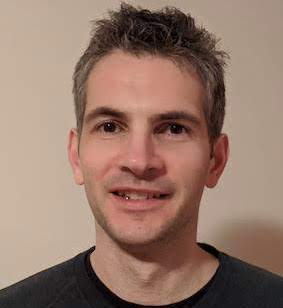
Matt Sharifi
Research Areas
Authored Publications
Sort By
MusicLM: Generating Music From Text
Andrea Agostinelli
Mauro Verzetti
Antoine Caillon
Qingqing Huang
Neil Zeghidour
Christian Frank
under review(2023)
Preview abstract
We introduce MusicLM, a model generating high-fidelity music from text descriptions such as "a calming violin melody backed by a distorted guitar riff". MusicLM casts the process of conditional music generation as a hierarchical sequence-to-sequence modeling task, and it generates music at 24 kHz that remains consistent over several minutes. Our experiments show that MusicLM outperforms previous systems both in audio quality and adherence to the text description. Moreover, we demonstrate that MusicLM can be conditioned on both text and a melody in that it can transform whistled and hummed melodies according to the style described in a text caption. To support future research, we publicly release MusicCaps, a dataset composed of 5.5k music-text pairs, with rich text descriptions provided by human experts.
Further links: samples, MusicCaps dataset
View details
Preview abstract
We propose SpeechPainter, a model for filling in gaps of up to one second in speech samples by leveraging an auxiliary textual input. We demonstrate that the model performs speech inpainting with the appropriate content, while maintaining speaker identity, prosody and recording environment conditions, and generalizing to unseen speakers. Our approach significantly outperforms baselines constructed using adaptive TTS, as judged by human raters in side-by-side preference and MOS tests.
View details
SPICE: Self-supervised pitch estimation
Christian Frank
Dominik Roblek
Mihajlo Velimirović
IEEE Transactions on Audio Speech and Language Processing (to appear)(2020)
Preview abstract
We propose a model to estimate the fundamental frequency in monophonic audio,
often referred to as pitch estimation. We acknowledge the fact that obtaining
ground truth annotations at the required temporal and frequency resolution is a
particularly daunting task. Therefore, we propose to adopt a self-supervised
learning technique, which is able to estimate pitch without any form
of supervision. The key observation is that pitch shift maps to a simple
translation when the audio signal is analysed through the lens of the constant-Q
transform (CQT). We design a self-supervised task by feeding two shifted slices
of the CQT to the same convolutional encoder, and require that the difference in
the outputs is proportional to the corresponding difference in pitch. In
addition, we introduce a small model head on top of the encoder, which is able
to determine the confidence of the pitch estimate, so as to distinguish between
voiced and unvoiced audio. Our results show that the proposed method is able to
estimate pitch at a level of accuracy comparable to fully supervised models,
both on clean and noisy audio samples, although it does not require access to large
labeled datasets.
View details
Training Keyword Spotters with Limited and Synthesized Speech Data
Dominik Roblek
James Lin
International Conference on Acoustics, Speech, and Signal Processing, IEEE, Barcelona, Spain(2020)
Preview abstract
With the rise of low power speech-enabled devices, there is a growing demand to quickly produce models for recognizing arbitrary sets of keywords. As with many machine learning tasks, one of the most challenging parts in the model creation process is obtaining a sufficient amount of high training data. In this paper, we explore the effectiveness of synthesized speech data in training small spoken term detection models. Instead of training such models directly on the audio or low level feature such as MFCCs we use a small speech embedding model trained to extract useful features for keyword spotting models. Using this embedding, we show that such a model for detecting 10 keywords when trained on only synthetic speech is equivalent to a model trained on over 50 real examples, and to a model trained on 4000 real examples if we do not use the speech embeddings.
View details
Preview abstract
We propose the Fréchet Audio Distance (FAD), a novel, reference-free evaluation metric for music enhancement algorithms. We demonstrate how typical evaluation metrics for speech enhancement and blind source separation can fail to accurately measure the perceived effect of a wide variety of distortions. As an alternative, we propose adapting the Fréchet Inception Distance (FID) metric used to evaluate generative image models to the audio domain. FAD is validated using a wide variety of artificial distortions and is compared to the signal based metrics signal to distortion ratio (SDR), cosine distance and magnitude L2 distance. We show that, with a correlation coefficient of 0.52, FAD correlates more closely with human perception than either SDR, cosine distance or magnitude L2 distance, with correlation coefficients of 0.39, -0.15 and -0.01 respectively.
View details
Now Playing: Continuous low-power music recognition
Dominik Roblek
James David Lyon
Julian James Odell
Mihajlo Velimirović
NIPS 2017 Workshop: Machine Learning on the Phone
Preview abstract
Existing music recognition applications require both user activation and a connection to a server that performs the actual recognition. In this paper we present a low power music recognizer that runs entirely on a mobile phone and automatically recognizes music without requiring any user activation. A small music detector runs continuously on the mobile phone’s DSP (digital signal processor) chip and only wakes main the processor when it is confident that music is present. Once woken the detector on the main processor is provided with an 8s buffer of audio which is then fingerprinted and compared to the stored fingerprints in the on-device fingerprint database of over 70000 songs.
View details