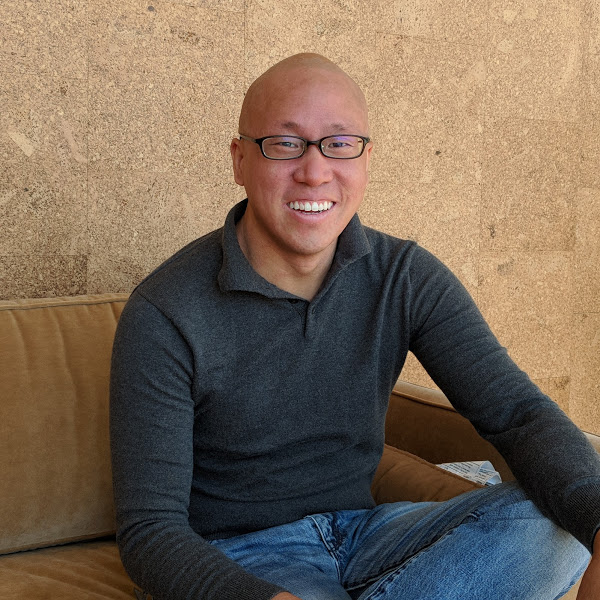
Daniel Tse
Research Areas
Authored Publications
Sort By
Triaging mammography with artificial intelligence: an implementation study
Sarah M. Friedewald
Sunny Jansen
Fereshteh Mahvar
Timo Kohlberger
David V. Schacht
Sonya Bhole
Dipti Gupta
Scott Mayer McKinney
Stacey Caron
David Melnick
Mozziyar Etemadi
Samantha Winter
Alejandra Maciel
Luca Speroni
Martha Sevenich
Arnav Agharwal
Rubin Zhang
Gavin Duggan
Shiro Kadowaki
Atilla Kiraly
Jie Yang
Basil Mustafa
Krish Eswaran
Shravya Shetty
Breast Cancer Research and Treatment (2025)
Preview abstract
Purpose
Many breast centers are unable to provide immediate results at the time of screening mammography which results in delayed patient care. Implementing artificial intelligence (AI) could identify patients who may have breast cancer and accelerate the time to diagnostic imaging and biopsy diagnosis.
Methods
In this prospective randomized, unblinded, controlled implementation study we enrolled 1000 screening participants between March 2021 and May 2022. The experimental group used an AI system to prioritize a subset of cases for same-visit radiologist evaluation, and same-visit diagnostic workup if necessary. The control group followed the standard of care. The primary operational endpoints were time to additional imaging (TA) and time to biopsy diagnosis (TB).
Results
The final cohort included 463 experimental and 392 control participants. The one-sided Mann-Whitney U test was employed for analysis of TA and TB. In the control group, the TA was 25.6 days [95% CI 22.0–29.9] and TB was 55.9 days [95% CI 45.5–69.6]. In comparison, the experimental group's mean TA was reduced by 25% (6.4 fewer days [one-sided 95% CI > 0.3], p<0.001) and mean TB was reduced by 30% (16.8 fewer days; 95% CI > 5.1], p=0.003). The time reduction was more pronounced for AI-prioritized participants in the experimental group. All participants eventually diagnosed with breast cancer were prioritized by the AI.
Conclusions
Implementing AI prioritization can accelerate care timelines for patients requiring additional workup, while maintaining the efficiency of delayed interpretation for most participants. Reducing diagnostic delays could contribute to improved patient adherence, decreased anxiety and addressing disparities in access to timely care.
View details
Prospective Multi-Site Validation of AI to Detect Tuberculosis and Chest X-Ray Abnormalities
Sahar Kazemzadeh
Atilla Kiraly
Nsala Sanjase
Minyoi Maimbolwa
Brian Shuma
Shahar Jamshy
Christina Chen
Arnav Agharwal
Chuck Lau
Daniel Golden
Jin Yu
Eric Wu
Kat Chou
Shravya Shetty
Krish Eswaran
Rory Pilgrim
Monde Muyoyeta
NEJM AI (2024)
Preview abstract
Background
Using artificial intelligence (AI) to interpret chest X-rays (CXRs) could support accessible triage tests for active pulmonary tuberculosis (TB) in resource-constrained settings.
