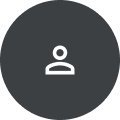
Richard Tucker
Research Areas
Authored Publications
Sort By
Simple and Effective Synthesis of Indoor 3D Scenes
Jing Yu Koh
Harsh Agrawal
Dhruv Batra
Honglak Lee
Yinfei Yang
Peter Anderson
AAAI(2023) (to appear)
Preview abstract
We study the problem of synthesizing immersive 3D indoor scenes from one or a few images. Our aim is to generate high-resolution images and videos from novel viewpoints, including viewpoints that extrapolate far beyond the input images while maintaining 3D consistency. Existing approaches are highly complex, with many separately trained stages and components. We propose a simple alternative: an image-to-image GAN that maps directly from reprojections of incomplete point clouds to full high-resolution RGB-D images. On the Matterport3D and RealEstate10K datasets, our approach significantly outperforms prior work when evaluated by humans, as well as on FID scores. Further, we show that our model is useful for generative data augmentation. A visionand-language navigation (VLN) agent trained with trajectories spatially-perturbed by our model improves success rate by up to 1.5% over a state of the art baseline on the mature R2R benchmark. Our code is publicly released to facilitate generative data augmentation and applications to downstream robotics and embodied AI tasks.
View details
Persistent Nature: A Generative Model of Unbounded 3D Worlds
Lucy Chai
Zhengqi Li
Phillip Isola
Computer Vision and Pattern Recognition (CVPR)(2023)
Preview abstract
Despite increasingly realistic image quality, recent 3D image generative models often operate on bounded domains with limited camera motions. We investigate the task of unconditionally synthesizing unbounded nature scenes, enabling arbitrarily large camera motion while maintaining a persistent 3D world model. Our scene representation consists of an extendable, planar scene layout grid, which can be rendered from arbitrary camera poses via a 3D decoder and volume rendering, and a panoramic skydome. Based on this representation, we learn a generative world model solely from single-view internet photos. Our method enables simulating long flights through 3D landscapes, while maintaining global scene consistency--for instance, returning to the starting point yields the same view of the scene. Our approach enables scene extrapolation beyond the fixed bounds of current 3D generative models, while also supporting a persistent, camera-independent world representation that stands in contrast to auto-regressive 3D prediction models.
View details
DynIBaR: Neural Dynamic Image-Based Rendering
Zhengqi Li
Qianqian Wang
Computer Vision and Pattern Recognition (CVPR)(2023)
Preview abstract
We address the problem of synthesizing novel views from a monocular video depicting complex dynamic scenes.
State-of-the-art methods based on temporally varying Neural Radiance Fields (aka \emph{dynamic NeRFs}) have shown impressive results on this task.
However, for long videos with complex object motions and uncontrolled camera trajectories, these methods can produce blurry or inaccurate renderings, hampering their use in real-world applications.
Rather than encoding a dynamic scene within the weights of MLPs, we present a new method that addresses these limitations by adopting a volumetric image-based rendering framework that synthesizes new viewpoints by aggregating features from nearby views in a scene-motion-aware manner.
Our system preserves the advantages for modeling complex scenes and view-dependent effects, but enables synthesizing photo-realistic novel views from long videos featuring complex scene dynamics with unconstrained camera trajectories.
We demonstrate significant improvements over state-of-the-art methods on dynamic scene datasets, and also apply our approach to in-the-wild videos with challenging camera and object motion, where prior methods fail to produce high-quality renderings.
View details
Deformable Sprites for Unsupervised Video Decomposition
Vickie Ye
Zhengqi Li
Angjoo Kanazawa
Computer Vision and Pattern Recognition (CVPR)(2022)
Preview abstract
We describe a method to extract persistent elements of a dynamic scene from an input video. We represent each scene element as a Deformable Sprite consisting of three components: 1) a 2D texture image for the entire video, 2) per-frame masks for the element, and 3) non-rigid deformations that map the texture image into each video frame. The resulting decomposition allows for applications such as consistent video editing. Deformable Sprites are a type of video auto-encoder model that is optimized on individual videos, and does not require training on a large dataset, nor does it rely on pre-trained models. Moreover, our method does not require object masks or other user input, and discovers moving objects of a wider variety than previous work. We evaluate our approach on standard video datasets and show qualitative results on a diverse array of Internet videos.
View details
Dimensions of Motion: Monocular Prediction through Flow Subspaces
Richard Strong Bowen*
Ramin Zabih
Proceedings of the International Conference on 3D Vision (3DV)(2022)
Preview abstract
We introduce a way to learn to estimate a scene representation from a single image by predicting a low-dimensional subspace of optical flow for each training example, which encompasses the variety of possible camera and object movement. Supervision is provided by a novel loss which measures the distance between this predicted flow subspace and an observed optical flow. This provides a new approach to learning scene representation tasks, such as monocular depth prediction or instance segmentation, in an unsupervised fashion using in-the-wild input videos without requiring camera poses, intrinsics, or an explicit multi-view stereo step. We evaluate our method in multiple settings, including an indoor depth prediction task where it achieves comparable performance to recent methods trained with more supervision.
