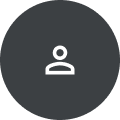
Grey Nearing
I am a Research Scientist on the Google Flood Forecasting team, and work on water-related topics.
Research Areas
Authored Publications
Sort By
Google
On the predictability of turbulent fluxes from land: PLUMBER2 MIP experimental description and preliminary results
Gab Abramowitz
Anna Ukkola
Sanaa Hobeichi
Jon Cranko Page
Mathew Lipson
Martin De Kauwe
Sam Green
Claire Brenner
Jonathan Frame
Martyn Clark
Martin Best
Peter Anthoni
Gabriele Arduini
Souhail Boussetta
Silvia Caldararu
Kyeungwoo Cho
Matthias Cuntz
David Fairbairn
Craig Ferguson
Hyungjun Kim
Yeonjoo Kim
Jürgen Knauer
David Lawrence
Xiangzhong Luo
Sergey Malyshev
Tomoko Nitta
Jerome Ogee
Keith Oleson
Catherine Ottlé
Phillipe Peylin
Patricia de Rosnay
Heather Rumbold
Bob Su
Nicolas Vuichard
Anthony Walker
Xiaoni Wang-Faivre
Yunfei Wang
Yijian Zeng
Hydrology and Earth Systems Sciences Discussions (2024)
A data-centric perspective on the information needed for hydrological uncertainty predictions
Andreas Auer
Martin Gauch
Frederik Kratzert
Sepp Hochreiter
Daniel Klotz
Hydrology and Earth System Sciences (2024)
Technical Note: The divide and measure nonconformity – how metrics can mislead when we evaluate on different data partitions
Daniel Klotz
Martin Gauch
Frederik Kratzert
Jakob Zscheischler
Hydrology and Earth System Sciences (2024)
An artificial neural network to estimate the foliar and ground cover input variables of the Rangeland Hydrology and Erosion Model
Mahmoud Saeedimoghaddam
David Goodrich
Mariano Hernandez
David Phillip Guertin
Loretta J. Metz
Guillermo Ponce-Campos
Haiyan Wei
Shea Burns
Sarah E. McCord
Mark A. Nearing
C. Jason Williams
Carrie-Ann Houdeshell
Mashrekur Rahman
Menberu B. Meles
Steve Barker
Journal of Hydrology (2024)
Caravan - A global community dataset for large-sample hydrology
Frederik Kratzert
Nans Addor
Tyler Erickson
Martin Gauch
Lukas Gudmundsson
Daniel Klotz
Sella Nevo
Guy Shalev
Scientific Data, 10 (2023), pp. 61
AI Increases Global Access to Reliable Flood Forecasts
Asher Metzger
Dana Weitzner
Frederik Kratzert
Guy Shalev
Martin Gauch
Sella Nevo
Shlomo Shenzis
Tadele Yednkachw Tekalign
Vusumuzi Dube
arXiv (2023)
On strictly enforced mass conservation constraints for modelling the Rainfall-Runoff process
Jonathan Frame
Frederik Kratzert
Hoshin Gupta
Paul Ullrich
Hydrological Processes (2023)