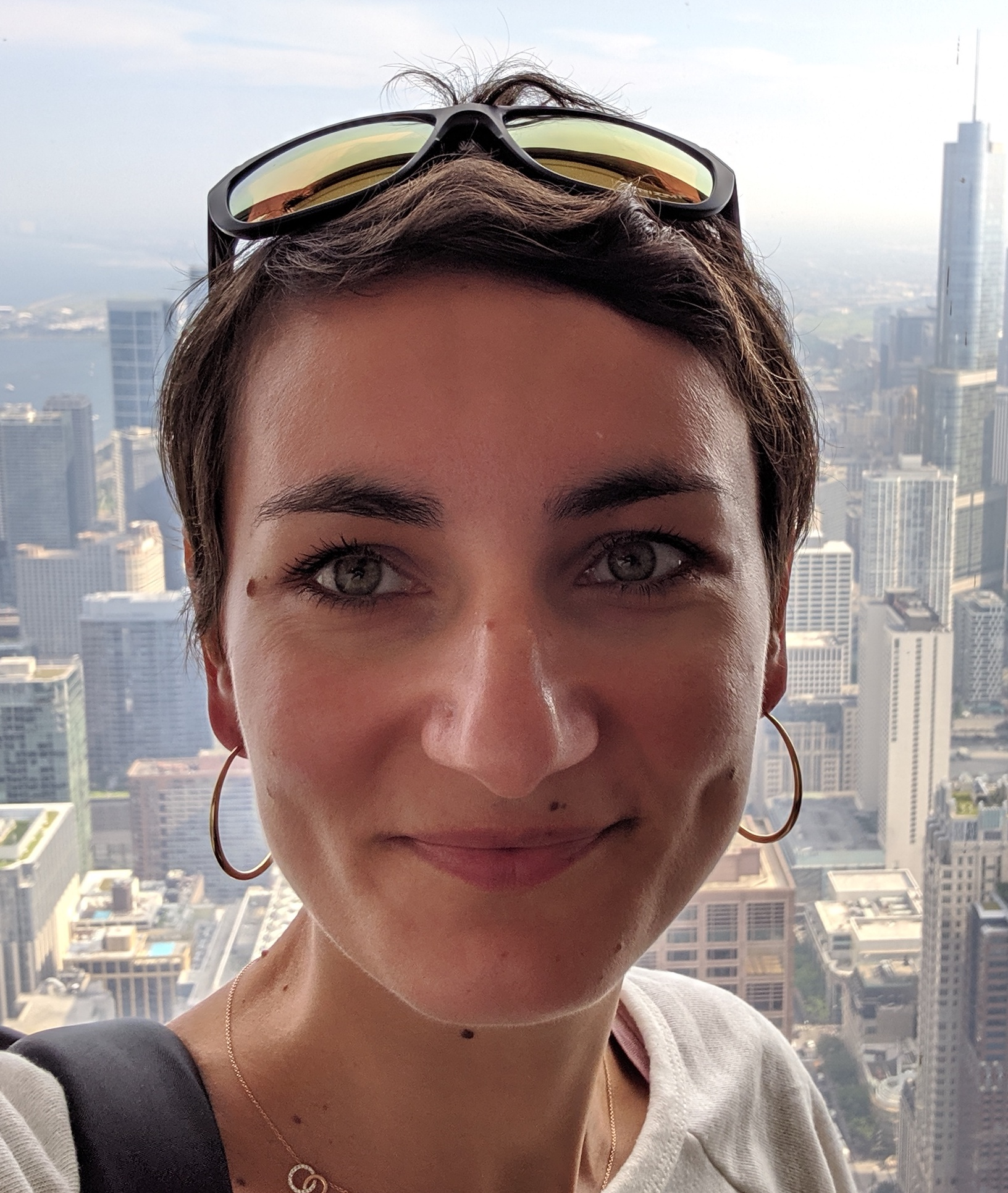
Monika Podsiadło
Research Areas
Authored Publications
Sort By
Preview abstract
Common text-to-speech (TTS) systems rely on training data
for modelling human speech. The quality of this data can
range from professional voice actors recording hand-curated
sentences in high-quality studio conditions, to found voice data
representing arbitrary domains. For years, the unit selection
technology dominant in the field required many hours of data
that was expensive and time-consuming to collect. With the advancement
of statistical methods of waveform generation, there
have been experiments with more noisy and often much larger
datasets (“big data”), testing the inherent flexibility of such systems.
In this paper we examine the relationship between training
data and speech synthesis quality. We then hypothesise that
statistical text-to-speech benefits from high acoustic quality corpora
with high level of prosodic variation, but that beyond the
first few hours of training data we don’t observe quality gains.
We then describe how we engineered a training dataset containing
optimized distribution of features, and how these features
were defined. Lastly, we present results from a series of
evaluation tests. These confirm our hypothesis and show how
a carefully engineered training corpus of a smaller size yields
the same speech quality as much larger datasets, particularly
for voices that use WaveNet.
View details
Preview abstract
Individuals with vision loss use text-to-speech (TTS) for most
of their interaction with devices, and rely on the quality of syn-
thetic voices to a much larger extent than any other user group.
In total, 33% of local synthesis requests for Google TTS come
from TalkBack, the Android screenreader, making it our top
client and making the visually-impaired users the heaviest con-
sumers of the technology. Despite this, very little attention has
been devoted to optimizing TTS voices for this user group and
the feedback on TTS voices from the blind has been tradition-
ally less-favourable. We present the findings from a TTS user
experience study conducted by Google with visually-impaired
screen reader users. The study comprised 14 focus groups and
evaluated a total of 95 candidate voices with 90 participants
across 3 countries. The study uncovered the distinctitve us-
age patterns of this user group, which point to different TTS
requirements and voice preferences from those of sighted users.
View details
Preview abstract
Modern Text-To-Speech (TTS) systems need to increasingly deal with multilingual input. Navigation, social and news are all domains with a large proportion of foreign words. However, when typical monolingual TTS voices are used, the synthesis quality on such input is markedly lower. This is because traditional TTS derives pronunciations from a lexicon or a Grapheme-To-Phoneme (G2P) model which was built using a pre-defined sound inventory and a phonotactic grammar for one language only. G2P models perform poorly on foreign words, while manual lexicon development is labour-intensive, expensive and requires extra storage. Furthermore, large phoneme inventories and phonotactic grammars contribute to data sparsity in unit selection systems. We present an automatic system for deriving pronunciations for foreign words that utilises the monolingual voice design and can rapidly scale to many languages. The proposed system, based on a neural network cross-lingual G2P model, does not increase the size of the voice database, doesn't require large data annotation efforts, is designed not to increase data sparsity in the voice, and can be sized to suit embedded applications.
View details