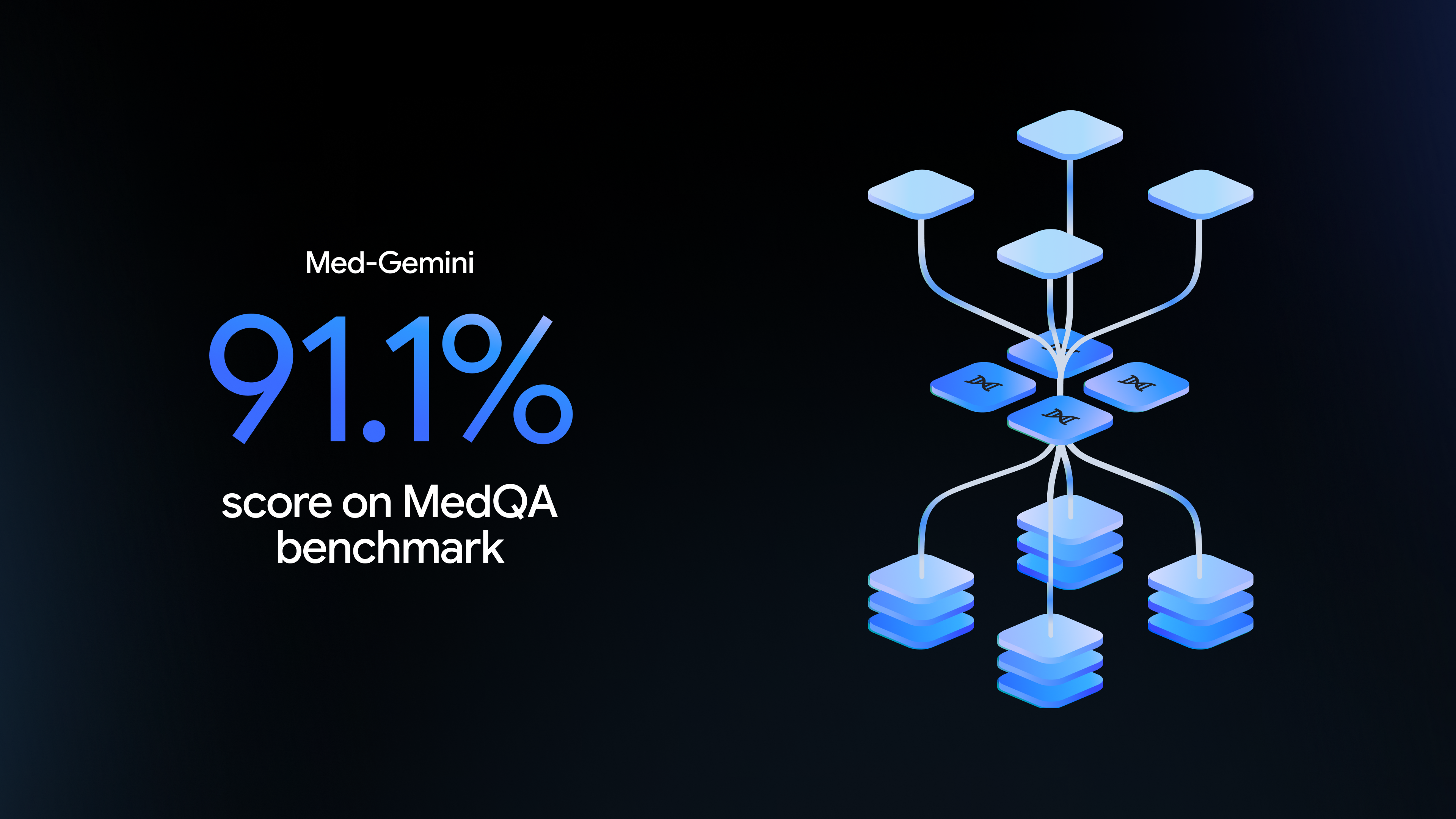
Advancing medical AI with Med-Gemini
May 15, 2024
Greg Corrado, Distinguished Scientist, Google Research, and Joëlle Barral, Senior Director, Google DeepMind
For AI models to perform well on diverse medical tasks and to meaningfully assist in clinician, researcher and patient workflows (like generating radiology reports or summarizing health information), they often require advanced reasoning and the ability to utilize specialized, up-to-date medical knowledge. In addition, strong performance requires models to move beyond short passages of text to understand complex multimodal data, including images, videos, and the extensive length and breadth of electronic health records (EHRs). With this in mind, Gemini models have demonstrated a leap forward in multimodal and long-context reasoning, which presents substantial potential in medicine.
