Conference on Indoor Positioning and Indoor Navigation (IPIN). Hong Kong, China, 2024.
Preview abstract
In this paper, we design a system to generate Pseudo Ground Control Points (PGCPs) using standard low-cost widely available GNSS receivers in a crowd-sourcing manner. We propose a number of GNSS points filters that removes different causes of errors and biases, and design a linear regression height estimator leading to high-accuracy PGCP elevations. Evaluation of our system shows that the PGCPs can achieve a median accuracy of 22.5 cm in 25 metropolitan areas in the USA.
View details
The Effect of Ground Truth Accuracy on the Evaluation of Localization Systems
Ahmed Shokry
Moustafa Youssef
40th IEEE International Conference on Computer Communications (INFOCOM). Virtual Conference, 2021.
Preview abstract
The ability to accurately evaluate the performance of location determination systems is crucial for many applications. Typically, the performance of such systems is obtained by comparing ground truth locations with estimated locations. However, these ground truth locations are usually obtained by clicking on a map or using other worldwide available technologies like GPS. This introduces ground truth errors that are due to the marking process, map distortions, or inherent GPS inaccuracy.
In this paper, we present a theoretical framework for analyzing the effect of ground truth errors on the evaluation of localization systems. Based on that, we design two algorithms for computing the real algorithmic error from the validation error and marking/map ground truth errors, respectively. We further establish bounds on different performance metrics.
Validation of our theoretical assumptions and analysis using real data collected in a typical environment shows the ability of our theoretical framework to correct the estimated error of a localization algorithm in the presence of ground truth errors. Specifically, our marking error algorithm matches the real error CDF within 4%, and our map error algorithm provides a more accurate estimate of the median/tail error by 150%/72% when the map is shifted by 6m.
View details
On the Ability of Mobile Sensor Networks to Diffuse Information
Ian Downes
Omprakash Gnawali
Leonidas Guibas
17th ACM/IEEE International Conference on Information Processing in Sensor Networks (CPS Week - IPSN). Porto, Portugal, 2018.
Preview abstract
We examine the ability of networks formed by mobile sensor nodes to diffuse information in the case when communication is only possible during opportunistic encounters. Our setting assumes that mobile nodes are continuously sensing the world and acquiring new information. We form an abstract model of this situation and show by theoretical analysis, simulation, and real mobility data that the diffusion of information in this setting cannot be as efficient as when we allow arbitrary contact patterns between the nodes with the same overall contact statistics. This establishes a fundamental asymptotic limitation on the information diffusion capacity of such opportunistic mobile sensor networks --- the encounter patterns arising out of physical motions in a geometric space are not ideal for information diffusion.
View details
Topology-Driven Trajectory Synthesis with an Example on Retinal Cell Motions
Leonidas Guibas
Michael Kerber
14th International Workshop on Algorithms in Bioinformatics (ALGO - WABI). Wroclaw, Poland, 2014.
Preview abstract
We design a probabilistic trajectory synthesis algorithm for generating time-varying sequences of geometric configuration data. The algorithm takes a set of observed samples (each may come from a different trajectory) and simulates the dynamic evolution of the patterns in O(n^2 log n) time. To synthesize geometric configurations with indistinct identities, we use the pair correlation function to summarize point distribution, and alpha-shapes to maintain topological shape features based on a fast persistence matching approach. We apply our method to build a computational model for the geometric transformation of the cone mosaic in retinitis pigmentosa --- an inherited and currently untreatable retinal degeneration.
View details
Building Markov State Models with Solvent Dynamics
Huang-Wei Chang
Lutz Maibaum
Vijay Pande
Gunnar Carlsson
Leonidas Guibas
11th Asia Pacific Bioinformatics Conference (APBC) & BMC Bioinformatics Journal. Vancouver, Canada, 2013.
Preview abstract
Background: Markov state models have been widely used to study conformational changes of biological macromolecules. These models are built from short timescale simulations and then propagated to extract long timescale dynamics. However, the solvent information in molecular simulations are often ignored in current methods, because of the large number of solvent molecules in a system and the indistinguishability of solvent molecules upon their exchange.
Methods: We present a solvent signature that compactly summarizes the solvent distribution in the high-dimensional data, and then define a distance metric between different configurations using this signature. We next incorporate the solvent information into the construction of Markov state models and present a fast geometric clustering algorithm which combines both the solute-based and solvent-based distances.
Results: We have tested our method on several different molecular dynamical systems, including alanine dipeptide, carbon nanotube, and benzene rings. With the new solvent-based signatures, we are able to identify different solvent distributions near the solute. Furthermore, when the solute has a concave shape, we can also capture the water number inside the solute structure. Finally we have compared the performances of different Markov state models. The experiment results show that our approach improves the existing methods both in the computational running time and the metastability.
Conclusions: In this paper we have initiated an study to build Markov state models for molecular dynamical systems with solvent degrees of freedom. The methods we described should also be broadly applicable to a wide range of biomolecular simulation analyses.
View details
Kinetically-aware Conformational Distances in Molecular Dynamics
Xiaoye Jiang
Leonidas Guibas
23rd Canadian Conference on Computational Geometry (CCCG). Toronto, Canada, 2011.
Preview abstract
In this paper, we present a novel approach for discovering kinetically metastable states of biomolecular conformations. Several kinetically-aware metrics which encode both geometric and kinetic information about biomolecules are proposed. We embed the new metrics into k-center clustering and r-cover clustering algorithms to estimate the metastable states. Those clustering algorithms using kinetically-aware metrics are tested on a large scale biomolecule conformation dataset. It turns out that our algorithms are able to identify the kinetic meaningful clusters.
View details
Distance between Folded Objects
Leonidas Guibas
27th European Workshop on Computational Geometry (EuroCG). Morschach, Switzerland, 2011.
Preview abstract
Geometric folding problems have recently attracted much attention in both mathematics and theoretical computer science. In this paper, we study the following basic problem: given a set of folded conformations of a unit-length rope in 1D, how do we decide which are more similar or less similar to each other? We first define a distance function between flat folded states that incorporates both the geometry and the overlap order. Then we do some computational experiments clustering random folded ropes with this distance metric. Finally, we generalize our results for 1D folded ropes to flat folded papers (origami) in 2D.
View details