Methods
The performance of two cloud-based CXR AI systems — one to detect TB and the other to detect CXR abnormalities — in a population with a high TB and human immunodeficiency virus (HIV) burden was evaluated. We recruited 1978 adults who had TB symptoms, were close contacts of known TB patients, or were newly diagnosed with HIV at three clinical sites. The TB-detecting AI (TB AI) scores were converted to binary using two thresholds: a high-sensitivity threshold and an exploratory threshold designed to resemble radiologist performance. Ten radiologists reviewed images for signs of TB, blinded to the reference standard. Primary analysis measured AI detection noninferiority to radiologist performance. Secondary analysis evaluated AI detection as compared with the World Health Organization (WHO) targets (90% sensitivity, 70% specificity). Both used an absolute margin of 5%. The abnormality-detecting AI (abnormality AI) was evaluated for noninferiority to a high-sensitivity target suitable for triaging (90% sensitivity, 50% specificity).
Results
Of the 1910 patients analyzed, 1827 (96%) had conclusive TB status, of which 649 (36%) were HIV positive and 192 (11%) were TB positive. The TB AI’s sensitivity and specificity were 87% and 70%, respectively, at the high-sensitivity threshold and 78% and 82%, respectively, at the balanced threshold. Radiologists’ mean sensitivity was 76% and mean specificity was 82%. At the high-sensitivity threshold, the TB AI was noninferior to average radiologist sensitivity (P<0.001) but not to average radiologist specificity (P=0.99) and was higher than the WHO target for specificity but not sensitivity. At the balanced threshold, the TB AI was comparable to radiologists. The abnormality AI’s sensitivity and specificity were 97% and 79%, respectively, with both meeting the prespecified targets.
Conclusions
The CXR TB AI was noninferior to radiologists for active pulmonary TB triaging in a population with a high TB and HIV burden. Neither the TB AI nor the radiologists met WHO recommendations for sensitivity in the study population. AI can also be used to detect other CXR abnormalities in the same population.
View details
Assistive AI in Lung Cancer Screening: A Retrospective Multinational Study in the United States and Japan
Atilla Kiraly
Corbin Cunningham
Ryan Najafi
Jie Yang
Chuck Lau
Diego Ardila
Scott Mayer McKinney
Rory Pilgrim
Mozziyar Etemadi
Sunny Jansen
Lily Peng
Shravya Shetty
Neeral Beladia
Krish Eswaran
Radiology: Artificial Intelligence (2024)
Preview abstract
Lung cancer is the leading cause of cancer death world-wide with 1.8 million deaths in 20201. Studies have concluded that low-dose computed tomography lung cancer screening can reduce mortality by up to 61%2 and updated 2021 US guidelines expanded eligibility. As screening efforts rise, AI can play an important role, but must be unobtrusively integrated into existing clinical workflows. In this work, we introduce a state-of-the-art, cloud-based AI system providing lung cancer risk assessments without requiring any user input. We demonstrate its efficacy in assisting lung cancer screening under both US and Japanese screening settings using different patient populations and screening protocols. Technical improvements over a previously described system include a focus on earlier cancer detection for improved accuracy, introduction of an effective assistive user interface, and a system designed to integrate into typical clinical workflows. The stand-alone AI system was evaluated on 3085 individuals achieving area under the curve (AUC) scores of 91.7% (95%CI [89.6, 95.2]), 93.3% (95%CI [90.2, 95.7]), and 89.1% (95%CI [77.7, 97.3]) on three datasets (two from US and one from Japan), respectively. To evaluate the system’s assistive ability, we conducted two retrospective multi-reader multi-case studies on 627 cases read by experienced board certified radiologists (average 20 years of experience [7,40]) using local PACS systems in the respective US and Japanese screening settings. The studies measured the reader’s level of suspicion (LoS) and categorical responses for scores and management recommendations under country-specific screening protocols. The radiologists’ AUC for LoS increased with AI assistance by 2.3% (95%CI [0.1-4.5], p=0.022) for the US study and by 2.3% (95%CI [-3.5-8.1], p=0.179) for the Japan study. Specificity for recalls increased by 5.5% (95%CI [2.7-8.5], p<0.0001) for the US and 6.7% (95%CI [4.7-8.7], p<0.0001) for the Japan study. No significant reduction in other metrics occured. This work advances the state-of-the-art in lung cancer detection, introduces generalizable interface concepts that can be applicable to similar AI applications, and demonstrates its potential impact on diagnostic AI in global lung cancer screening with results suggesting a substantial drop in unnecessary follow-up procedures without impacting sensitivity.
