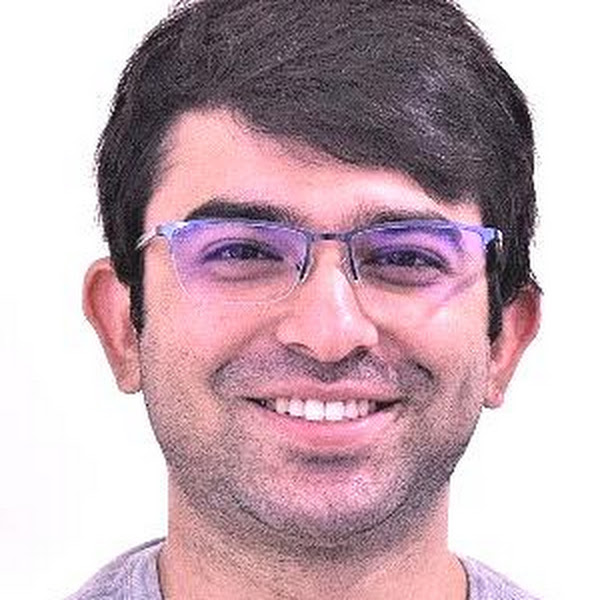
Divy Thakkar
I am an HCI researcher with a focus on Human-AI interactions in low-resource communities. My prior research has examined AI for Social Good for marginalised communities, Future of Work, Algorithmic Fairness of education/ job Platforms and the inequitable computing scenario in India.
Research Areas
Authored Publications
Sort By
Google
Public Health Calls for/with AI: An Ethnographic Perspective
Azra Ismail
Neha Kumar
Neha Madhiwalla
ACM Conference On Computer-Supported Cooperative Work And Social Computing (2023)
Deployed SAHELI: Field Optimization of Intelligent RMAB for Maternal and Child Care
Shresth Verma
Aditya S. Mate
Paritosh Verma
Sruthi Gorantala
Neha Madhiwalla
Aparna Hegde
Manish Jain
Innovative Applications of Artificial Intelligence (IAAI) (2023) (to appear)
Facilitating Human-Wildlife Cohabitation through Conflict Prediction
Susobhan Ghosh
Pradeep Varakantham
Aniket Bhatkhande
Tamanna Ahmad
Anish Andheria
Wenjun Li
IAAI Technical Track on Emerging Applications of AI (2022)
When is ML data good?: Valuing in Public Health Datafication
Azra Ismail
Pratyush Kumar
Alex Hanna
Nithya Sambasivan
Neha Kumar
CHI 2022 (2022) (to appear)
Measuring Data Collection Diligence for Community Healthcare
Ramesha Karunasena
Md Sarfrazul Ambiya
Arunesh Sinha
Ruchit Nagar
Dhyanesh Narayanan
ACM conference on Equity and Access in Algorithms, Mechanisms, and Optimization 2021 (2021)
Towards an AI-Powered Future that Works for Vocational Workers
Neha Kumar
Nithya Sambasivan
Conference on Human Factors in Computing Systems, Proceedings of the 2020 CHI Conference on Human Factors in Computing Systems (2020)
The Unexpected Entry and Exodus of Women in Computing and HCI in India
Nithya Sambasivan*
Purva Yardi
Pratap Kalenahalli Sudarshan
Kentaro Toyama
SIGCHI, ACM (2018)