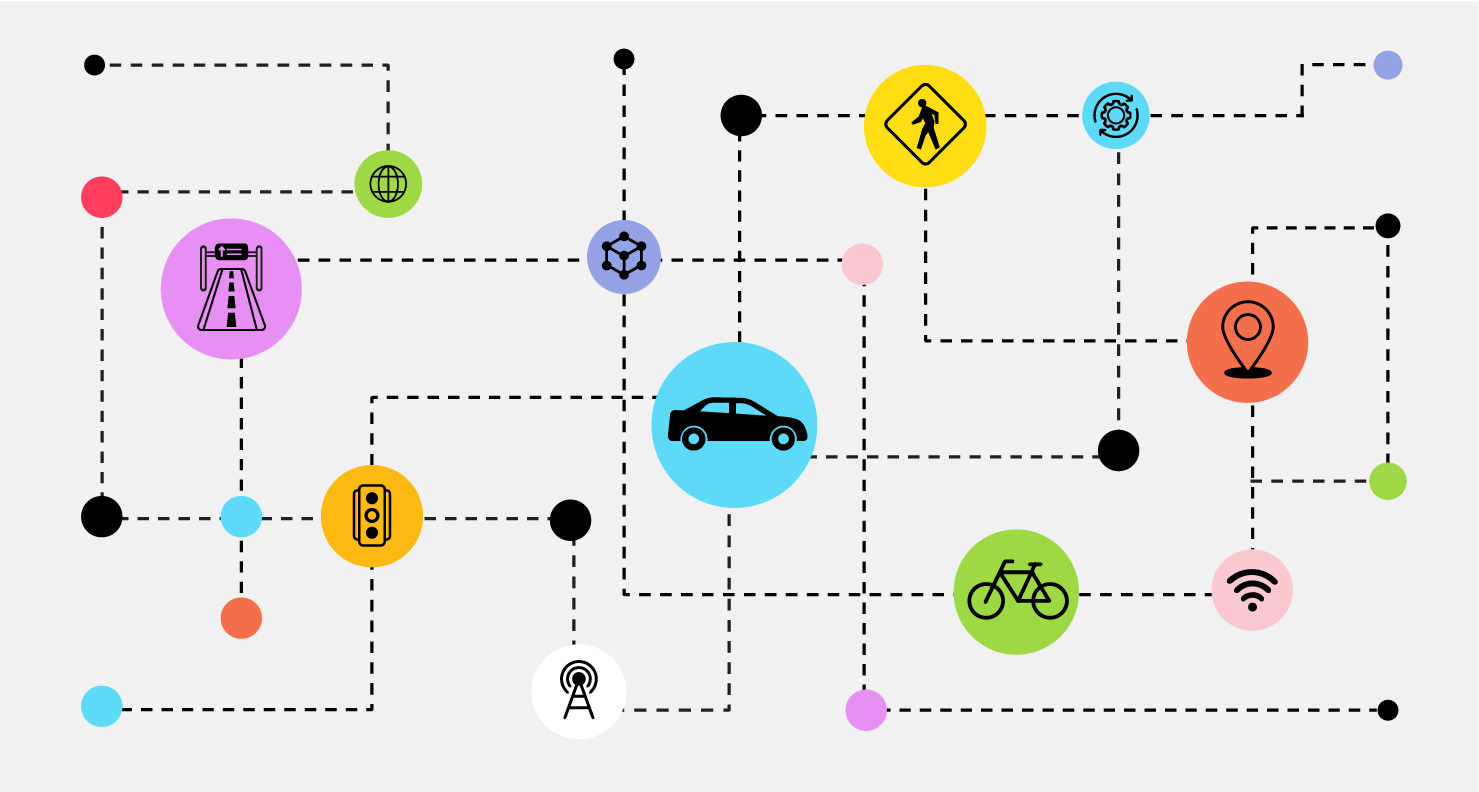
Introducing Mobility AI: Advancing urban transportation
April 23, 2025
Neha Arora, Software Engineer, and Ivan Kuznetsov, Group Product Manager, Google Research
Google Research introduces Mobility AI, a program leveraging AI advancements in measurement, simulation, and optimization to provide transportation agencies with powerful tools for data-driven policymaking, traffic management, and continuous monitoring of urban transportation systems.
Quick links
Traffic gridlock, environmental concerns, and unacceptable road fatalities (38,585 U.S. traffic deaths in 2023) are among the increasingly complex transportation challenges being faced by rapidly growing cities. These issues are compounded by evolving trends: ride-sharing services alter parking demand while increasing the need for efficient traffic management; the rise of e-commerce reshapes urban logistics, creating new mobility patterns that call for innovative curb space and road capacity solutions; and the prospect of autonomous vehicles presents both challenges in infrastructure and regulation and opportunities for safer, more efficient transportation. Concurrently, the evolution and maturation of AI technologies present powerful new capabilities that enable us to tackle these complex issues at scale, offering new ways to manage congestion, enhance public and multimodal transportation, support freight movement, and build community resiliency.