Today we present two recent research papers in which we explore the possibilities of Gemini in the healthcare space and introduce Med-Gemini, a new family of next-generation models fine-tuned for the medical domain. This family of models builds upon Google’s Gemini models by fine-tuning on de-identified medical data while inheriting Gemini’s native reasoning, multimodal, and long-context abilities. Med-Gemini builds on our initial research into medically tuned large language models with Med-PaLM.
The first paper, “Capabilities of Gemini Models in Medicine”, describes a broad exploration of Gemini’s capabilities across a wide range of text, image, video, and EHR tasks. We benchmark the new Med-Gemini models on 14 tasks spanning text, multimodal and long-context applications, and demonstrate strong results, including a new state-of-the-art of 91.1% accuracy for the popular MedQA benchmark.
In the second paper, “Advancing Multimodal Medical Capabilities of Gemini”, we offer a deeper dive into Med-Gemini’s multimodal capabilities through application to radiology, pathology, dermatology, ophthalmology, and genomics in healthcare. We focus on clinical applicability, improving benchmarks, and leveraging specialist evaluations to assess the models’ capabilities. For the first time, we demonstrate how large multimodal models can interpret complex 3D scans, answer clinical questions, and generate state-of-the-art radiology reports. Additionally, we demonstrate a novel mechanism to encode genomic information for risk prediction using large language models across a wealth of disease areas with strong results.
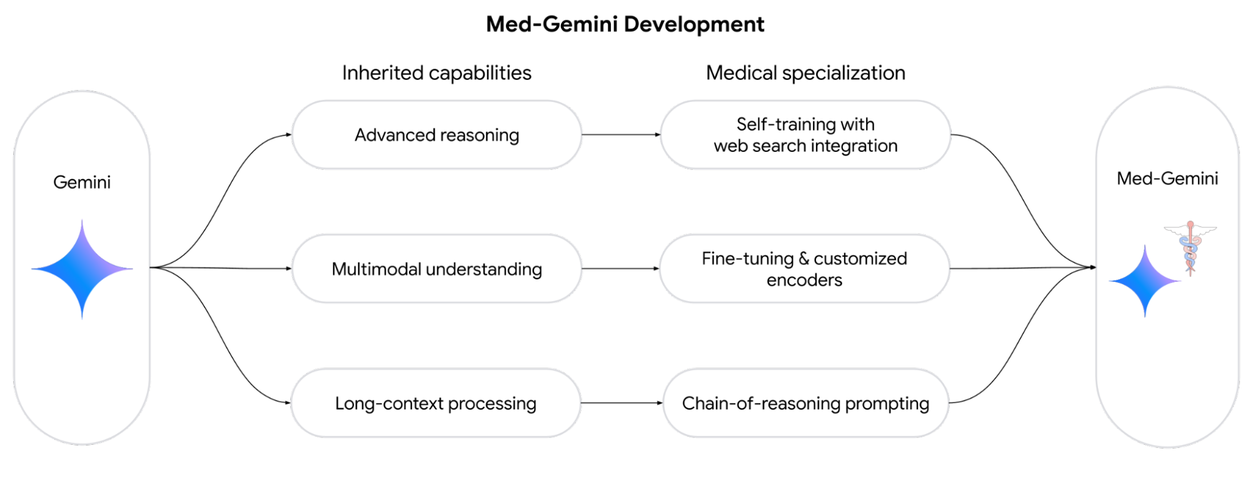
We introduce Med-Gemini, a family of highly capable, multimodal medical models built upon Gemini. We enhance our models' clinical reasoning through self-training and web search integration, improve multimodal performance via fine-tuning and customized encoders, and better utilize long-context capabilities with chain-of-reasoning prompting.
The broad capabilities of Gemini models in medicine
In “Capabilities of Gemini Models in Medicine”, we enhance our models’ clinical reasoning capabilities through self-training and web search integration, while improving multimodal performance through fine-tuning and customized encoders.
We then benchmark Med-Gemini models on 14 tasks spanning text, multimodal and long-context applications. In the popular MedQA US Medical Licensing Exam (USMLE)-style question benchmark, Med-Gemini achieves a state-of-the-art performance of 91.1% accuracy, surpassing our prior best of Med-PaLM 2 by 4.6% (shown below). We conducted a careful inspection of the MedQA benchmark with expert clinicians, and found that 7.4% of the questions were deemed unfit for evaluation as they either lacked key information or supported multiple plausible interpretations. We account for these data quality issues to more precisely characterize the performance of our model.
