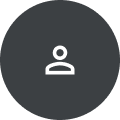
Weilong Yang
Weilong Yang is a senior staff software engineer, and a Tech Lead / Manager in Machine Perception at Google Research. He and his team are exploring new methods of applying AI in content creation, e.g., design creation, image / video ads generation, video highlight selection, thumbnail image generation, etc. Dr. Yang is involved in organizing the AI for content creation workshop in CVPR 2020, and he has served in the program committees of CVPR, ECCV, ICCV, and NIPS for many years. His research interests include deep learning, generative model, video classification, etc.
Research Areas
Authored Publications
Sort By
Google
Pose Embeddings: A Deep Architecture for Learning to Match Human Poses
Greg Mori
Nisarg Kothari
Alexander Toshev
arXiv (2015)