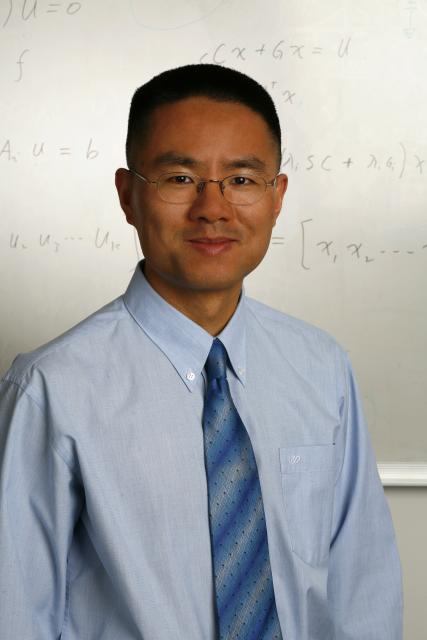
Zhenhai Zhu
Zhenhai did research in the area of electronic design automation (MIT, IBM TJ Watson, Cadence Research Lab) and parallel computing (NVidia) before joining Google. At Google he works on vision and language multi-modality and generic neural network design.
Research Areas
Authored Publications
Sort By
Preview abstract
Current image captioning methods are usually trained via (penalized) maximum
likelihood estimation. However, the log-likelihood score of a
caption does not correlate well with human assessments of quality.
Standard syntactic evaluation metrics,
such BLEU, METEOR and ROUGE, are also not well correlated.
The SPICE and CIDEr metrics are better correlated,
but have traditionally been hard to optimize for.
In this paper, we show how to use a policy gradient (PG) algorithm to directly optimize a
combination of SPICE and CIDEr (a combination we call SPIDEr): the
SPICE score ensures our captions are semantically faithful to the
image, and the CIDEr score ensures our captions are syntactically fluent.
The PG algorithm we propose improves on the prior MIXER approach,
by using Monte Carlo rollouts instead of mixing ML training with PG.
We show empirically that our algorithm leads to improved results
compared to MIXER. Finally, we shoow that using our PG algorithm
to optimize the novel SPIDEr
metric results in image captions that are strongly preferred by
human raters compared to captions generated by the same model but
trained using different objective functions.
View details