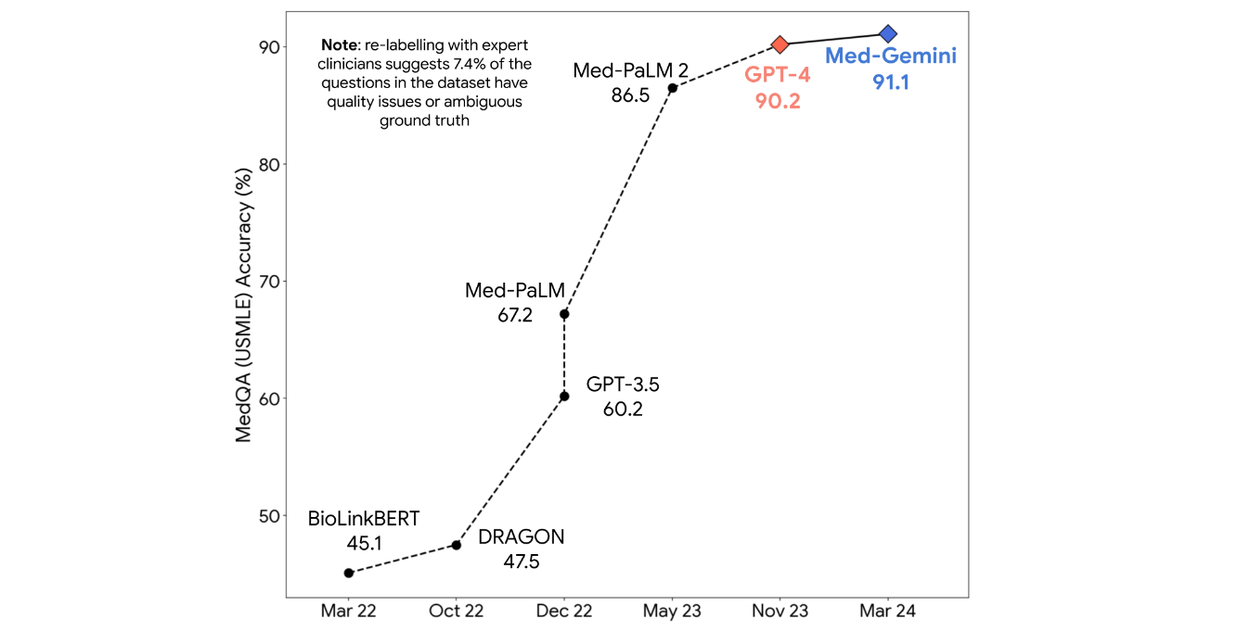
On the MedQA (USMLE-style) benchmark, Med-Gemini attains a new state-of-the-art score, surpassing our prior best (Med-PaLM 2) by a significant margin of 4.6%.
Our approach utilizes uncertainty-guided web search to enable the model to use accurate and up-to-date information. This approach generalizes to achieve state-of-the-art performance in other challenging benchmarks, including complex diagnostic challenges from NEJM clinico-pathological conferences.
On multimodal benchmarks such as NEJM Image Challenges and multimodal USMLE-style questions, Med-Gemini achieves a new state of the art, surpassing GPT-4 V by a wide margin. We demonstrate the effectiveness of long-context capabilities through medical video and EHR question answering on which Med-Gemini surpasses prior bespoke methods in a zero-shot manner. In order to assess performance on common text-based tasks, we evaluated performance on medical text summarization and simplification of referral letter generation, finding that Med-Gemini–produced drafts were preferred to those of clinicians for succinctness, coherence, and in some cases also for accuracy. In multiple qualitative examples, we demonstrate the clear potential for Med-Gemini to provide accurate and useful conversation about multimodal artifacts such as medical imaging (dermatology or radiology) or long multimodal research articles.
In this safety-critical domain, considerable further research is needed before real-world application can be considered. For example, additional research and evaluations on potential biases, safety, and reliability are essential to ensure building safe applications. These systems should also be thoroughly evaluated not just in isolation, but with human experts in the loop.
