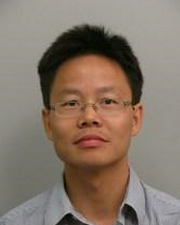
Aiyou Chen
Aiyou Chen received a B.Sc degree in mathematics from Wuhan University in 1997, M.Sc degree from Peking University in 2000, and a Ph.D. degree in statistics from UC Berkeley in 2004. His Ph.D. dissertation was on semiparametric inference for independent component analysis. He was a Member of Technical Staff at Bell Labs, Murray Hill, NJ, from Aug 2004 to Aug 2010 with research in statistical methodologies and applications mostly with network data. Since then, he works as a Statistician at Google.
Authored Publications
Sort By
Preview abstract
Evaluating the incremental return on ad spend (iROAS) of a prospective online marketing strategy (i.e., the ratio of the strategy’s causal effect on some response metric of interest relative to its causal effect on the ad spend) has become increasingly more important. Although randomized “geo experiments” are frequently employed for this evaluation, obtaining reliable estimates of iROAS can be challenging, as oftentimes only a small number of highly heterogeneous units are used. Moreover, advertisers frequently impose budget constraints on their ad spends which further complicates causal
inference by introducing interference between the experimental units. In this paper we formulate a novel statistical framework for inferring the iROAS of online advertising from randomized paired geo experiment, which further motivates and provides new insights into Rosenbaum’s arguments on instrumental variables, and we propose and develop a robust, distribution-free and interpretable estimator “Trimmed Match” as well as a data-driven choice of the tuning parameter which may be of independent interest. We investigate the sensitivity of Trimmed Match to some violations of its assumptions and show that it can be more efficient than some alternative estimators based on
simulated data. We then demonstrate its practical utility with real case studies.
View details
Preview abstract
We develop new semiparametric methods for estimating treatment effects. We
focus on a setting where the outcome distributions may be thick tailed, where treatment
effects are small, where sample sizes are large and where assignment is completely random. This setting is of particular interest in recent experimentation in tech companies.
We propose using parametric models for the treatment effects, as opposed to parametric models for the full outcome distributions. This leads to semiparametric models for
the outcome distributions. We derive the semiparametric efficiency bound for this setting,
and propose efficient estimators. In the case with a constant treatment effect one of the
proposed estimators has an interesting interpretation as a weighted average of quantile
treatment effects, with the weights proportional to (minus) the second derivative of the log
of the density of the potential outcomes. Our analysis also results in an extension of Huber’s model and trimmed mean to include asymmetry and a simplified condition on linear
combinations of order statistics, which may be of independent interest.
View details
Preview abstract
How to measure the incremental return on Ad spend (iROAS) is a fundamental problem for the online advertising industry. A standard modern tool is to run randomized geo experiments, where experimental units are non-overlapping ad-targetable geographical areas (Vaver & Koehler 2011). However, how to design a reliable and cost-effective geo experiment can be complicated, for example: 1) the number of geos is often small, 2) the response metric (e.g. revenue) across geos can be very heavy-tailed due to geo heterogeneity, and furthermore 3) the response metric can vary dramatically over time. To address these issues, we propose a robust nonparametric method for the design, called Trimmed Match Design
(TMD), which extends the idea of Trimmed Match (Chen & Au 2019) and furthermore integrates the techniques of optimal subset pairing and sample splitting in a novel and systematic manner. Some simulation and real case studies are presented. We also point out a few open problems for future research.
View details
Bias Correction For Paid Search In Media Mix Modeling
Jim Koehler
Mike Perry
research.google.com, Google Inc. (2018)
Preview abstract
Evaluating the return on ad spend (ROAS), the causal effect of advertising
on sales, is critical to advertisers for understanding the performance of their
existing marketing strategy as well as how to improve and optimize it. Media
Mix Modeling (MMM) has been used as a convenient analytical tool to address
the problem using observational data. However it is well recognized that MMM
suffers from various fundamental challenges: data collection, model specification
and selection bias due to ad targeting, among others (Chan & Perry 2017; Wolfe
2016).
In this paper, we study the challenge associated with measuring the impact
of search ads in MMM, namely the selection bias due to ad targeting. Using
causal diagrams of the search ad environment, we derive a statistically principled
method for bias correction based on the back-door criterion (Pearl 2013).
We use case studies to show that the method provides promising results by
comparison with results from randomized experiments. We also report a more
complex case study where the advertiser had spent on more than a dozen media
channels but results from a randomized experiment are not available. Both our
theory and empirical studies suggest that in some common, practical scenarios,
one may be able to obtain an approximately unbiased estimate of search ad
ROAS.
