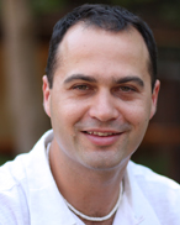
Liviu Panait
Liviu Panait received a Ph.D. degree in Computer Science from George Mason University in 2007, and is currently working on organizing the world's information and making it universally accessible and useful. His research interests include machine learning, multiagent systems, computer games, artificial life, data mining, and information retrieval.
Liviu Panait co-chaired the AAMAS 2007 Workshop on Adaptive and Learning Agents, the AAMAS 2006 Workshop on Adaptation and Learning in Autonomous Agents and Multiagent Systems, co-organized the AAAI 2005 Fall Symposium on Coevolutionary and Coadaptive Systems, served as a program committee member or as an invited reviewer for multiple international conferences and journals, and he is a member of the IEEE Task Force on Coevolution. He is a co-author of the ECJ evolutionary computation library and the MASON multi-agent simulation toolkit. For more information, please visit his home page.
Research Areas
Authored Publications
Sort By
Google
Learning to Generate Image Embeddings with User-level Differential Privacy
Maxwell D. Collins
Yuxiao Wang
Sewoong Oh
IEEE Conference on Computer Vision and Pattern Recognition (CVPR) (2023) (to appear)
Theoretical Convergence Guarantees for Cooperative Coevolutionary Algorithms
Evolutionary Computation Journal (2010)
Theoretical Advantages of Lenient Learners in Multiagent Systems
Karl Tuyls
Proceedings of the Sixth International Conference on Autonomous Agents and Multi-agent Systems (AAMAS-07), ACM (2007)