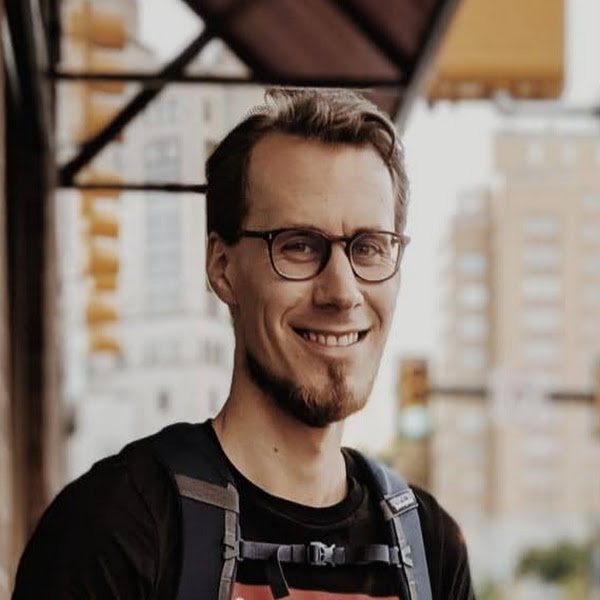
Thiemo Alldieck
Research Areas
Authored Publications
Sort By
Google
VLOGGER: Multimodal Diffusion for Embodied Avatar Synthesis
Enric Corona
Andrei Zanfir
Proceedings of the Computer Vision and Pattern Recognition Conference (CVPR) (2025)
SPHEAR: Spherical Head Registration for Complete Statistical 3D Modeling
Andrei Zanfir
Teodor Szente
Mihai Zanfir
International Conference on 3D Vision (2024)
DiffHuman: Probabilistic Photorealistic 3D Reconstruction of Humans
Akash Sengupta
Enric Corona
Andrei Zanfir
Proceedings of the IEEE/CVF Conference on Computer Vision and Pattern Recognition (CVPR) (2024)
Instant 3D Human Avatar Generation using Image Diffusion Models
Enric Corona
European Conference on Computer Vision (ECCV) (2024)
Score Distillation Sampling with Learned Manifold Corrective
European Conference on Computer Vision (ECCV) (2024)
Structured 3D Features for Reconstructing Controllable Avatars
Enric Corona
Mihai Zanfir
Andrei Zanfir
Proceedings of the IEEE/CVF Conference on Computer Vision and Pattern Recognition (CVPR) (2023)
DreamHuman: Animatable 3D Avatars from Text
Andrei Zanfir
Mihai Fieraru
Advances in Neural Information Processing Systems (2023)
Photorealistic Monocular 3D Reconstruction of Humans Wearing Clothing
Mihai Zanfir
Proceedings of the IEEE/CVF Conference on Computer Vision and Pattern Recognition (CVPR), IEEE (2022)
H-NeRF: Neural Radiance Fields for Rendering and Temporal Reconstruction of Humans in Motion
Hongyi Xu
Advances in Neural Information Processing Systems (NeurIPS) (2021)