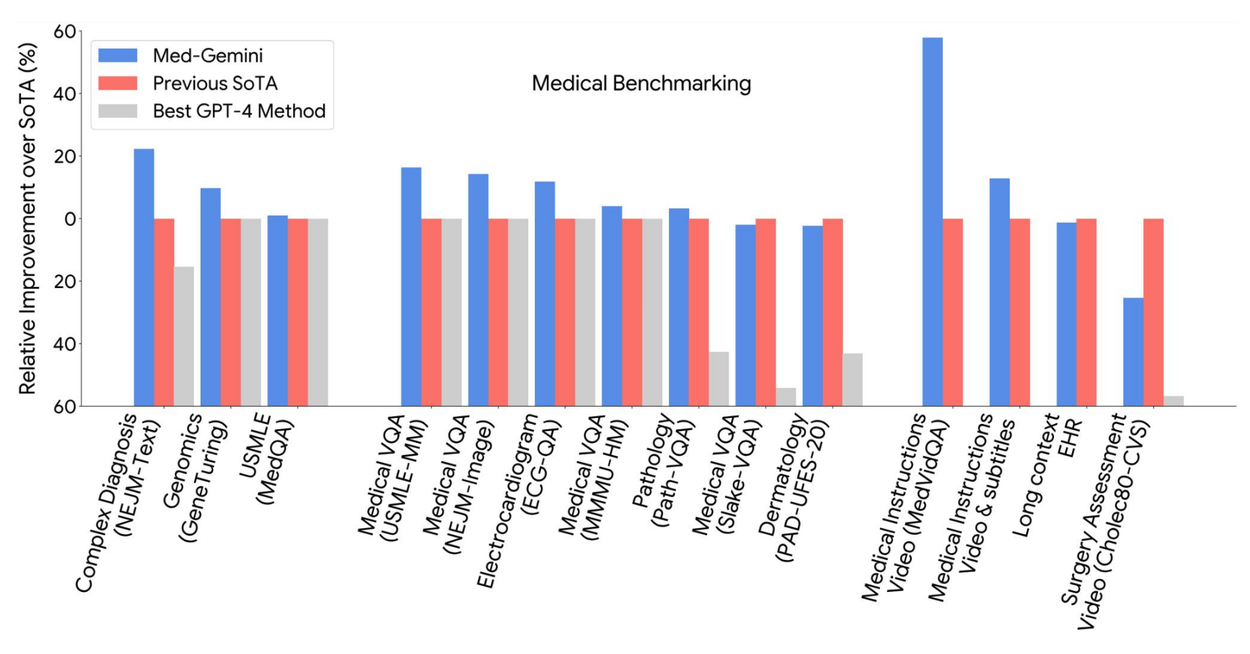
Med-Gemini models achieve state-of-the-art performance on 10 out of 14 medical benchmarks that span text, multimodal, and long-context applications, and surpass the GPT-4 model family on every benchmark where a direct comparison could be made.
Advancing multimodal medical capabilities
Our second piece of research, “Advancing Multimodal Medical Capabilities of Gemini”, expands on our multimodal medical large language model work from 2023 by diving deeper into multimodal capabilities made possible by the Gemini family of models. We focus on tuning for radiology, pathology, dermatology, ophthalmology, and genomics applications in healthcare via Med-Gemini-2D, Med-Gemini-3D, and Med-Gemini-Polygenic.
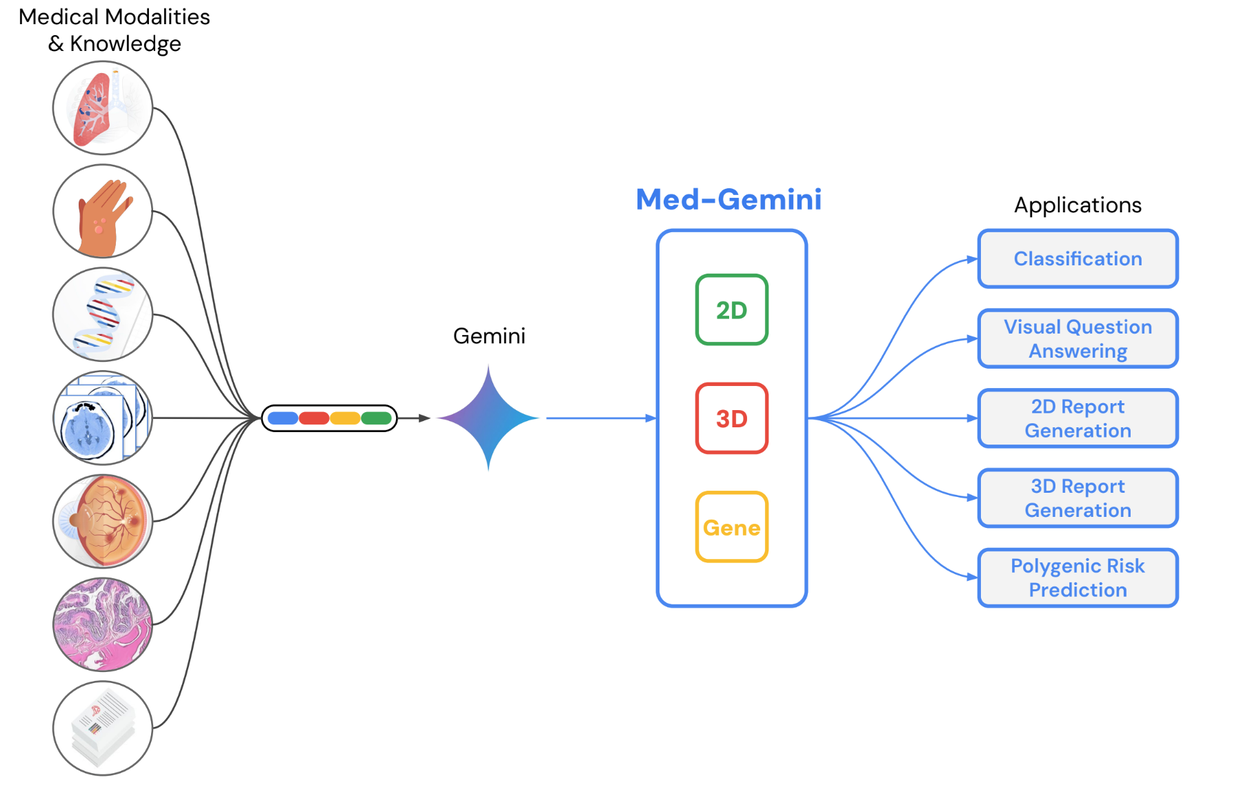
Trained on a range of conventional 2D medical images (chest X-rays, CT slices, pathology slides, etc) using de-identified medical data with free text labels, Med-Gemini-2D is able to perform a number of tasks, such as classification, visual question answering, and text generation. One example includes report generation for chest X-rays, which was shown to exceed previous state-of-the-art results by up to 12% across normal and abnormal scans from two separate datasets.
Med-Gemini-3D can also understand and write radiology reports for 3D studies like computed tomography (CT) imaging of the head (example shown below). Volumetric, 3D scans are an essential tool in modern medicine, providing more context for countless diagnostic and treatment decisions requiring an understanding of 3D anatomy. Technically, 3D reflects a meaningful jump in multimodal clinical task complexity when compared to 2D. Additionally, in evaluation of the reports generated by Med-Gemini-3D, more than half were determined to result in the same care recommendations made by a radiologist. However, additional real-world research and validation is needed to ensure consistent expert reporting quality.
