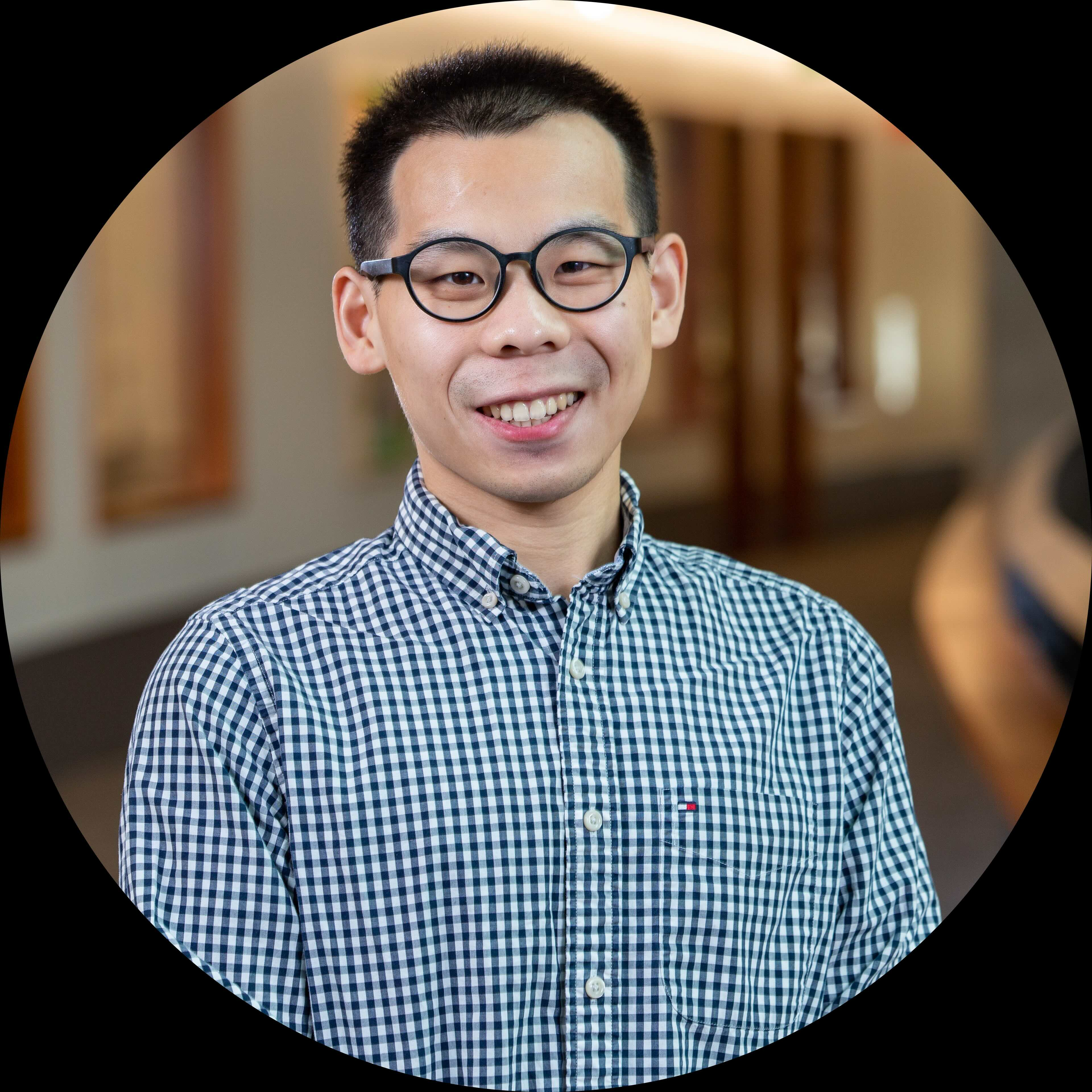
Xin Liu
Xin Liu is a Research Scientist at Google Consumer Health Research. He received his PhD in Computer Science from the University of Washington in 2023. His PhD research was supported by the Google PhD Fellowship .
His work is at the intersection of ubiquitous and mobile computing, machine learning, and health. In Xin's research, he studies how to enable mobile health + AI at scale, with a focus on building foundation models for sensor and consumer health data.
More information: https://xliucs.github.io/
Authored Publications
Sort By
Evidence of Differences in Diurnal Electrodermal Patterns by Mental Health Status in Free-Living Data
Daniel McDuff
Isaac Galatzer-Levy
Seamus Thomson
Andrew Barakat
Conor Heneghan
Samy Abdel-Ghaffar
Jake Sunshine
Ming-Zher Poh
Lindsey Sunden
Allen Jiang
Ari Winbush
Benjamin Nelson
Nicholas Allen
medRxiv (2024)
Preview abstract
Electrodermal activity (EDA) is a standardized measure of sympathetic arousal that has been linked to depression in laboratory experiments. However, the inability to measure EDA passively over time and in the real-world has limited conclusions that can be drawn about EDA as an indicator of mental health status outside of a controlled setting. Recent smartwatches have begun to incorporate wrist-worn continuous EDA sensors that enable longitudinal measurement in every-day life. This work presents the first example of passively collected, diurnal variations in EDA present in people with depression, anxiety and perceived stress. Subjects who were depressed had higher tonic EDA and heart rate, despite not engaging in greater physical activity, compared to those that were not depressed. EDA measurements showed differences between groups that were most prominent during the early morning. We did not observe amplitude or phase differences in the diurnal patterns.