View details
SLIDE: Single Image 3D Photography with Soft Layering and Depth-aware Inpainting
Varun Jampani*
Huiwen Chang*
Kyle Gregory Sargent
Dominik Philemon Kaeser
Ce Liu
ICCV 2021(2021)
Preview abstract
Single image 3D photography enables viewers to view a still image from novel viewpoints. Recent approaches for single-image view synthesis combine monocular depth network along with inpainting networks resulting in compelling novel view synthesis results. A drawback of these approaches is the use of hard layering making them not suitable to model intricate appearance effects such as matting. We present SLIDE, a modular and unified system for single image 3D photography that uses simple yet effective soft layering strategy to model appearance effects. In addition, we propose a novel depth-aware training of inpainting network suitable for 3D photography task. Extensive experimental analysis on 3 different view synthesis datasets in combination with user studies on in-the-wild image collections demonstrate the superior performance of our technique in comparison to existing strong baselines.
View details
Infinite Nature: Perpetual View Synthesis of Natural Scenes from a Single Image
Varun Jampani
Angjoo Kanazawa
International Conference on Computer Vision (ICCV)(2021)
Preview abstract
We introduce the problem of perpetual view generation—long-range generation of novel views corresponding to an arbitrarily long camera trajectory given a single image. This is a challenging problem that goes far beyond the capabilities of current view synthesis methods, which work for a limited range of viewpoints and quickly degenerate when presented with a large camera motion. Methods designed for video generation also have limited ability to produce long video sequences and are often agnostic to scene geometry. We take a hybrid approach that integrates both geometry and image synthesis in an iterative render, refine, and repeat framework, allowing for long-range generation that cover large distances after hundreds of frames. Our approach can be trained from a set of monocular video sequences without any manual annotation. We propose a dataset of aerial footage of natural coastal scenes, and compare our method with recent view synthesis and conditional video generation baselines, showing that it can generate plausible scenes for much longer time horizons over large camera trajectories compared to existing methods.
View details
KeypointDeformer: Unsupervised 3D Keypoint Discovery for Shape Control
Tomas Jakab
Jiajun Wu
Angjoo Kanazawa
Computer Vision and Pattern Recognition (CVPR)(2021)
Preview abstract
We present KeypointDeformer, a novel unsupervised method for shape control through automatically discovered 3D keypoints. Our approach produces intuitive and semantically consistent control of shape deformations. Moreover, our discovered 3D keypoints are consistent across object category instances despite large shape variations. Since our method is unsupervised, it can be readily deployed to new object categories without requiring expensive annotations for 3D keypoints and deformations.
View details
De-rendering the World’s Revolutionary Artefacts
Elliott Wu
Jiajun Wu
Angjoo Kanazawa
Computer Vision and Pattern Recognition (CVPR)(2021)
Preview abstract
Recent works have shown exciting results in unsupervised image de-rendering—learning to decompose 3D shape, appearance, and lighting from single-image collections without explicit supervision. However, many of these assume simplistic material and lighting models. We propose a method, termed RADAR (Revolutionary Artefact De-rendering And Re-rendering), that can recover environment illumination and surface materials from real single-image collections, relying neither on explicit 3D supervision, nor on multi-view or multi-light images. Specifically, we focus on rotationally symmetric artefacts that exhibit challenging surface properties including specular reflections, such as vases. We introduce a novel self-supervised albedo discriminator, which allows the model to recover plausible albedo without requiring any ground-truth during training. In conjunction with a shape reconstruction module exploiting rotational symmetry, we present an end-to-end learning framework that is able to de-render the world's revolutionary artefacts. We conduct experiments on a real vase dataset and demonstrate compelling decomposition results, allowing for applications including free-viewpoint rendering and relighting.
View details
Preview abstract
Neural implicit shape representations are an emerging paradigm that offers many potential benefits over conventional discrete representations, including memory efficiency at a high spatial resolution. Generalizing across shapes with such neural implicit representations amounts to learning priors over the respective function space and enables geometry reconstruction from partial or noisy observations. Existing generalization methods rely on conditioning a neural network on a low-dimensional latent code that is either regressed by an encoder or jointly optimized in the auto-decoder framework. Here, we formalize learning of a shape space as a meta-learning problem and leverage gradient-based meta-learning algorithms to solve this task. We demonstrate that this approach performs on par with auto-decoder based approaches while being an order of magnitude faster at test-time inference. We further demonstrate that the proposed gradient-based method outperforms encoder-decoder based methods that leverage pooling-based set encoders.
View details