In response to these dynamic shifts, Google Research is introducing Mobility AI, a program focused on driving scientific breakthroughs in mobility. This program translates cutting-edge research into core technologies that underpin solutions available via Google Public Sector and Google Maps Platform, empowering transportation leaders to understand and improve urban transportation. Our goal is to equip transportation agencies, planners, engineers, researchers, and policymakers with the capabilities needed for data-driven policy making, effective traffic management, and continuous monitoring of transportation systems — from roads and transit to cycling and pedestrian infrastructure.
In this blog, we’ll explain the foundational research driving Mobility AI and explore the key technological advances from Google within the core pillars fundamental to this area of research: measurement, simulation, and optimization. This research aims to enable deeper understanding and replicating of the transportation network and mobility patterns, and better design and evaluation of interventions.
A foundation of research and innovation
For over two decades, Google Research has been a leader in transportation innovation, developing advanced mapping and routing technologies and traffic prediction algorithms that help people navigate cities more efficiently. We’ve invested heavily in understanding traffic formation, developing simulations to design interventions, and evaluating traffic management strategies. The availability of high-resolution, global-scale mobility data, paired with advances in transportation science and simulation calibration, paves the way for scalable solutions.
Building on this expertise, Mobility AI represents a focused effort to structure and integrate Google's research in this domain. We are making the next generation of AI-driven transportation technologies available by building foundational capabilities that provide the scientific and technological backbone needed by public agencies and their partners to tackle urban transportation challenges with precision and impact.
The Mobility AI framework: Measurement, simulation, optimization
Our research is organized into three pillars:
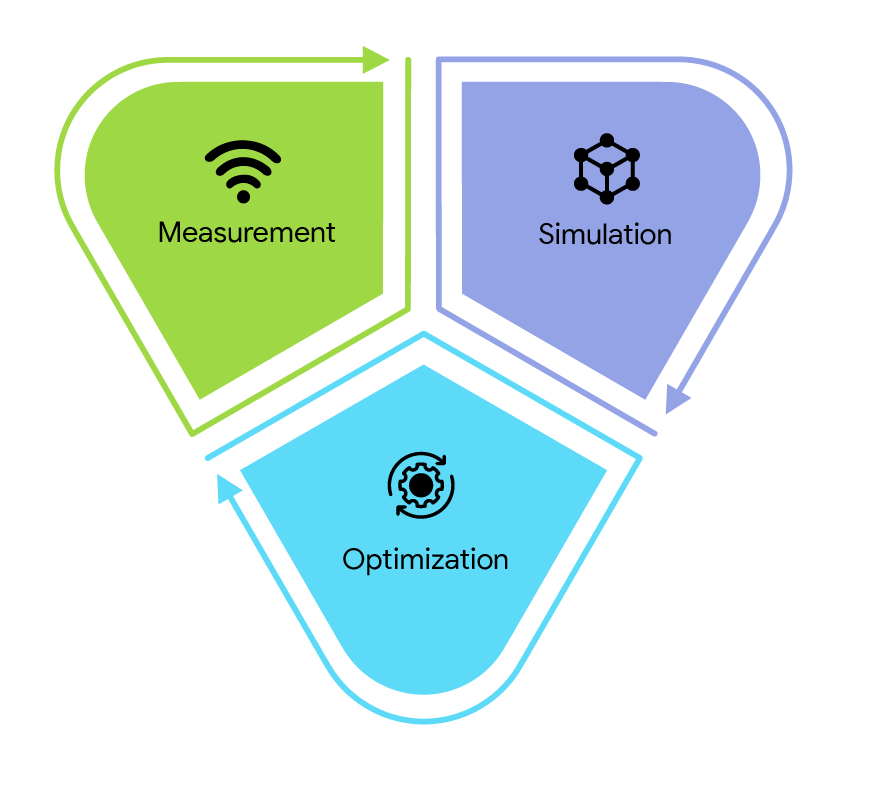
The Mobility AI framework.
1. Measurement: Understanding mobility patterns
Accurately evaluating the current state of the transportation network and mobility patterns is the first step to improving mobility. This involves gathering and analyzing real-time and historical data from various sources to understand both current and historical conditions and trends. We need to track the effects of changes as we implement them in the network. ML powers estimations and metric computations, while statistical approaches measure impact. Key areas include:
Congestion functions
Similar to well-known fundamental diagrams of traffic flow, congestion functions mathematically describe how rising vehicle volume increases congestion and reduces travel speeds, providing crucial insights into traffic behavior. Unlike fundamental diagrams, congestion functions are built based on a portion of vehicles (e.g., floating car data) rather than all traveling vehicles. We have advanced the understanding of congestion formation and propagation using an ML approach that created city-wide models, which enable robust inference on roads with limited data and, through analytical formulation, reveal how traffic signal adjustments influence flow distribution and congestion patterns in urban areas.