View details
What Are The Odds? Language Models are Capable of Probabilistic Reasoning
Akshay Paruchuri
Shun Liao
Jake Sunshine
Tim Althoff
Daniel McDuff
arXiv (2024)
Preview abstract
Language models (LM) are capable of remarkably complex linguistic tasks; however, numerical reasoning is an area in which they frequently struggle. An important but rarely evaluated form of reasoning is understanding probability distributions. In this paper we focus on evaluating the probabilistic reasoning capabilities of LMs using idealized and real-world statistical distributions. We perform a systematic evaluation of state-of-the-art LMs on three tasks: estimating percentiles, drawing samples, and calculating probabilities. We find that zero-shot performance varies dramatically across different families of distributions and that performance can be improved significantly by using anchoring examples (shots) from within a distribution, or to a lesser extent across distributions within the same family. For real-world distributions, the absence of in-context examples can be substituted with context from which the LM can retrieve some statistics. Finally, we show that simply providing the mean and standard deviation of real-world distributions improves performance. To conduct this work, we developed a comprehensive benchmark distribution dataset with associated question-answer pairs that we release publicly, including questions about population health, climate, and finance.
View details
Towards a Personal Health Large Language Model
Anastasiya Belyaeva
Nick Furlotte
Zhun Yang
Chace Lee
Erik Schenck
Yojan Patel
Jian Cui
Logan Schneider
Robby Bryant
Ryan Gomes
Allen Jiang
Roy Lee
Javier Perez
Jamie Rogers
Cathy Speed
Shyam Tailor
Megan Walker
Jeffrey Yu
Tim Althoff
Conor Heneghan
Mark Malhotra
Shwetak Patel
Shravya Shetty
Jiening Zhan
Yeswanth Subramanian
Daniel McDuff
arXiv (2024)
Preview abstract
Large language models (LLMs) can retrieve, reason over, and make inferences about a wide range of information. In health, most LLM efforts to date have focused on clinical tasks. However, mobile and wearable devices, which are rarely integrated into clinical tasks, provide a rich, continuous, and longitudinal source of data relevant for personal health monitoring. Here we present a new model, Personal Health Large Language Model (PH-LLM), a version of Gemini fine-tuned for text understanding and reasoning over numerical time-series personal health data for applications in sleep and fitness. To systematically evaluate PH-LLM, we created and curated three novel benchmark datasets that test 1) production of personalized insights and recommendations from measured sleep patterns, physical activity, and physiological responses, 2) expert domain knowledge, and 3) prediction of self-reported sleep quality outcomes. For the insights and recommendations tasks we created 857 case studies in sleep and fitness. These case studies, designed in collaboration with domain experts, represent real-world scenarios and highlight the model’s capabilities in understanding and coaching. Through comprehensive human and automatic evaluation of domain-specific rubrics, we observed that both Gemini Ultra 1.0 and PH-LLM are not statistically different from expert performance in fitness and, while experts remain superior for sleep, fine-tuning PH-LLM provided significant improvements in using relevant domain knowledge and personalizing information for sleep insights. To further assess expert domain knowledge, we evaluated PH-LLM performance on multiple choice question examinations in sleep medicine and fitness. PH-LLM achieved 79% on sleep (N=629 questions) and 88% on fitness (N=99 questions), both of which exceed average scores from a sample of human experts as well as benchmarks for receiving continuing credit in those domains. To enable PH-LLM to predict self-reported assessments of sleep quality, we trained the model to predict self-reported sleep disruption and sleep impairment outcomes from textual and multimodal encoding representations of wearable sensor data. We demonstrate that multimodal encoding is both necessary and sufficient to match performance of a suite of discriminative models to predict these outcomes. Although further development and evaluation are necessary in the safety-critical personal health domain, these results demonstrate both the broad knowledge base and capabilities of Gemini models and the benefit of contextualizing physiological data for personal health applications as done with PH-LLM.
View details
SimPer: Simple Self-Supervised Learning of Periodic Targets
Yuzhe Yang
Jiang Wu
Dina Katabi
Ming-Zher Poh
Daniel McDuff
International Conference on Learning Representations (ICLR) (2023)
Preview abstract
From human physiology to environmental evolution, important processes in nature often exhibit meaningful and strong periodic or quasi-periodic changes. Due to their inherent label scarcity, learning useful representations for periodic tasks with limited or no supervision is of great benefit. Yet, existing self-supervised learning (SSL) methods overlook the intrinsic periodicity in data, and fail to learn representations that capture periodic or frequency attributes. In this paper, we present SimPer, a simple contrastive SSL regime for learning periodic information in data. To exploit the periodic inductive bias, SimPer introduces customized augmentations, feature similarity measures, and a generalized contrastive loss for learning efficient and robust periodic representations. Extensive experiments on common real-world tasks in human behavior analysis, environmental sensing, and healthcare domains verify the superior performance of SimPer compared to state-of-the-art SSL methods, highlighting its intriguing properties including better data efficiency, robustness to spurious correlations, and generalization to distribution shifts.
View details
Large Language Models are Few-Shot Health Learners
Daniel McDuff
Isaac Galatzer-Levy
Jake Sunshine
Jiening Zhan
Ming-Zher Poh
Shun Liao
Paolo Di Achille
Shwetak Patel
ArXiv (2023)
Preview abstract
Large language models (LLMs) can capture rich representations of concepts that are useful for real-world tasks. However, language alone is limited. While existing LLMs excel at text-based inferences, health applications require that models be grounded in numerical data (e.g., vital signs, laboratory values in clinical domains; steps, movement in the wellness domain) that is not easily or readily expressed as text in existing training corpus. We demonstrate that with only few-shot tuning, a large language model is capable of grounding various physiological and behavioral time-series data and making meaningful inferences on numerous health tasks for both clinical and wellness contexts. Using data from wearable and medical sensor recordings, we evaluate these capabilities on the tasks of cardiac signal analysis, physical activity recognition, metabolic calculation (e.g., calories burned), and estimation of stress reports and mental health screeners.
View details