View details
Development of a Machine Learning Model for Sonographic Assessment of Gestational Age
Chace Lee
Angelica Willis
Christina Chen
Amber Watters
Bethany Stetson
Akib Uddin
Jonny Wong
Rory Pilgrim
Kat Chou
Shravya Ramesh Shetty
Ryan Gomes
JAMA Network Open (2023)
Preview abstract
Importance: Fetal ultrasonography is essential for confirmation of gestational age (GA), and accurate GA assessment is important for providing appropriate care throughout pregnancy and for identifying complications, including fetal growth disorders. Derivation of GA from manual fetal biometry measurements (ie, head, abdomen, and femur) is operator dependent and time-consuming.
Objective: To develop artificial intelligence (AI) models to estimate GA with higher accuracy and reliability, leveraging standard biometry images and fly-to ultrasonography videos.
Design, Setting, and Participants: To improve GA estimates, this diagnostic study used AI to interpret standard plane ultrasonography images and fly-to ultrasonography videos, which are 5- to 10-second videos that can be automatically recorded as part of the standard of care before the still image is captured. Three AI models were developed and validated: (1) an image model using standard plane images, (2) a video model using fly-to videos, and (3) an ensemble model (combining both image and video models). The models were trained and evaluated on data from the Fetal Age Machine Learning Initiative (FAMLI) cohort, which included participants from 2 study sites at Chapel Hill, North Carolina (US), and Lusaka, Zambia. Participants were eligible to be part of this study if they received routine antenatal care at 1 of these sites, were aged 18 years or older, had a viable intrauterine singleton pregnancy, and could provide written consent. They were not eligible if they had known uterine or fetal abnormality, or had any other conditions that would make participation unsafe or complicate interpretation. Data analysis was performed from January to July 2022.
Main Outcomes and Measures: The primary analysis outcome for GA was the mean difference in absolute error between the GA model estimate and the clinical standard estimate, with the ground truth GA extrapolated from the initial GA estimated at an initial examination.
Results: Of the total cohort of 3842 participants, data were calculated for a test set of 404 participants with a mean (SD) age of 28.8 (5.6) years at enrollment. All models were statistically superior to standard fetal biometry–based GA estimates derived from images captured by expert sonographers. The ensemble model had the lowest mean absolute error compared with the clinical standard fetal biometry (mean [SD] difference, −1.51 [3.96] days; 95% CI, −1.90 to −1.10 days). All 3 models outperformed standard biometry by a more substantial margin on fetuses that were predicted to be small for their GA.
Conclusions and Relevance: These findings suggest that AI models have the potential to empower trained operators to estimate GA with higher accuracy.
