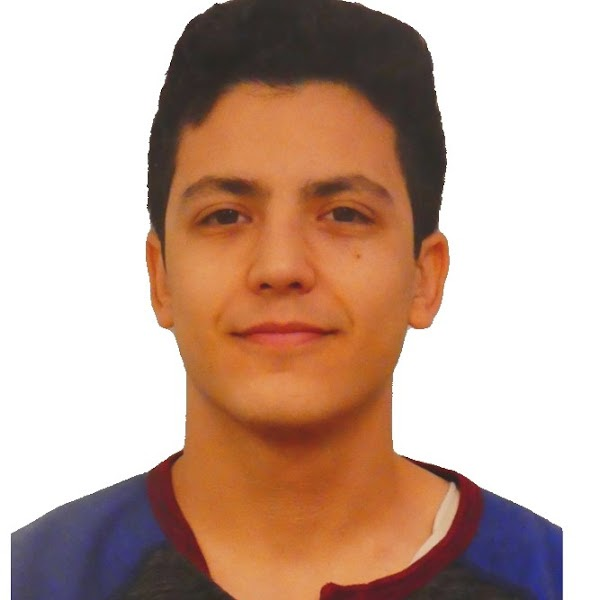
Avishai Zagoury
Software Engineer at Google Research
Authored Publications
Sort By
Preview abstract
Given the ubiquity of negative campaigning in recent political elections, we find it important to study its properties from a computational perspective. To this end, we present a model where elections can be manipulated by convincing voters to demote specific non-favored candidates, and study its properties in the classic setting of scoring rules. When the goal is constructive (making a preferred candidate win), we prove that finding such a demotion strategy is easy for Plurality and Veto, while generally hard for t-approval and Borda. We also provide a t-factor approximation for t-approval for every fixed t, and a 3-factor approximation algorithm for Borda. Interestingly enough - following recent trends in political science that show that the effectiveness of negative campaigning depends on the type of candidate and demographic - when assigning varying prices to different possible demotion operations, we are able to provide inapproximability results. When the goal is destructive (making the leading opponent lose), we show that the problem is easy for a broad class of scoring rules.
View details
Preview abstract
Although large neural language models (LMs) like BERT can be finetuned to yield state-of-the-art results on many NLP tasks, it is often unclear what these models actually learn. Here we study using such LMs to fill in entities in comparative questions, like “Which country is older, India or ___?”—i.e., we study the ability of neural LMs to ask (not answer) reasonable questions. We show that accuracy in this fill-in-the-blank task is well-correlated with human judgements of whether a question is reasonable, and that these models can be trained to achieve nearly human-level performance in completing comparative questions in three different sub-domains. However, analysis shows that what they learn fails to model any sort of broad notion of which entities are semantically comparable or similar—instead the trained models are very domain-specific, and performance is highly correlated with co-occurrences between specific entities observed in the training set. This is true both for models that are pre-trained on general text corpora, as well as models trained on a large corpus of comparison questions. Our study thus reinforces recent results on the difficulty of making claims about a deep model’s world knowledge or linguistic competence based on performance on specific benchmark problems. We make our evaluation datasets publicly available to foster future research.
View details