Foundational geospatial understanding
We develop novel frameworks, leveraging techniques like self-supervised learning on geospatial data and movement patterns, to learn embeddings that capture both local characteristics and broader spatial relationships. These representations improve the understanding of mobility patterns and can aid downstream tasks, especially where data might be sparse or when complementing other data modalities. Collaboration with related Google Research efforts in Geospatial Reasoning using generative AI and foundation models is crucial for advancing these capabilities.
Parking insights
Understanding urban intricacies includes parking. Building on our work using ML to predict parking difficulty, Mobility AI aims to provide better insights for managing parking availability, crucial for various people, including commuters, ride-sharing drivers, commercial delivery vehicles, and the emerging needs of self-driving vehicles.
Origin–destination travel demand estimation
Origin–destination (OD) travel demand, which describes where trips — like daily commutes, goods deliveries, or shopping journeys — start and end, is fundamental to understanding and optimizing mobility. Knowing these patterns is crucial because it reveals exactly where the transportation network is stressed and where services or infrastructure improvements are most needed. We calibrate OD matrices — tables quantifying these trips between locations — to accurately replicate observed traffic patterns, providing a spatially complete understanding essential for planning and optimization of transportation networks.
Performance metrics: Safety, emissions and congestion impact
We use aggregated and anonymized Google Maps traffic trends to assess impact of transportation interventions on congestion, and we build models to assess safety and emissions impact. To build safety metrics scalably, we go beyond reactive crash data by utilizing hard braking events (HBEs). HBEs are shown to be strongly correlated with crashes and can be used for road safety services to pinpoint high-risk locations and predict future collision risks.
To measure environmental impact, we've developed AI models in partnership with the National Renewable Energy Laboratory (NREL) that predict vehicle energy consumption (whether gas, diesel, hybrid, or electric). This powers fuel-efficient routing in Google Maps, estimated to have helped avoid 2.9M metric tons of GHG emissions in the US alone, which is equivalent to taking ~650,000 cars off the road for a year. This capability is fundamental for monitoring climate and health impacts related to transportation choices.
Impact evaluation
Randomized trials are often infeasible for evaluating transportation policy changes. To assess the impact of a change, we need to estimate outcomes in its absence. This can be done by finding cities or regions with similar mobility patterns to serve as a “control group”. Our analysis of NYC's congestion pricing demonstrates this method through use of sophisticated statistical techniques like synthetic controls to rigorously estimate the policy's impact and by providing valuable insights for agencies evaluating interventions.
2. Simulation
For the simulation pillar, we build high-resolution digital twins — virtual replicas — of transportation systems. These allow planners and engineers to test interventions, forecast outcomes, and evaluate "what-if" scenarios (from minor signal timing changes to major infrastructure projects or emergency response plans) before implementing them in the real world.
Our goal is to extend the reach of traffic simulations, traditionally confined to localized areas like single highways or arterials, to encompass entire metropolitan networks with thousands of road segments. We also aim for rapid deployment across new cities and scenarios. Accomplishing this was challenging in the past as adjusting simulation parameters to match the specific dynamics of a particular setting, also known as calibration, was done using sparsely available traffic sensor data. This dependency limited the ability to observe and reproduce a wide range of congestion patterns. Our research has overcome this limitation by developing methods to calibrate simulations by incorporating more comprehensive data, like aggregated and anonymized speeds, counts, and path travel times, into physics-informed traffic models. Furthermore, recognizing the computational demands of detailed simulation calibration, we are actively working to improve efficiency alongside these advances in scaling and accuracy.
Since digital twins are used to carry out what-if analysis to estimate the impact of potential future interventions on the mobility network, it is important to understand how uncertainty in the simulation inputs (e.g., demand, supply) impacts the simulation outputs. We develop algorithms that quantify this input uncertainty. This enables cities to use the digital twins to carry out robust what-if analysis.
3. Optimization
Leveraging insights from measurement and simulation, we apply advanced algorithms and AI to identify the most effective solutions for transportation networks. This includes:
- Intervention design: Creating data-driven plans for specific challenges. For example, we collaborated with Seattle DOT on post-event traffic guidance and developed detailed evacuation plans for the city of Mill Valley, identifying network modifications to increase effectiveness during emergency response scenarios. This also has applications in optimizing traffic flow around work zones or managing freight movement.
- Routing strategies: Developing routing approaches that improve overall network efficiency and reduce social costs.
- Traffic signal control: Optimizing signal timing to reduce delays and emissions (like Project Green Light).
Shaping the future of Urban Mobility, together
Mobility AI represents Google Research's ongoing commitment to applying scientific advancements, including foundational machine learning and algorithms, to help build more efficient, safe, and resilient transportation systems for functional urban areas. By focusing on robust measurement, predictive simulation, and effective optimization, we aim to provide the technology needed to manage congestion, improve public transportation access, and enhance community well-being through better routing, mapping and modeling.
We believe collaboration is key to advancing Mobility AI. We are eager to work with transportation agencies, planners, researchers, and mobility providers to translate the research insights from Mobility AI into practical applications, leveraging digital infrastructure and enabling better data sharing for maximum impact.
Interested in learning more about these research advances and discussing the potential for your organization? Please fill out our interest form to connect with our team.