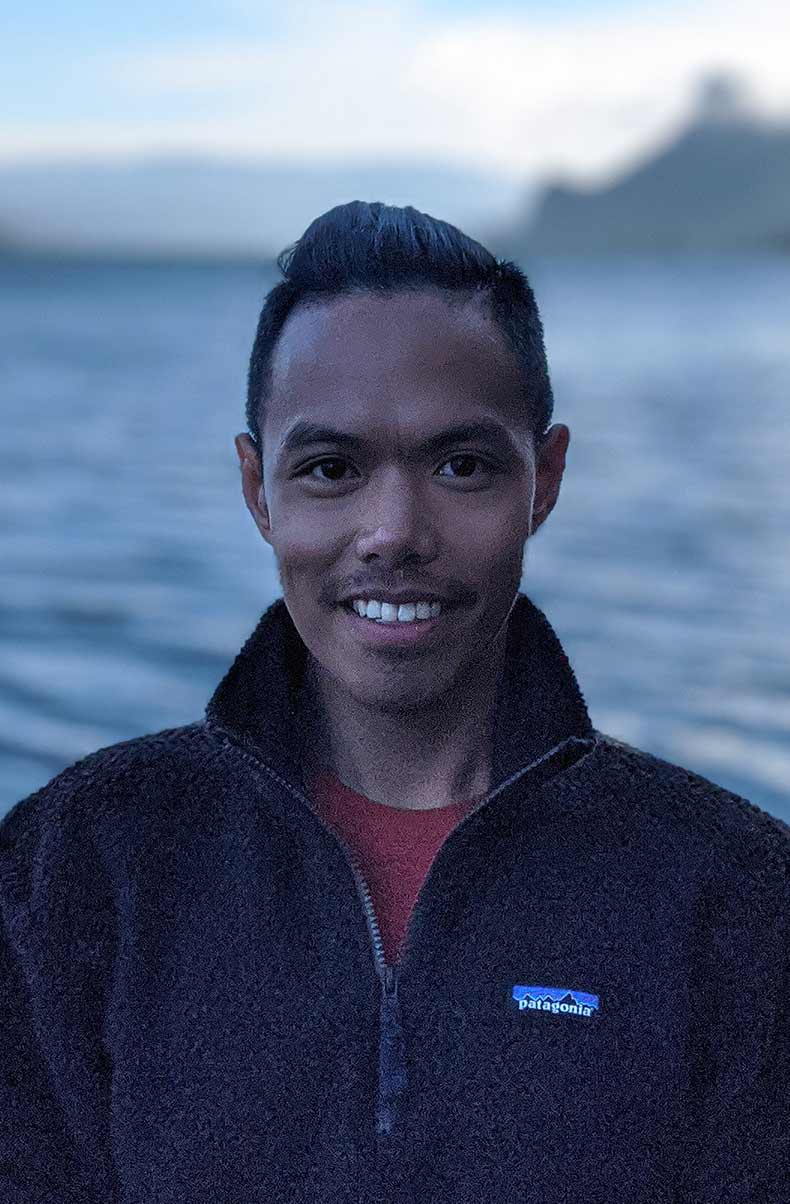
Oliver Siy
Authored Publications
Sort By
Relational Affect in Dyadic Interactions
CHI Conference on Human Factors in Computing Systems (2024)
Preview abstract
Relational affect is the affective response (encompassing emotion, expression, feeling) that emerges from an interaction between two people. The case study presented here introduces the concept of relational affect through a human perceptual rating task. Forty-five raters watched short video clips of two people interacting and described their perceived emotion of the individuals and that of the overall interaction. Our qualitative analysis of the rater responses showed that raters used a variety of schemes to reason about emotion, including expressions, context, and perceived appraisal of the event. These reasoning schemes were notably different for perceived individual emotion and relational affect. Our findings show that the vocabulary use for relational affect is distinct from that of individual emotion and relational affect as a phenomenon deepens our understanding of social interactions and moves the field a step closer to realizing the goal of fluid interactions between people and technology.
View details
"Because AI is 100% right and safe": User Attitudes and Sources of AI Authority in India
Shivani Kapania
Gabe Clapper
Azhagu SP
Nithya Sambasivan
CHI Conference on Human Factors in Computing Systems (CHI '22), ACM (2022) (to appear)
Preview abstract
Most prior work on human-AI interaction is set in communities that indicate skepticism towards AI, but we know less about contexts where AI is viewed as aspirational. We investigated the perceptions around AI systems by drawing upon 32 interviews and 459 survey respondents in India. Not only do Indian users accept AI decisions (79.2% respondents indicate acceptance), we find a case of AI authority--- AI has a legitimized power to influence human actions, without requiring adequate evidence about the capabilities of the system. AI authority manifested into four user attitudes of vulnerability: faith, forgiveness, self-blame, and gratitude, pointing to higher tolerance for system misfires, and introducing potential for irreversible individual and societal harm. We urgently call for calibrating AI authority, reconsidering success metrics and responsible AI approaches and present methodological suggestions for research and deployments in India.
View details
Preview abstract
Two decades ago, the advent of competency-based medical education (CBME) marked a paradigm shift in assessment. Now, medical education is on the cusp of another transformation driven by advances in the field of artificial intelligence (AI). In this article, the authors explore the potential value of AI in advancing CBME and entrustable professional activities by shifting the focus of education from assessment of learning to assessment for learning. The thoughtful integration of AI technologies in observation is proposed to aid in restructuring our current system around the goal of assessment for learning by creating continuous, tight feedback loops that were not before possible. The authors argued that this personalized and less judgmental relationship between learner and machine could shift today’s dominating mindset on grades and performance to one of growth and mastery learning that leads to expertise. However, because AI is neither objective nor value free, the authors stress the need for continuous co-production and evaluation of the technology with geographically and culturally diverse stakeholders to define desired behavior of the machine and assess its performance.
View details
Adapting User Experience Research Methods for AI-Driven Experiences
Kira Awadalla
Gregorio Convertino
Elizabeth Churchill
ACM CHI 2020 (2020)
Preview abstract
This short paper describes how to adapt user experience research methods for artificial intelligence (AI)-driven applications. Presently, there is a dearth of guidance for conducting UX research on AI-driven experiences. We describe what makes this class of experiences unique, propose a preliminary foundational framework to categorize AI-driven experiences, and within the framework we show an example of methodological adaptations via a case study.
View details