View details
ELIXR: Towards a general purpose X-ray artificial intelligence system through alignment of large language models and radiology vision encoders
Shawn Xu
Lin Yang
Christopher Kelly
Timo Kohlberger
Martin Ma
Atilla Kiraly
Sahar Kazemzadeh
Zakkai Melamed
Jungyeon Park
Patricia MacWilliams
Chuck Lau
Christina Chen
Mozziyar Etemadi
Sreenivasa Raju Kalidindi
Kat Chou
Shravya Shetty
Daniel Golden
Rory Pilgrim
Krish Eswaran
arxiv (2023)
Preview abstract
Our approach, which we call Embeddings for Language/Image-aligned X-Rays, or ELIXR, leverages a language-aligned image encoder combined or grafted onto a fixed LLM, PaLM 2, to perform a broad range of tasks. We train this lightweight adapter architecture using images paired with corresponding free-text radiology reports from the MIMIC-CXR dataset. ELIXR achieved state-of-the-art performance on zero-shot chest X-ray (CXR) classification (mean AUC of 0.850 across 13 findings), data-efficient CXR classification (mean AUCs of 0.893 and 0.898 across five findings (atelectasis, cardiomegaly, consolidation, pleural effusion, and pulmonary edema) for 1% (~2,200 images) and 10% (~22,000 images) training data), and semantic search (0.76 normalized discounted cumulative gain (NDCG) across nineteen queries, including perfect retrieval on twelve of them). Compared to existing data-efficient methods including supervised contrastive learning (SupCon), ELIXR required two orders of magnitude less data to reach similar performance. ELIXR also showed promise on CXR vision-language tasks, demonstrating overall accuracies of 58.7% and 62.5% on visual question answering and report quality assurance tasks, respectively. These results suggest that ELIXR is a robust and versatile approach to CXR AI.
View details
A mobile-optimized artificial intelligence system for gestational age and fetal malpresentation assessment
Ryan Gomes
Bellington Vwalika
Chace Lee
Angelica Willis
Joan T. Price
Christina Chen
Margaret P. Kasaro
James A. Taylor
Elizabeth M. Stringer
Scott Mayer McKinney
Ntazana Sindano
William Goodnight, III
Justin Gilmer
Benjamin H. Chi
Charles Lau
Terry Spitz
Kris Liu
Jonny Wong
Rory Pilgrim
Akib Uddin
Lily Hao Yi Peng
Kat Chou
Jeffrey S. A. Stringer
Shravya Ramesh Shetty
Communications Medicine (2022)
Preview abstract
Background
Fetal ultrasound is an important component of antenatal care, but shortage of adequately trained healthcare workers has limited its adoption in low-to-middle-income countries. This study investigated the use of artificial intelligence for fetal ultrasound in under-resourced settings.
Methods
Blind sweep ultrasounds, consisting of six freehand ultrasound sweeps, were collected by sonographers in the USA and Zambia, and novice operators in Zambia. We developed artificial intelligence (AI) models that used blind sweeps to predict gestational age (GA) and fetal malpresentation. AI GA estimates and standard fetal biometry estimates were compared to a previously established ground truth, and evaluated for difference in absolute error. Fetal malpresentation (non-cephalic vs cephalic) was compared to sonographer assessment. On-device AI model run-times were benchmarked on Android mobile phones.
Results
Here we show that GA estimation accuracy of the AI model is non-inferior to standard fetal biometry estimates (error difference -1.4 ± 4.5 days, 95% CI -1.8, -0.9, n=406). Non-inferiority is maintained when blind sweeps are acquired by novice operators performing only two of six sweep motion types. Fetal malpresentation AUC-ROC is 0.977 (95% CI, 0.949, 1.00, n=613), sonographers and novices have similar AUC-ROC. Software run-times on mobile phones for both diagnostic models are less than 3 seconds after completion of a sweep.
Conclusions
The gestational age model is non-inferior to the clinical standard and the fetal malpresentation model has high AUC-ROCs across operators and devices. Our AI models are able to run on-device, without internet connectivity, and provide feedback scores to assist in upleveling the capabilities of lightly trained ultrasound operators in low resource settings.
