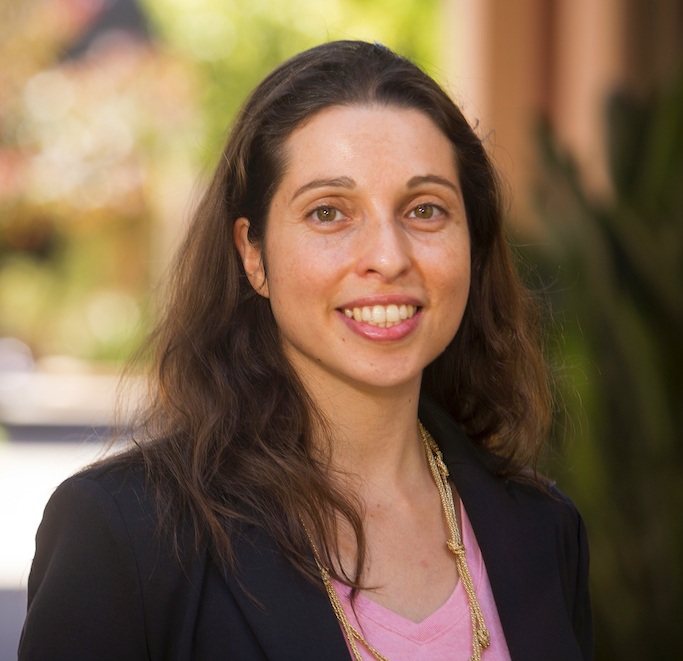
Orly Liba
I'm working in the Creative Camera team, which is a part of GCam, in Machine Perception. We develop computational photography algorithms for the Pixel phones and Google Photos.
I completed my PhD at Stanford in Electrical Engineering. My research was focused on creating new computational and optical tools for seeing under the skin with OCT (optical coherence tomography).
Prior to Stanford, I worked for 4 years in the high-tech industry as an algorithms engineer on computational photography and computer vision.
Research Areas
Authored Publications
Sort By
Google
Zoom-to-Inpaint: Image Inpainting with High Frequency Details
Huiwen Chang
Kfir Aberman
Munchurl Kim
Neal Wadhwa
Nikhil Karnad
Nori Kanazawa
Rahul Garg
Soo Ye Kim
arXiv (2020)
Sky Optimization: Semantically aware image processing in low-light photography
Yun-Ta Tsai
Huizhong Chen
(2020), pp. 526-527
Handheld Mobile Photography in Very Low Light
Kiran Murthy
Yun-Ta Tsai
Tim Brooks
Tianfan Xue
Nikhil Karnad
Dillon Sharlet
Ryan Geiss
Samuel W. Hasinoff
Marc Levoy
ACM Transactions on Graphics, 38 (2019), pp. 16