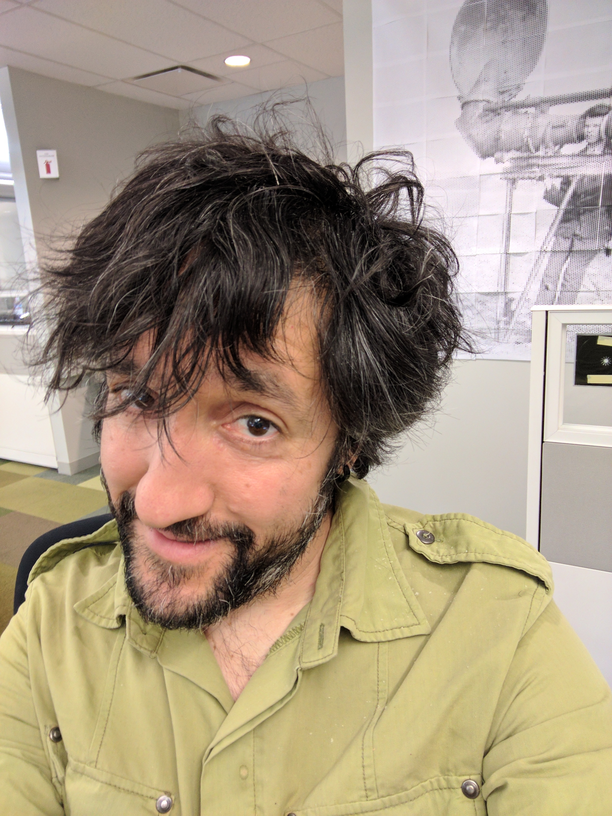
Rif A. Saurous
Authored Publications
Sort By
Sequential Monte Carlo Learning for Time Series Structure Discovery
Matthew D. Hoffman
Vikash Mansinghka
Feras Saad
Proceedings of the 40th International Conference on Machine Learning (2023), pp. 29473-29489
Preview abstract
This paper presents a new approach to automatically discovering accurate
models of complex time series data. Working within a Bayesian nonparametric
prior over a symbolic space of Gaussian process time series models, we
present a novel structure learning algorithm that integrates sequential
Monte Carlo (SMC) and involutive MCMC for highly effective posterior
inference. Our method can be used both in "online'' settings, where new
data is incorporated sequentially in time, and in "offline'' settings, by
using nested subsets of historical data to anneal the posterior. Empirical
measurements on a variety of real-world time series show that our method
can deliver 10x--100x runtime speedups over previous MCMC and greedy-search
structure learning algorithms for the same model family. We use our method
to perform the first large-scale evaluation of Gaussian process time series
structure learning on a widely used benchmark of 1,428 monthly econometric
datasets, showing that our method discovers sensible models that deliver
more accurate point forecasts and interval forecasts over multiple horizons
as compared to prominent statistical and neural baselines that struggle on
this challenging data.
View details
Coincidence, Categorization, and Consolidation: Learning to Recognize Sounds with Minimal Supervision
Proceedings of ICASSP 2020 (2020) (to appear)
Preview abstract
Humans do not acquire perceptual abilities like we train machines. While machine learning algorithms typically operate on large collections of randomly-chosen, explicitly-labeled examples, human acquisition relies far greater on multimodal unsupervised learning (as infants) and active learning (as children). With this motivation, we present a learning framework for sound representation and recognition that combines (i) a self-supervised objective based on a general notion of unimodal and cross-modal coincidence, (ii) a novel clustering objective that reflects our need to impose categorical structure on our experiences, and (iii) a cluster-based active learning procedure that solicits targeted weak supervision to consolidate hypothesized categories into relevant semantic classes. By jointly training a single sound embedding/clustering/classification network according to these criteria, we achieve a new state-of-the-art unsupervised audio representation and demonstrate
up to 20-fold reduction in labels required to reach a desired classification performance.