View details
Simplified Transfer Learning for Chest X-ray Models using Less Data
Christina Chen
AJ Maschinot
Jenny Huang
Chuck Lau
Sreenivasa Raju Kalidindi
Mozziyar Etemadi
Florencia Garcia-Vicente
David Melnick
Krish Eswaran
Neeral Beladia
Dilip Krishnan
Shravya Ramesh Shetty
Radiology (2022)
Preview abstract
Background: Developing deep learning models for radiology requires large data sets and substantial computational resources. Data set size limitations can be further exacerbated by distribution shifts, such as rapid changes in patient populations and standard of care during the COVID-19 pandemic. A common partial mitigation is transfer learning by pretraining a “generic network” on a large nonmedical data set and then fine-tuning on a task-specific radiology data set. Purpose: To reduce data set size requirements for chest radiography deep learning models by using an advanced machine learning approach (supervised contrastive [SupCon] learning) to generate chest radiography networks. Materials and Methods: SupCon helped generate chest radiography networks from 821 544 chest radiographs from India and the United States. The chest radiography networks were used as a starting point for further machine learning model development for 10 prediction tasks (eg, airspace opacity, fracture, tuberculosis, and COVID-19 outcomes) by using five data sets comprising 684 955 chest radiographs from India, the United States, and China. Three model development setups were tested (linear classifier, nonlinear classifier, and fine-tuning the full network) with different data set sizes from eight to 85. Results: Across a majority of tasks, compared with transfer learning from a nonmedical data set, SupCon reduced label requirements up to 688-fold and improved the area under the receiver operating characteristic curve (AUC) at matching data set sizes. At the extreme low-data regimen, training small nonlinear models by using only 45 chest radiographs yielded an AUC of 0.95 (noninferior to radiologist performance) in classifying microbiology-confirmed tuberculosis in external validation. At a more moderate data regimen, training small nonlinear models by using only 528 chest radiographs yielded an AUC of 0.75 in predicting severe COVID-19 outcomes. Conclusion: Supervised contrastive learning enabled performance comparable to state-of-the-art deep learning models in multiple clinical tasks by using as few as 45 images and is a promising method for predictive modeling with use of small data sets and for predicting outcomes in shifting patient populations.
View details
Deep Learning Detection of Active Pulmonary Tuberculosis at Chest Radiography Matched the Clinical Performance of Radiologists
Sahar Kazemzadeh
Jin Yu
Shahar Jamshy
Rory Pilgrim
Christina Chen
Neeral Beladia
Chuck Lau
Scott Mayer McKinney
Thad Hughes
Atilla Peter Kiraly
Sreenivasa Raju Kalidindi
Monde Muyoyeta
Jameson Malemela
Ting Shih
Lily Hao Yi Peng
Kat Chou
Cameron Chen
Krish Eswaran
Shravya Ramesh Shetty
Radiology (2022)
Preview abstract
Background: The World Health Organization (WHO) recommends chest radiography to facilitate tuberculosis (TB) screening. However, chest radiograph interpretation expertise remains limited in many regions. Purpose: To develop a deep learning system (DLS) to detect active pulmonary TB on chest radiographs and compare its performance to that of radiologists. Materials and Methods: A DLS was trained and tested using retrospective chest radiographs (acquired between 1996 and 2020) from 10 countries. To improve generalization, large-scale chest radiograph pretraining, attention pooling, and semisupervised learning (“noisy-student”) were incorporated. The DLS was evaluated in a four-country test set (China, India, the United States, and Zambia) and in a mining population in South Africa, with positive TB confirmed with microbiological tests or nucleic acid amplification testing (NAAT). The performance of the DLS was compared with that of 14 radiologists. The authors studied the efficacy of the DLS compared with that of nine radiologists using the Obuchowski-Rockette-Hillis procedure. Given WHO targets of 90% sensitivity and 70% specificity, the operating point of the DLS (0.45) was prespecified to favor sensitivity. Results: A total of 165 754 images in 22 284 subjects (mean age, 45 years; 21% female) were used for model development and testing. In the four-country test set (1236 subjects, 17% with active TB), the receiver operating characteristic (ROC) curve of the DLS was higher than those for all nine India-based radiologists, with an area under the ROC curve of 0.89 (95% CI: 0.87, 0.91). Compared with these radiologists, at the prespecified operating point, the DLS sensitivity was higher (88% vs 75%, P < .001) and specificity was noninferior (79% vs 84%, P = .004). Trends were similar within other patient subgroups, in the South Africa data set, and across various TB-specific chest radiograph findings. In simulations, the use of the DLS to identify likely TB-positive chest radiographs for NAAT confirmation reduced the cost by 40%–80% per TB-positive patient detected. Conclusion: A deep learning method was found to be noninferior to radiologists for the determination of active tuberculosis on digital chest radiographs.
