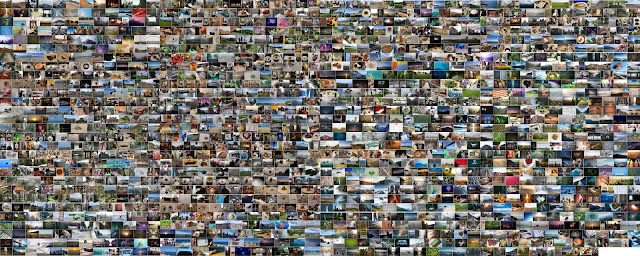
Introducing the CVPR 2018 Learned Image Compression Challenge
January 10, 2018
Posted by Michele Covell, Research Scientist, Google Research
Quick links
Edit 17/01/2018: Due to popular request, the CLIC competition submission deadline has been extended to April 22. Please see compression.cc for more details.
Image compression is critical to digital photography — without it, a 12 megapixel image would take 36 megabytes of storage, making most websites prohibitively large. While the signal-processing community has significantly improved image compression beyond JPEG (which was introduced in the 1980’s) with modern image codecs (e.g., BPG, WebP), many of the techniques used in these modern codecs still use the same family of pixel transforms as are used in JPEG. Multiple recent Google projects improve the field of image compression with end-to-end with machine learning, compression through superresolution and creating perceptually improved JPEG images, but we believe that even greater improvements to image compression can be obtained by bringing this research challenge to the attention of the larger machine learning community.
To encourage progress in this field, Google, in collaboration with ETH and Twitter, is sponsoring the Workshop and Challenge on Learned Image Compression (CLIC) at the upcoming 2018 Computer Vision and Pattern Recognition conference (CVPR 2018). The workshop will bring together established contributors to traditional image compression with early contributors to the emerging field of learning-based image compression systems. Our invited speakers include image and video compression experts Jim Bankoski (Google) and Jens Ohm (RWTH Aachen University), as well as computer vision and machine learning experts with experience in video and image compression, Oren Rippel (WaveOne) and Ramin Zabih (Google, on leave from Cornell).
![]() |
Training set of 1,633 uncompressed images from both the Mobile and Professional datasets, available on compression.cc |
The first large-image compression systems using neural networks were published in 2016 [Toderici2016, Ballé2016] and were only just matching JPEG performance. More recent systems have made rapid advances, to the point that they match or exceed the performance of modern industry-standard image compression [Ballé2017, Theis2017, Agustsson2017, Santurkar2017, Rippel2017]. This rapid advance in the quality of neural-network-based compression systems, based on the work of a comparatively small number of research labs, leads us to expect even more impressive results when the area is explored by a larger portion of the machine-learning community.
We hope to get your help advancing the state-of-the-art in this important application area, and we encourage you to participate if you are planning to attend CVPR this year! Please see compression.cc for more details about the new datasets and important workshop deadlines. Training data is already available on that site. The test set will be released on February 15 and the deadline for submitting the compressed versions of the test set is February 22. Edit 17/01/2018: Due to popular request, the CLIC competition submission deadline has been extended to April 22. Please see compression.cc for more details.
-
Labels:
- Machine Perception