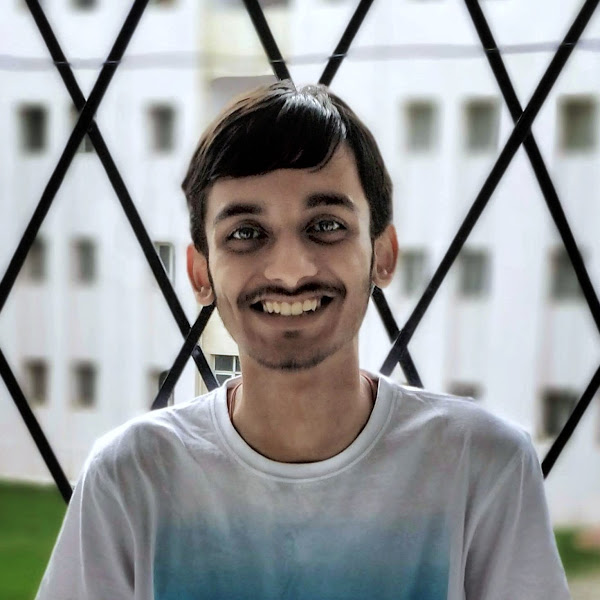
Vishal Venkat Batchu
I've been with Google Research India since 2020 working on the intersection of deep learning and earth observation sciences. In the past, I have worked on quantization and binarization of deep learning models at IIIT Hyderabad focusing on the reduction of model sizes and improving their efficiency. Currently at Google, I am working on geoscience tasks such as river segmentation and the estimation of soil moisture from satellite imagery. Link to my personal website
Research Areas
Authored Publications
Sort By
Cross-modal distillation for flood extent mapping
Shubhika Garg
Ben Feinstein
Shahar Timnat
Gideon Dror
Adi Gerzi Rosenthal
Environmental Data Science(2023)
Preview abstract
The increasing intensity and frequency of floods is one of the many consequences of our changing climate. In this work, we explore ML techniques that improve the flood detection module of an operational early flood warning system. Our method exploits an unlabelled dataset of paired multi-spectral and Synthetic Aperture Radar (SAR) imagery to reduce the labeling requirements of a purely supervised learning method. Prior works have used unlabelled data by creating weak labels out of them. However, from our experiments we noticed that such a model still ends up learning the label mistakes in those weak labels. Motivated by knowledge distillation and semi supervised learning, we explore the use of a teacher to train a student with the help of a small hand labelled dataset and a large unlabelled dataset. Unlike the conventional self distillation setup, we propose a cross modal distillation framework that transfers supervision from a teacher trained on richer modality (multi-spectral images) to a student model trained on SAR imagery. The trained models are then tested on the Sen1Floods11 dataset. Our model outperforms the Sen1Floods11 baseline model trained on the weak labeled SAR imagery by an absolute margin of 6.53% Intersection-over-Union (IoU) on the test split.
View details
Preview abstract
We develop a deep learning based convolutional-regression model that estimates the volumetric soil moisture content in the top ~5 cm. Input predictors include Sentinel-1 (active radar), Sentinel-2 (optical imagery), and SMAP (passive radar) as well as geophysical variables from SoilGrids and modelled soil moisture fields from GLDAS. The model was trained and evaluated on data from ~1300 in-situ sensors globally over the period 2015 - 2021 and obtained an average per-sensor correlation of 0.72 and ubRMSE of 0.0546. These results are benchmarked against 13 other soil moisture estimates at different locations, and an ablation study was used to identify important predictors.
View details
Cross Modal Distillation for Flood Extent Mapping
Shubhika Garg
Ben Feinstein
Shahar Timnat
Gideon Dror
Adi Gerzi Rosenthal
Tackling Climate Change with Machine Learning, NeurIPS 2022 Workshop
Preview abstract
The increasing intensity and frequency of floods is one of the many consequences of our changing climate. In this work, we explore ML techniques that improve the flood detection module of an operational early flood warning system. Our method exploits an unlabelled dataset of paired multi-spectral and Synthetic Aperture Radar (SAR) imagery to reduce the labeling requirements of a purely supervised learning method. Past attempts have used such unlabelled data by creating weak labels out of them, but end up learning the label mistakes in those weak labels. Motivated by knowledge distillation and semi supervised learning, we explore the use of a teacher to train a student with the help of a small hand labeled dataset and a large unlabelled dataset. Unlike the conventional self distillation setup, we propose a cross modal distillation framework that transfers supervision from a teacher trained on richer modality (multi-spectral images) to a student model trained on SAR imagery. The trained models are then tested on the Sen1Floods11 dataset. Our model outperforms the Sen1Floods11 SAR baselines by an absolute margin of 4.15% pixel wise Intersection-over-Union (IoU) on the test split.
View details