View details
Deep learning for distinguishing normal versus abnormal chest radiographs and generalization to two unseen diseases tuberculosis and COVID-19
Shahar Jamshy
Charles Lau
Eddie Santos
Atilla Peter Kiraly
Jie Yang
Rory Pilgrim
Sahar Kazemzadeh
Jin Yu
Lily Hao Yi Peng
Krish Eswaran
Neeral Beladia
Cameron Chen
Shravya Ramesh Shetty
Scientific Reports (2021)
Preview abstract
Chest radiography (CXR) is the most widely-used thoracic clinical imaging modality and is crucial for guiding the management of cardiothoracic conditions. The detection of specific CXR findings has been the main focus of several artificial intelligence (AI) systems. However, the wide range of possible CXR abnormalities makes it impractical to detect every possible condition by building multiple separate systems, each of which detects one or more pre-specified conditions. In this work, we developed and evaluated an AI system to classify CXRs as normal or abnormal. For training and tuning the system, we used a de-identified dataset of 248,445 patients from a multi-city hospital network in India. To assess generalizability, we evaluated our system using 6 international datasets from India, China, and the United States. Of these datasets, 4 focused on diseases that the AI was not trained to detect: 2 datasets with tuberculosis and 2 datasets with coronavirus disease 2019. Our results suggest that the AI system trained using a large dataset containing a diverse array of CXR abnormalities generalizes to new patient populations and unseen diseases. In a simulated workflow where the AI system prioritized abnormal cases, the turnaround time for abnormal cases reduced by 7–28%. These results represent an important step towards evaluating whether AI can be safely used to flag cases in a general setting where previously unseen abnormalities exist. Lastly, to facilitate the continued development of AI models for CXR, we release our collected labels for the publicly available dataset.
View details
Preview abstract
Objective:
Demonstrate the importance of combining multiple readers' opinions, in a context-aware manner, when establishing the reference standard for validation of artificial intelligence (AI) applications for, e.g. chest radiographs. By comparing individual readers, majority vote of a panel, and panel-based discussion, we identify methods which maximize interobserver agreement and label reproducibility.
Methods:
1100 frontal chest radiographs were evaluated for 6 findings: airspace opacity, cardiomegaly, pulmonary edema, fracture, nodules, and pneumothorax. Each image was reviewed by six radiologists, first individually and then via asynchronous adjudication (web-based discussion) in two panels of three readers to resolve disagreements within each panel. We quantified the reproducibility of each method by measuring interreader agreement.
Results:
Panel-based majority vote improved agreement relative to individual readers for all findings. Most disagreements were resolved with two rounds of adjudication, which further improved reproducibility for some findings, particularly reducing misses. Improvements varied across finding categories, with adjudication improving agreement for cardiomegaly, fractures, and pneumothorax.
Conclusion:
The likelihood of interreader agreement, even within panels of US board-certified radiologists, must be considered before reads can be used as a reference standard for validation of proposed AI tools. Agreement and, by extension, reproducibility can be improved by applying majority vote, maximum sensitivity, or asynchronous adjudication for different findings, which supports the development of higher quality clinical research.
View details