View details
Estimating the Changing Infection Rate of COVID-19 Using Bayesian Models of Mobility
Matthew D. Hoffman
Shawn O'Banion
Xue Ben
medRxiv, https://www.medrxiv.org/content/10.1101/2020.08.06.20169664v1.full (2020)
Preview abstract
In order to prepare for and control the continued spread of the COVID-19 pandemic while minimizing its economic impact, the world needs to be able to estimate and predict COVID-19’s spread.
Unfortunately, we cannot directly observe the prevalence or growth rate of COVID-19; these must be inferred using some kind of model.
We propose a hierarchical Bayesian extension to the classic susceptible-exposed-infected-removed (SEIR) compartmental model that adds compartments to account for isolation and death and allows the infection rate to vary as a function of both mobility data collected from mobile phones and a latent time-varying factor that accounts for changes in behavior not captured by mobility data. Since confirmed-case data is unreliable, we infer the model’s parameters conditioned on deaths data. We replace the exponential-waiting-time assumption of classic compartmental models with Erlang distributions, which allows for a more realistic model of the long lag between exposure and death. The mobility data gives us a leading indicator that can quickly detect changes in the pandemic’s local growth rate and forecast changes in death rates weeks ahead of time. This is an analysis of observational data, so any causal interpretations of the model's inferences should be treated as suggestive at best; nonetheless, the model’s inferred relationship between different kinds of trips and the infection rate do suggest some possible hypotheses about what kinds of activities might contribute most to COVID-19’s spread.
View details
Large-Scale Weakly-Supervised Content Embeddingsfor Music Recommendation and Tagging
Li Zhang
John Roberts Anderson
Qingqing Huang
ICASSP 2020 (2020)
Preview abstract
We explore content-based representation learning strategies tailored for
large-scale, uncurated music collections that afford only weak supervision
through unstructured natural language metadata and co-listen statistics. At the
core is a hybrid training scheme that uses classification and metric learning
losses to incorporate both metadata-derived text labels and aggregate co-listen
supervisory signals into a single convolutional model. The resulting joint text
and audio content embedding defines a similarity metric and supports prediction
of semantic text labels using a vocabulary of unprecedented granularity, which
we refine using a novel word-sense disambiguation procedure. As input to simple
classifier architectures, our representation achieves state-of-the-art
performance on two music tagging benchmarks.
View details
Automatically batching control-intensive programs for modern accelerators
Matthew D. Hoffman
Dougal Maclaurin
Alexey Radul
Third Conference on Systems and Machine Learning, Austin, TX (2020)
Preview abstract
We present a general approach to batching arbitrary computations for
GPU and TPU accelerators. We demonstrate the effectiveness of our
method with orders-of-magnitude speedups on the No U-Turn Sampler
(NUTS), a workhorse algorithm in Bayesian statistics. The central
challenge of batching NUTS and other Markov chain Monte Carlo
algorithms is data-dependent control flow and recursion. We overcome
this by mechanically transforming a single-example implementation into
a form that explicitly tracks the current program point for each batch
member, and only steps forward those in the same place. We present
two different batching algorithms: a simpler, previously published one
that inherits recursion from the host Python, and a more complex,
novel one that implmenents recursion directly and can batch across it.
We implement these batching methods as a general program
transformation on Python source. Both the batching system and the
NUTS implementation presented here are available as part of the
popular TensorFlow Probability software package.
View details
Differentiable Consistency Constraints for Improved Deep Speech Enhancement
Michael Chinen
Jeremy Thorpe
IEEE International Conference on Acoustics, Speech, and Signal Processing (2019)
Preview abstract
In recent years, deep networks have led to dramatic improvements in speech enhancement by framing it as a data-driven pattern recognition problem. In many modern enhancement systems, large amounts of data are used to train a deep network to estimate masks for complex-valued short-time Fourier transforms (STFTs) to suppress noise and preserve speech. However, current masking approaches often neglect two important constraints: STFT consistency and mixture consistency. Without STFT consistency, the system’s output is not necessarily the STFT of a time-domain signal, and without mixture consistency, the sum of the estimated sources does not necessarily equal the input mixture. Furthermore, the only previous approaches that apply mixture consistency use real-valued masks; mixture consistency has been ignored for complex-valued masks. In this paper, we show that STFT consistency and mixture consistency can be jointly imposed by adding simple differentiable projection layers to the enhancement network. These layers are compatible with real or complex-valued masks. Using both of these constraints with complex-valued masks provides a 0.7 dB increase in scale-invariant signal-to-distortion ratio (SI-SDR) on a large dataset of speech corrupted by a wide variety of nonstationary noise across a range of input SNRs.
