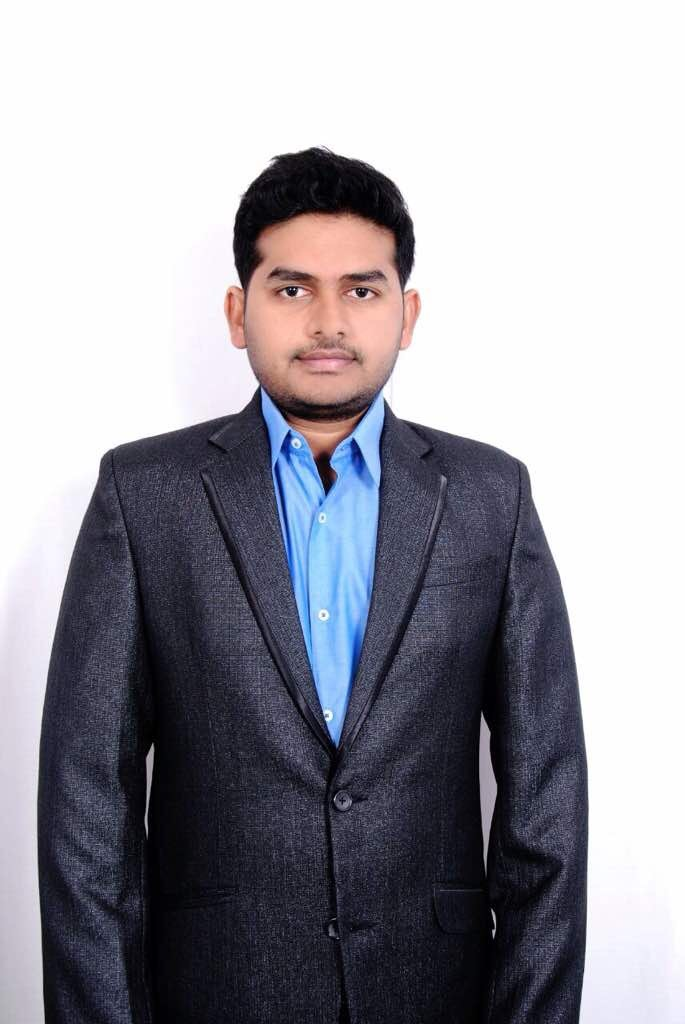
Rajesh Daruvuri
Rajesh Daruvuri is a seasoned Cloud Solution Consultant at Google, specializing in enterprise data solutions, AI, and business intelligence. With 15 years of experience, Rajesh brings a wealth of knowledge in advanced cloud computing, data management, and analytics. He is driven by a passion for creating innovative AI products that deliver exceptional user experiences and solve real-world problems.
Rajesh's expertise spans a wide range of technologies, including Google Cloud AI, Looker, BigQuery, Databricks, Azure Synapse, Snowflake, and Microsoft BI tools. He is adept at working with ETL processes, data warehousing, and analytics platforms like Power BI and Tableau. His recent focus has been on leveraging the power of Looker to help organizations derive actionable insights from their data. He has a proven track record of enhancing Looker's performance, training Looker admin teams, and ensuring seamless integration with other data platforms like BigQuery, Databricks, BigLake, and GenAI.
Rajesh is a results-oriented professional with a strong focus on building win-win partnerships and achieving business outcomes. He is eager to contribute his expertise to the academic community and foster the next generation of data and AI professionals.
Authored Publications
Sort By
Preview abstract
Cloud computing architectures are more scalable and economical which is the main reason that has contributed to its popularity. However, they bring their own set of challenges when it comes to workload scheduling and resource utilization because virtual machines (VM) and applications have to share different types of resources like servers, storage, etc. Historically, other strategies for workload balancing and resource management include manual configuration or simplistic heuristics that do not provide effective optimizations of resource usage and performance. In this technical brief, we propose an approach built on the use of unsupervised learning techniques to detect usage patterns perceptively and improve resource utilization, which corresponds to both optimal performance and automatically balanced workload among VMs. We are making use of clustering algorithms to cluster similar workloads and then resource allocation for each group based on demand. The point of this step is to use the resources more effectively so we do not run into resource exhaustion. We also integrate anomaly detection methods within our system for identifying and handling abnormal behavior by both monitoring and placing resources. We experiment with region traces from production workloads to demonstrate the benefits of our approach, showing marked improvements in workload balancing and resource utilization over current practices.
View details
Streamlining Workload Management in AI-Driven Cloud Architectures: A Comparative Algorithmic Approach
Pravallika Mannem
Kiran Kumar Patibandla
International Research Journal of Engineering and Technology, 11 (2024), pp. 113-121
Preview abstract
The use of artificial intelligence (AI) in cloud architectures has significantly increased processing efficiency and scale. However, with the development of complex algorithms and big data as well as surprisingly entered into our machine learning world; workload management becomes a significant issue in AI cloud computing. Existing workload management solutions are rule-based heuristics that may result in underutilization of resources and poor performance. For that, we present an algorithmic comparative approach to easing the burden of workload management for AI-driven cloud architectures. This is in contrast to executing a batch of tasks with different algorithms and comparing performance, cost, etc. We use ML methods to determine the best algorithm for our workload, and then deploy this in a self-contained binary that can switch between algorithms at runtime on an available resource. We validated our scheme with simulations, which demonstrates the capability of superior resource use and diminished completion time in comparison to rule-based schemes. When needed, flexibility and scalability allow you easier control over workloads that are subject to change or allocation. By simplifying AI-driven cloud workload management, the elasticity of their overall approach greatly enhances efficiency and scalability for those organizations looking to run even larger and take advantage of more complex workloads faster Tweet this Share on Facebook.
View details
Preview abstract
With the rapid development and widespread application of the Internet of Things (IoT), big data, and 5G networks, traditional cloud computing is increasingly unable to handle the massive amounts of data generated by network edge devices. In response, edge computing has emerged as a promising solution. However, due to its open nature, characterized by content awareness, real-time computing, and parallel processing, edge computing exacerbates the existing data security and privacy challenges already present in cloud environments. This paper outlines the research background of data security and privacy protection in edge computing and proposes a data-centric security framework. It provides a comprehensive review of the most recent advancements in key technologies related to data security, access control, identity authentication, and privacy protection that could be applicable to edge computing. The scalability and applicability of various approaches are analyzed and discussed in detail. Additionally, several practical instances of edge computing that are currently in use are introduced. Finally, the paper highlights key research directions and offers recommendations for future study.
View details
Reinforcement Deep Learning Approach for Multi-User Task Offloading in Edge-Cloud Joint Computing Systems
Kiran Kumar Patibandla
International Journal Of Research In Electronics And Computer Engineering, 11 (2023), pp. 8
Preview abstract
To enhance system utility in multi-user task offloading, a reinforcement deep learning-based task offloading scheme within an edge-cloud joint computing framework is proposed. This scheme leverages deep reinforcement learning to optimize the collaborative allocation of resources between edge and cloud, improving decision-making for task offloading modes. A reinforcement learning algorithm based on submodular theory is developed to fully utilize both computing and communication resources in edge and cloud environments. Simulation results show that the proposed scheme significantly reduces execution delays and energy consumption. Even under resource-constrained conditions with multiple users, the system maintains stable performance and high efficiency.
View details