View details
Data Enriched Linear Regression
Art Owen
Electronic Journal of Statistics, 9 (2015), pp. 1078-1112 (to appear)
Preview abstract
We present a linear regression method for predictions on a small data set making use of a second possibly biased data set that may be much larger. Our method fits linear regressions to the two data sets while penalizing the difference between predictions made by those two models. The resulting algorithm is a shrinkage method similar to those used in small area estimation. We find a Stein-type result for Gaussian responses: when the model has 5 or more coefficients and 10 or more error degrees of freedom, it becomes inadmissible to use only the small data set, no matter how large the bias is. We also present both plug-in and AICc-based methods to tune our penalty parameter. Most of our results use an L2 penalty, but we obtain formulas for L1 penalized estimates when the model is specialized to the location setting. Ordinary Stein shrinkage provides an inadmissibility result for only 3 or more coefficients, but we find that our shrinkage method typically produces much lower squared errors in as few as 5 or 10 dimensions when the bias is small and essentially equivalent squared errors when the bias is large.
View details
Preview abstract
There is increasing interest in measuring the overlap and/or incremental reach of cross-media campaigns. The direct method is to use a cross-media panel but these are expensive to scale across all media. Typically, the cross-media panel is too small to produce reliable estimates when the interest comes down to subsets of the population. An alternative is to combine information from a small cross-media panel with a larger, cheaper but potentially biased single media panel. In this article, we develop a data enrichment approach specifically for incremental reach estimation. The approach not only integrates information from both panels that takes into account potential panel bias, but borrows strength from modeling conditional dependence of cross-media reaches. We demonstrate the approach with data from six campaigns for estimating YouTube video ad incremental reach over TV. In a simulation directly modeled on the actual data, we find that
data enrichment yields much greater accuracy than one would get by either ignoring the larger panel, or by using it in a data fusion.
View details
Cluster forest
Donghui Yan
Michael I Jordan
Computational Statistics and Data Analysis, 66 (2013), pp. 178-192
Preview abstract
With inspiration from Random Forests (RF) in the context of classification, a new clustering ensemble method---Cluster Forests (CF) is proposed. Geometrically, CF randomly probes a high-dimensional data cloud to obtain "good local clusterings" and then aggregates via spectral clustering to obtain cluster assignments for the whole dataset. The search for good local clusterings is guided by a cluster quality measure kappa. CF progressively improves each local clustering in a fashion that resembles the tree growth in RF. Empirical studies on several real-world datasets under two different performance metrics show that CF compares favorably to its competitors. Theoretical analysis reveals that the kappa measure makes it possible to grow the local clustering in a desirable way---it is "noise-resistant". A closed-form expression is obtained for the mis-clustering rate of spectral clustering under a perturbation model, which yields new insights into some aspects of spectral clustering.
View details
Preview abstract
Many algorithms have been proposed for fitting network models with communities but most of them do not scale well to large networks, and often fail on sparse networks. Here we propose a new fast pseudo-likelihood method for fitting the stochastic block model for networks, as well as a variant that allows for an arbitrary degree distribution by conditioning on degrees. We show that the algorithms perform well under a range of settings, including on very sparse networks, and illustrate on the example of a network of political blogs. We also propose spectral clustering with perturbations, a method of independent interest, which works well on sparse networks where regular spectral clustering fails, and use it to provide an initial value for pseudo-likelihood.
View details
Efficient Spectral Neighborhood Blocking for Entity Resolution
Liangcai Shu
Ming Xiong
Weiyi Meng
International Conference on Data Engineering 2011 (ICDE), IEEE, pp. 1-12
Preview abstract
In many telecom and web applications, there is a
need to identify whether data objects in the same source or
different sources represent the same entity in the real world. This problem arises for subscribers in multiple services, customers in supply chain management, and users in social networks when there lacks a unique identifier across multiple data sources to represent a real-world entity. Entity resolution is to identify and discover objects in the data sets that refer to the same entity in the real world.
We investigate the entity resolution problem for large data sets where efficient and scalable solutions are needed. We propose a novel unsupervised blocking algorithm, namely SPectrAl Neighborhood (SPAN), which constructs a fast bipartition tree for the records based on spectral clustering such that real entities can be identified accurately by neighborhood records in the tree. There
are two major novel aspects in our approach: 1) We develop a fast algorithm that performs spectral clustering without computing pairwise similarities explicitly, which dramatically improves the scalability of the standard spectral clustering algorithm; 2) We utilize a stopping criterion specified by Newman-Girvan modularity in the bipartition process. Our experimental results with both synthetic and real-world data demonstrate that SPAN is robust and outperforms other blocking algorithms in terms of
accuracy while it is efficient and scalable to deal with large data sets.
View details
Preview abstract
Probability models on graphs are becoming increasingly
important in many applications, but statistical tools for fitting such models are not yet well developed. Here we propose a general method of moments approach that can be used to fit a large class of probability models through empirical counts of certain patterns in a graph. We
establish some general asymptotic properties of empirical graph moments and prove consistency of the estimates as the graph size grows for all ranges of the average degree including Ω(1). Additional results are obtained for the important special case of degree distributions.
View details