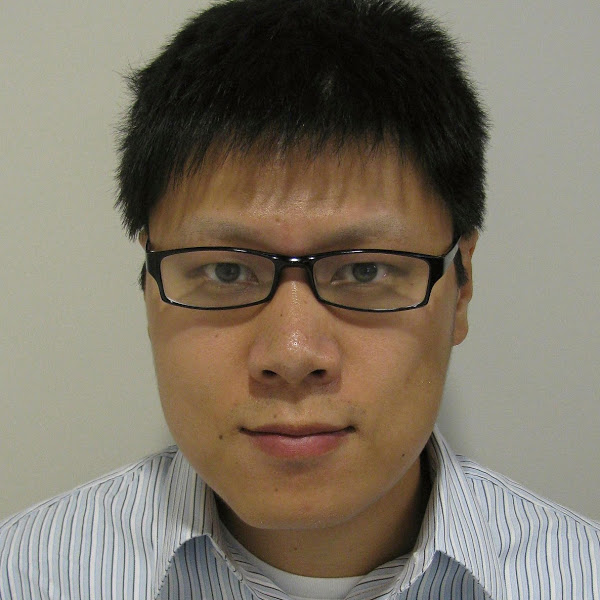
Renshen Wang
Research Areas
Authored Publications
Sort By
Google
FormNetV2: Inductive Multimodal Graph Contrastive Learning for Form Document Information Extraction
Chun-Liang Li
Hao Zhang
Xiang Zhang
Kihyuk Sohn
Nikolai Glushnev
Joshua Ainslie
Nan Hua
ACL (2023)
Text Reading Order in Uncontrolled Conditions by Sparse Graph Segmentation
International Conference on Document Analysis and Recognition (ICDAR) (2023) (to appear)
FormNet: Structural Encoding beyond Sequential Modeling in Form Document Information Extraction
Chun-Liang Li
Nan Hua
Joshua Ainslie
Association for Computational Linguistics (ACL) (2022)
Unified Line and Paragraph Detection by Graph Convolutional Networks
International Workshop on Document Analysis System (DAS) (2022)
Post-OCR Paragraph Recognition by Graph Convolutional Networks
Winter Conference on Applications of Computer Vision (WACV) 2022
ROPE: Reading Order Equivariant Positional Encoding for Graph-based Document Information Extraction
Chun-Liang Li
Chu Wang
Association for Computational Linguistics (ACL) (2021)