Beyond report generation, Med-Gemini-2D also achieves a new state-of-the-art for chest X-ray visual question answering, and performs well across a range of classification tasks across histopathology, ophthalmology, dermatology, and radiology, approaching the performance of task-specific models.
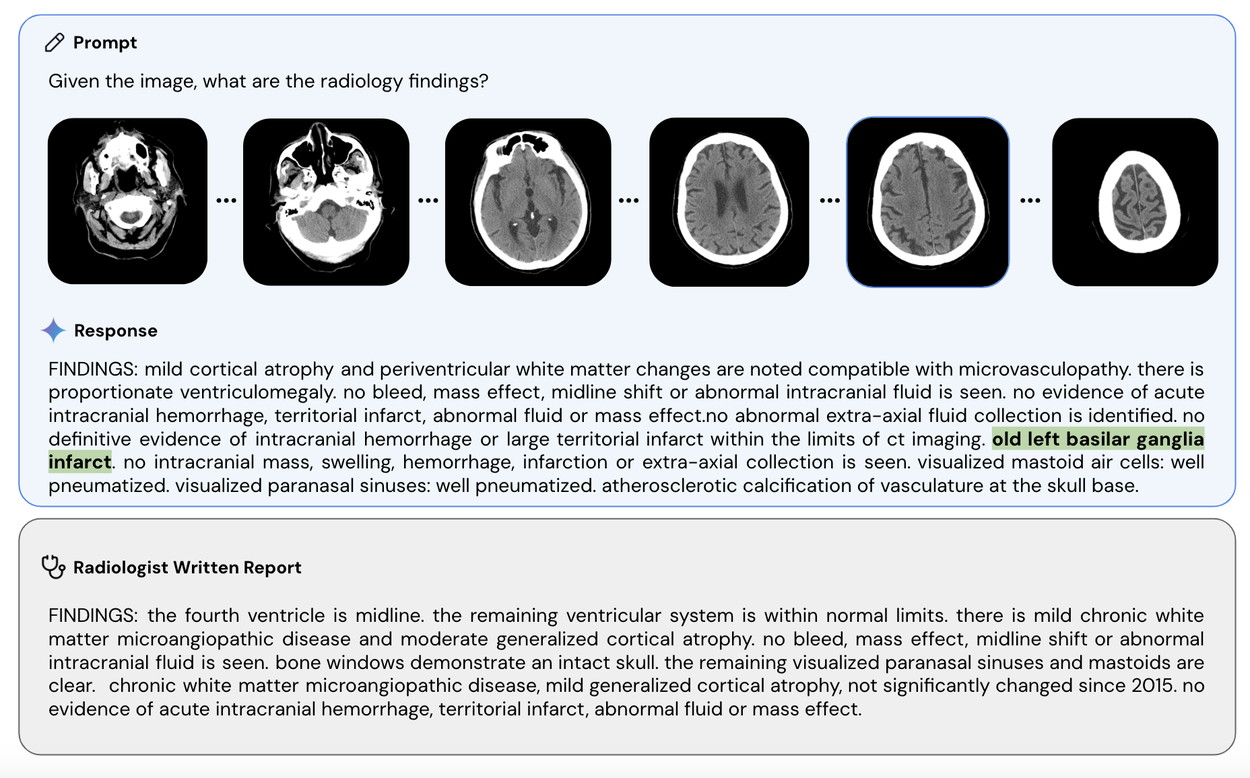
Med-Gemini-3D is able to generate reports for CT scans, a far more complex form of imaging than standard X-rays. In this example, Med-Gemini-3D’s report has correctly included a pathology (highlighted in green) that was missed in the original radiologist’s report. Note that 'basilar' is a common mis-transcription of 'basal' that Med-Gemini has learned from the training data, though the meaning of the report is unchanged.
Beyond imaging, our new Med-Gemini-Polygenic research model is the first language model to perform disease and health outcome prediction from genomic data. The model outperforms previous linear polygenic scores for prediction of eight health outcomes (depression, stroke, glaucoma, rheumatoid arthritis, all cause mortality, coronary artery disease, chronic obstructive pulmonary disease (COPD), type 2 diabetes) and is surprisingly able to predict an additional six health outcomes for which it had not explicitly been trained. Based on this performance, we believe the model is able to leverage intrinsic knowledge about genetic correlation for predicting health outcomes.
Evaluation is an increasingly critical piece of research in this domain. In this work the team explores both benchmarks and other objective measures for classification and closed-ended questions (e.g., yes/no or limited choice formats) as well as specialist panel-based assessments for text generation and open-ended questions. For the former, the field has relied on traditional benchmarks to provide objective measures of progress, and we use many of them in our research today. For the latter, we believe evaluation is still in its infancy and will benefit from more input across a broad range of health professions.
