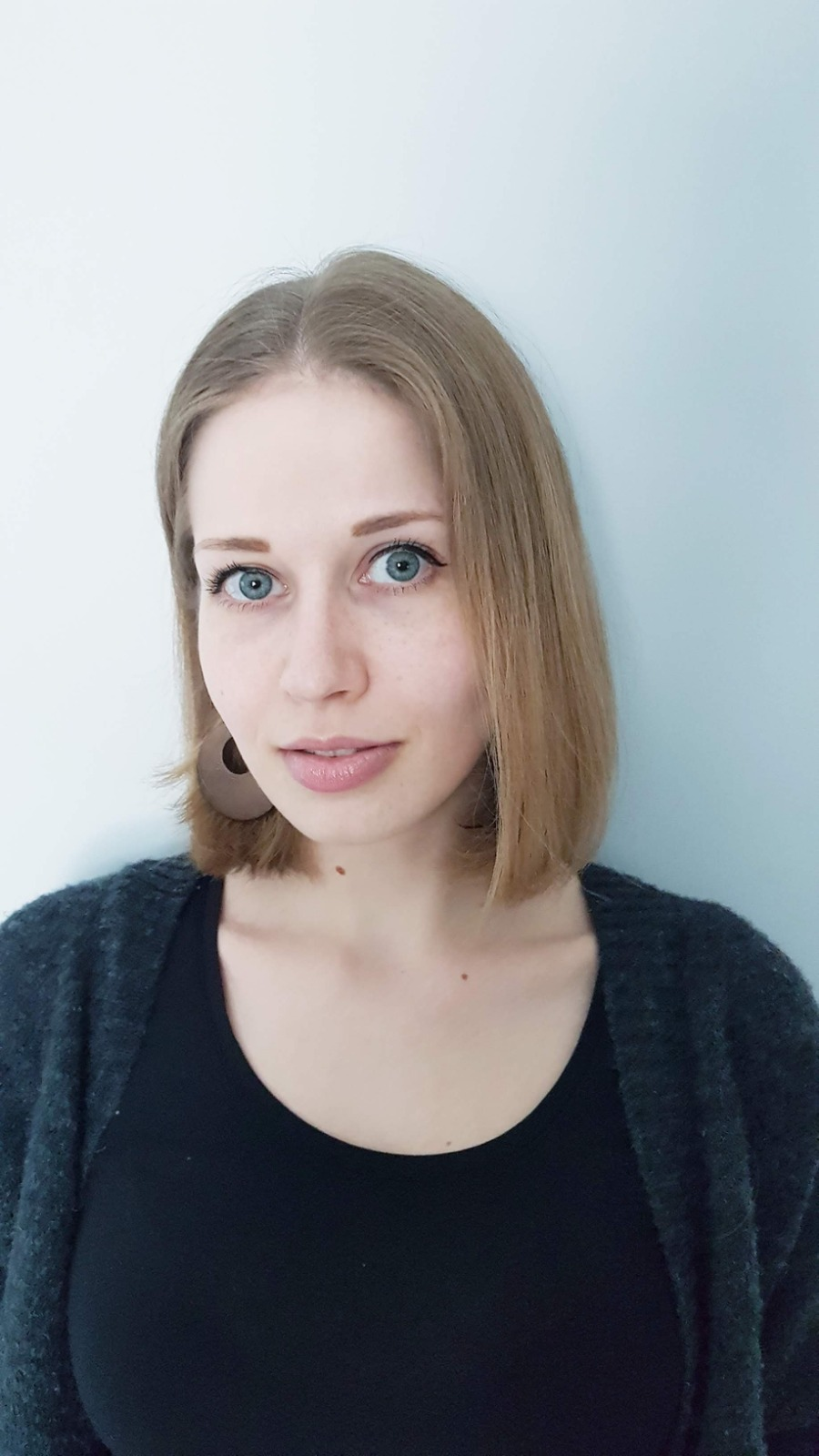
Alëna Aksënova
Alëna is a linguist working on automatic speech recognition. Prior to joining Google, she focused on formal language theory and its applications.
Authored Publications
Sort By
Accented Speech Recognition: Benchmarking, Pre-training, and Diverse Data
Zhehuai Chen
Chung-Cheng Chiu
Pavel Golik
Wei Han
Levi King
Suzan Schwartz
(2022)
Preview abstract
Building inclusive speech recognition systems is a crucial step towards developing technologies that speakers of all language varieties can use. Therefore, ASR systems must work for everybody independently of the way they speak. To accomplish this goal, there should be available data sets representing language varieties, and also an understanding of model configuration that is the most helpful in achieving robust understanding of all types of speech. However, there are not enough data sets for accented speech, and for the ones that are already available, more training approaches need to be explored to improve the quality of accented speech recognition. In this paper, we discuss recent progress towards developing more inclusive ASR systems, namely, the importance of building new data sets representing linguistic diversity, and exploring novel training approaches to improve performance for all users. We address recent directions within benchmarking ASR systems for accented speech, measure the effects of wav2vec 2.0 pre-training on accented speech recognition, and highlight corpora relevant for diverse ASR evaluations.
View details
How Might We Create Better Benchmarks for Speech Recognition?
James Flynn
Pavel Golik
ACL-IJCNLP 2021 Workshop on Benchmarking: Past, Present and Future(2021)
Preview abstract
The applications of automatic speech recognition (ASR) systems are proliferating, in part due to recent significant quality improvements. However, as recent work indicates, even state-of-the-art speech recognition systems – some which deliver impressive benchmark results, struggle to generalize across use cases. We review relevant work, and, hoping to inform future benchmark development, outline a taxonomy of speech recognition use cases, proposed for the next generation of ASR benchmarks. We also survey work on metrics, in addition to the de facto standard Word Error Rate (WER) metric, and we introduce a versatile framework designed to describe interactions between linguistic variation and ASR performance metrics.
View details
Preview abstract
This paper proposes a grammatical inference algorithm to learn a formal class of input-sensitive tier-based strictly local languages across multiple tiers from positive data only, when the locality of the tier-constraints and of the tier-projection function is set to two (MITSL-2,2; De Santo and Graf, 2019). We then conduct simulations showing that the algorithm succeeds in learning MITSL-2,2 from an initial set of artificial languages.
View details
Preview abstract
In this paper, we use a novel algorithmic approach to explore dialectal variation in American English speech. Without the need for human phonemic annotations, we are able to use an existing corpus transcribed in text form only. Our results show that, in general, American English dialects can be divided into two larger groups: dialects of the South (Texas to North Carolina except for peninsular Florida), and the rest of the country. Our results confirm some well-known results from dialectology, such as the pin-pen merger, but show that some other ones, such as the cot-caught merger, may be losing their isogloss boundaries. Moreover, we demonstrate that our algorithm can extend to dialectal features in other languages.
View details