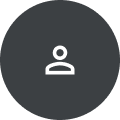
Alexander Mordvintsev
Authored Publications
Sort By
Google
Transformers learn in-context by gradient descent
João Sacramento
International Conference on Machine Learning (2023), pp. 35151-35174
The Building Blocks of Interpretability
Christopher Olah
Arvind Satyanarayan
Ian Johnson
Shan Carter
Ludwig Schubert
Katherine Ye
Distill (2018)
Learning by Association - A versatile semi-supervised training method for neural networks
Philip Haeusser
Daniel Cremers
IEEE Conference on Computer Vision and Pattern Recognition (CVPR) (2017)
Associative Domain Adaptation
Philip Haeusser
Thomas Frerix
Daniel Cremers
International Conference on Computer Vision (ICCV), IEEE (2017) (to appear)