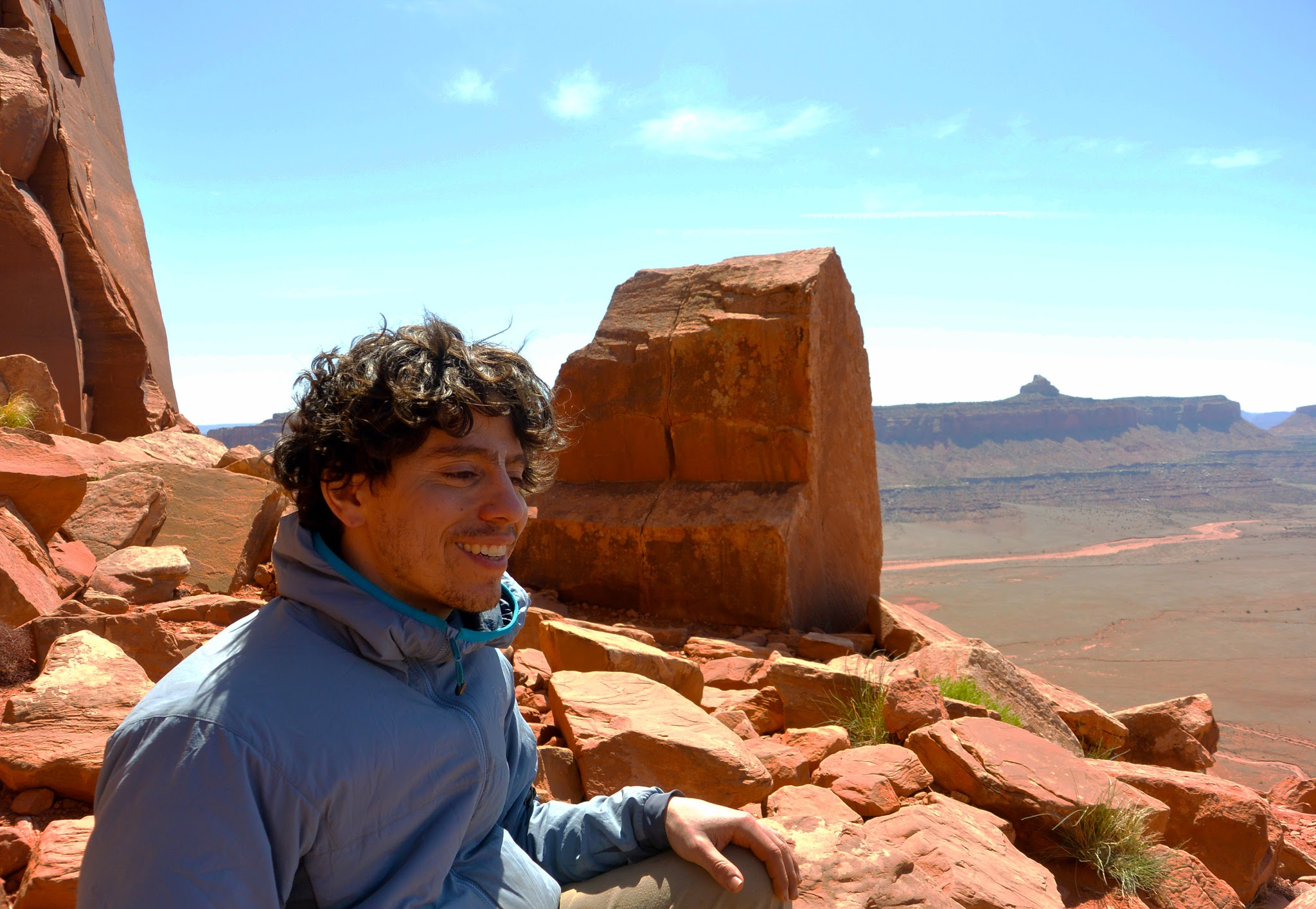
Assaf Hurwitz-Michaely
Research Areas
Authored Publications
Sort By
Preview abstract
We present a novel approach for improving overall quality of
keyword spotting using contextual automatic speech recognition
(ASR) system. On voice-activated devices with limited resources,
it is common that a keyword spotting system is run on
the device in order to detect a trigger phrase (e.g. “ok google”)
and decide which audio should be sent to the server (to be transcribed
by the ASR system and processed to generate a response
to the user). Due to limited resources on a device, the device
keyword spotting system might introduce false accepts (FAs)
and false rejects (FRs) that can cause a negative user experience.
We describe a system that uses server-side contextual ASR and
dynamic classes for improved keyword spotting. We show that
this method can significantly reduce FA rates (by 89%) while
minimally increasing FR rate (0.15%). Furthermore, we show
that this system helps reduce Word Error Rate (WER) (by 10%
to 50% relative, on different test sets) and allows users to speak
seamlessly, without pausing between the trigger phrase and the
command.
View details
Unsupervised Context Learning For Speech Recognition
Justin Scheiner
Spoken Language Technology (SLT) Workshop, IEEE(2016)
Preview abstract
It has been shown in the literature that automatic speech
recognition systems can greatly benefit from contextual in-
formation [ref]. The contextual information can be used to
simplify the search and improve recognition accuracy. The
types of useful contextual information can include the name
of the application the user is in, the contents on the user’s
phone screen, user’s location, a certain dialog state, etc.
Building a separate language model for each of these types
of context is not feasible due to limited resources or limited
amount of training data.
In this paper we describe an approach for unsupervised
learning of contextual information and automatic building of
contextual (biasing) models. Our approach can be used to
build a large number of small contextual models from a lim-
ited amount of available unsupervised training data. We de-
scribe how n-grams relevant for a particular context are au-
tomatically selected as well as how an optimal size of a final
contextual model built is chosen. Our experimental results
show great accuracy improvements for several types of con-
text.
View details
Bringing Contextual Information to Google Speech Recognition
Preview
Keith Hall
David Rybach
Pedro Moreno
Interspeech 2015, International Speech Communications Association