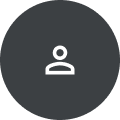
Tongzhou Chen
Research Areas
Authored Publications
Sort By
Google
Multilingual Second-Pass Rescoring for Automatic Speech RecognitionSystems
Pedro Moreno Mengibar
ICASSP (2022)
On Weight Interpolation of the Hybrid Autoregressive Transducer Model
David Rybach
Interspeech 2022, Interspeech 2022 (2022) (to appear)
Mixture Model Attention: Flexible Streaming and Non-Streaming Automatic Speech Recognition
Pedro Jose Moreno Mengibar
Proceedings of Interspeech, 2021 (to appear)