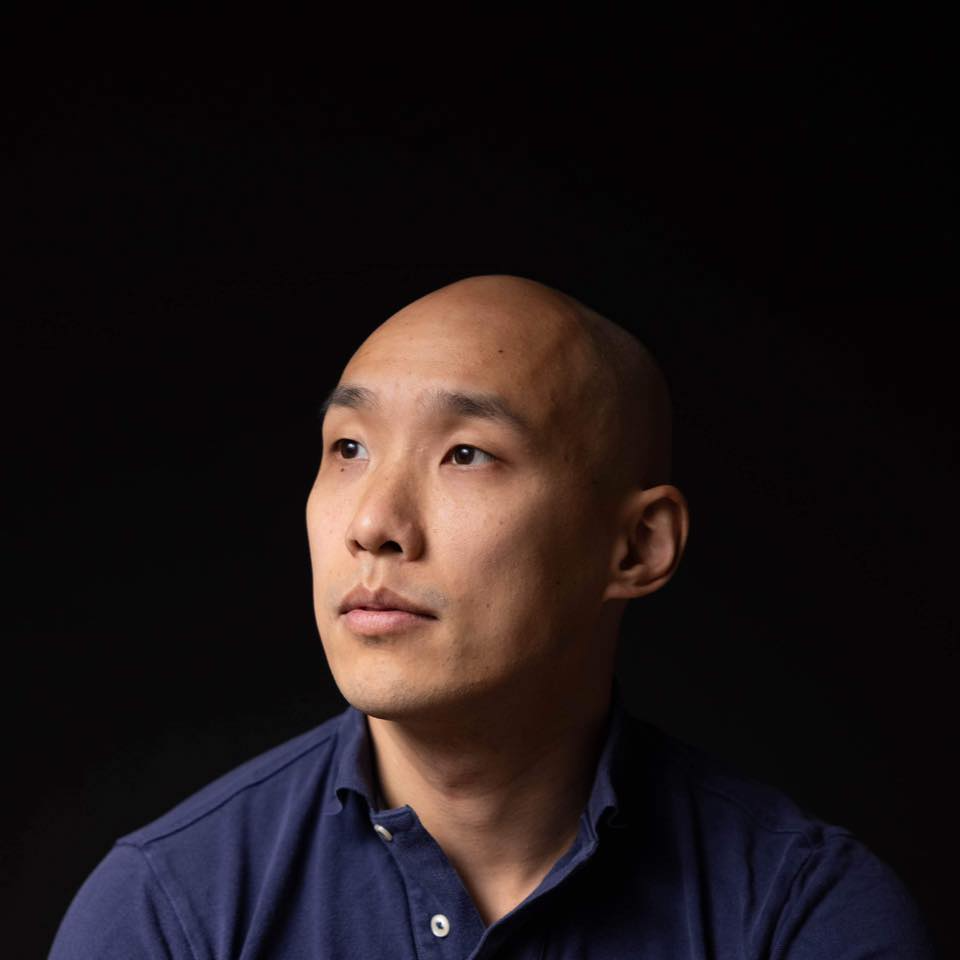
Daniel C. Suo
Authored Publications
Sort By
Adaptive Gradient Methods at the Edge of Stability
Behrooz Ghorbani
David Cardoze
Jeremy Cohen
Justin Gilmer
Shankar Krishnan
NeuRIPS 2022(2022) (to appear)
Preview abstract
Little is known about the training dynamics of adaptive gradient methods like Adam in deep learning. In this paper, we shed light on the behavior of these algorithms in the full-batch and sufficiently large batch settings. Specifically, we show that during full-batch training, the maximum eigenvalue of the \emph{preconditioned} Hessian typically equilibrates at the stability threshold of a related non-adaptive algorithm. For Adam with step size $\eta$ and $\beta_1 = 0.9$, this stability threshold is $38/\eta$. Similar effects occur during minibatch training, especially as the batch size grows. Yet, even though adaptive methods train at the “Edge of Stability,” their behavior in this regime differs in a crucial way from that of their non-adaptive counterparts. Whereas non-adaptive algorithms are forced to remain in low-curvature regions of the loss landscape, we demonstrate that adaptive gradient methods often advance into high-curvature regions, while adapting the preconditioner to compensate. We believe that our findings will serve as a foundation for the community’s future understanding of adaptive gradient methods in deep learning.
View details
Machine learning for medical ventilator control
Cyril Zhang
Daniel Cohen
Edgar Minasyan
Elad Hazan
Julienne LaChance
Karan Singh
Manuel Schottdorf
Paula Nicoleta Gradu
Tom Zajdel
Udaya Ghai
ML4H(2021) (to appear)
Preview abstract
We consider the problem of controlling a medical ventilator for pressure controlled ventilation. The goal is to control airflow in and out of a sedated patient’s lung ac-cording to a trajectory of airway pressures specified by a clinician.
PID, either hand-tuned or using lung-breath simulators based on gas dynamics, is the state-of-the-art control for ventilators.
We consider a data-driven machine learning methodology to tackle this problem via first training a simulator based on collected data and then using this simulator to train controllers based on artificial neural networks. We show that our controller is able to track significantly better than PID controllers on FDA specified benchmarks.
View details
Deluca -- A Differentiable Control Library: Environments, Methods, and Benchmarking
Alex Jiawen Yu
Anirudha Majumdar
Cyril Zhang
John Olof Hallman
Karan Singh
Paula Nicoleta Gradu
Udaya Ghai
Differentiable computer vision, graphics, and physics in machine learning workshop at Neurips 2020(2020) (to appear)
Preview abstract
We present an open-source library of natively differentiable physics and robotics environments, accompanied by gradient-based control methods and a benchmarking suite.
The introduced environments allow auto-differentiation through the simulation dynamics, and thereby permit fast training of controllers. The library features several popular environments, including classical control settings from OpenAI Gym \cite{brockman2016openai}. We also provide a novel differentiable environment, based on deep neural networks, that simulates medical ventilation.
We give several use-cases of new scientific results obtained using the library. This includes a medical ventilator simulator and controller, an adaptive control method for time-varying linear dynamical systems, and new gradient-based methods for control of linear dynamical systems with adversarial perturbations.
View details