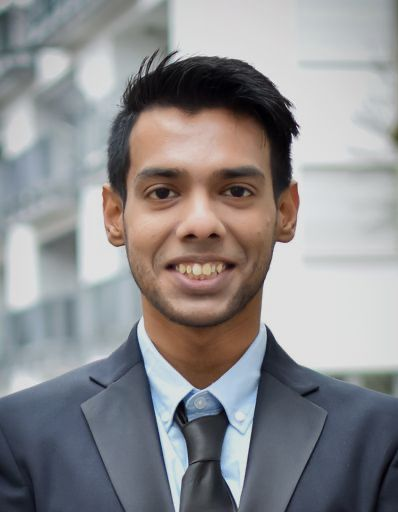
Kripasindhu Sarkar
Kripasindhu Sarkar is a Research Scientist in the AR perception group at Google, where he works on photorealistic rendering of humans and human centric vision in the context of AR and VR. Prior to Google, he was a postdoctoral researcher in the Visual Computing and AI department of Prof. Christian Theobalt at Max-Planck Institute for Informatics, and he obtained his PhD under Prof. Didier Stricker at German Research Center for Artificial Intelligence (DFKI) Kaiserslautern. He got his bachelor and masters degrees from the Indian Institute of Technology Kharagpur, India (IIT Kharagpur).
Research Areas
Authored Publications
Sort By
Google
Learning Personalized High Quality Volumetric Head Avatars from Monocular RGB Videos
Ziqian Bai
Danhang "Danny" Tang
Di Qiu
Abhimitra Meka
Mingsong Dou
Ping Tan
Thabo Beeler
2023 IEEE/CVF Conference on Computer Vision and Pattern Recognition (CVPR), IEEE