Conclusion
Our research provides a glimpse of an exciting future where AI can broadly and deeply contribute to the medical domain. Med-Gemini demonstrates powerful multimodal capabilities that have the potential to assist in clinician, researcher, and patient workflows. While our findings are promising, and provide a step forward in the potential of AI models in medicine, it is important for these methods to be thoroughly tested in a variety of settings beyond traditional benchmarks. This is an essential step to ensure models like these are safe and reliable before being deployed in real-world situations involving patients or other users.
We’re excited about the potential of Med-Gemini in the medical domain and believe the broader researcher and developer community can play a role in continuing to improve the model’s safety and reliability, while also helping us better understand novel use cases. While Med-Gemini is not a product offering available for commercial use, one of the next steps is to explore its capabilities through collaboration with Google Cloud healthcare and life science customers and researchers. If your organization is interested in working with our research teams on this journey, please fill out the Research Partner Interest form here.
Acknowledgements
The research described here is joint work across teams at Google Research and Google DeepMind, including the authors of “Capabilities of Gemini Models in Medicine” and “Advancing Multimodal Medical Capabilities of Gemini” and partnering teams. We also thank Sami Lachgar, Lauren Winer and John Guilyard for their support with narratives and visuals. Finally, we are grateful to Michael Howell, James Manyika, Jeff Dean, Karen DeSalvo, Yossi Matias, Zoubin Ghahramani and Demis Hassabis for their support during the course of this project.