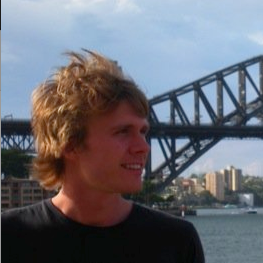
Joseph Kelly
Research Areas
Authored Publications
Sort By
Media Mix Model Calibration With Bayesian Priors
Mike Wurm
Brenda Price
Ying Liu
research.google(2024)
Preview abstract
Effective model calibration is a critical and indispensable component in developing Media Mix Models (MMMs). One advantage of Bayesian-based MMMs lies in their capacity to accommodate the information from experiment results and the modelers' domain knowledge about the ad effectiveness by setting priors for the model parameters. However, it remains ambiguous about how and which Bayesian priors should be tuned for calibration purpose. In this paper, we propose a new calibration method through model reparameterization. The reparameterized model includes Return on Ads Spend (ROAS) as a model parameter, enabling straightforward adjustment of its prior distribution to align with either experiment results or the modeler's prior knowledge. The proposed method also helps address several key challenges regarding combining MMMs and incrementality experiments. We use simulations to demonstrate that our approach can significantly reduce the bias and uncertainty in the resultant posterior ROAS estimates.
View details
Preview abstract
We introduce Predictive State Propensity Subclassification (PSPS), a
novel estimation method for undertaking causal inference from
observational studies. The methodology applies to both discrete and
continuous treatments and can estimate unit-level and population-level
average treatment effects. PSPS combines propensity and outcome models
into one encompassing probabilistic model, which can be jointly
estimated using maximum likelihood or Bayesian inference. We give a
detailed overview on the TensorFlow implementation for likelihood
optimization and show via large-scale simulations that it outperforms
several state of the art methods -- both in terms of bias and variance
for average as well as unit-level treatment effects. Finally we
illustrate the methodology and algorithms on standard datasets in the
literature.
View details
Preview abstract
In this paper we tackle the marketing problem of assigning credit for a successful outcome to events that occur prior to the success, otherwise known as the attribution problem. In the world of digital advertising, attribution is widely used to formulate and evaluate marketing but often without a clear specification of the measurement objective and the decision-making needs. We formalize the problem of attribution under a causal framework, note its shortcomings, and suggest an attribution algorithm evaluated via simulation.
View details