View details
Natural TTS Synthesis By Conditioning WaveNet On Mel Spectrogram Predictions
Ruoming Pang
Yu Zhang
Yuxuan Wang
Yonghui Wu
Yannis Agiomyrgiannakis
Mike Schuster
Jonathan Shen
Navdeep Jaitly
Zongheng Yang
ICASSP (2018)
Preview abstract
This paper describes Tacotron 2, a neural network architecture for speech synthesis directly from text. The system is composed of a recurrent sequence-to-sequence feature prediction network that maps character embeddings to mel-scale spectrograms, followed by a modified WaveNet model acting as a vocoder to synthesize timedomain waveforms from those spectrograms. Our model achieves a mean opinion score (MOS) of 4.53 comparable to a MOS of 4.58 for professionally recorded speech.
To validate our design choices, we present ablation studies of key components of our system and evaluate the impact of using mel spectrograms as the input to WaveNet instead of linguistic, duration, and F0 features. We further demonstrate that using a compact acoustic intermediate representation enables significant simplification of the WaveNet architecture.
View details
VoiceFilter: Targeted Voice Separation by Speaker-Conditioned Spectrogram Masking
Ye Jia
Prashant Sridhar
ICASSP 2019 (2018)
Preview abstract
In this paper, we present a novel system that separates the voice of a target speaker from multi-speaker signals, by making use of a reference signal from the target speaker. We achieve this by training two separate neural networks: (1) A speaker recognition network that produces speaker-discriminative embeddings; (2) A spectrogram masking network that takes both noisy spectrogram and speaker embedding as input, and produces a mask. Our system significantly reduces the speech recognition WER on multi-speaker signals, with minimal WER degradation on single-speaker signals.
View details
EXPLORING TRADEOFFS IN MODELS FOR LOW-LATENCY SPEECH ENHANCEMENT
Michael Chinen
Jeremy Thorpe
Proceedings of the 16th International Workshop on Acoustic Signal Enhancement (2018)
Preview abstract
We explore a variety of configurations of neural networks for one- and
two-channel spectrogram-mask-based speech enhancement. Our best model improves on
state-of-the-art performance on the CHiME2 speech enhancement task.
We examine trade-offs among non-causal lookahead, compute work, and parameter count versus enhancement performance and find that zero-lookahead models can achieve, on average, only 0.5 dB worse performance than our best bidirectional model. Further, we find that 200 milliseconds of lookahead is sufficient to achieve performance within about 0.2 dB from our best bidirectional model.
View details
Neumann Optimizer: A Practical Optimizer for Deep Neural Networks
Ying Xiao
Shankar Krishnan
International Conference on Learning Representations (ICLR) (2018)
Preview abstract
Progress in deep learning is slowed by the days or weeks it takes to train large models. The natural solution of using more hardware is limited by diminishing returns, and leads to inefficient use of additional resources. In this paper, we present a large batch, stochastic optimization algorithm that is both faster than widely used algorithms for fixed amounts of computation, and is also able to scale up substantially better as more computational resources become available. Our algorithm implicitly computes the inverse hessian of each mini-batch to produce descent directions. We demonstrate the effectiveness of our algorithm by successfully training large ImageNet models (Inception V3, Resnet-50, Resnet-101 and Inception-Resnet) with mini-batch sizes of up to 32000 with no loss in validation error relative to current baselines, and no increase in the total number of steps. At smaller mini-batch sizes, our optimizer improves the validation error in these models by 0.8-0.9%. Alternatively, we can trade off this accuracy to reduce the number of training steps needed by roughly 10-30%. Our work is practical and easily usable by others -- only one hyperparameter (learning rate) needs tuning, and furthermore, the algorithm is as computationally cheap as the commonly used adam optimizer.
View details