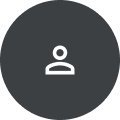
Nikos Kolotouros
Nikos Kolotouros is a Research Scientist at Google Zurich. Nikos obtained his PhD in Computer and Information Science from the University of Pennsylvania advised by Prof. Kostas Daniilidis. His work focused on 3D Computer Vision and more specifically on model-based 3D human reconstruction. Before that, he studied Electrical and Computer Engineering at the National Technical University of Athens where he worked with Prof. Petros Maragos.
Research Areas
Authored Publications
Google Publications
Other Publications
Sort By
DiffHuman: Probabilistic Photorealistic 3D Reconstruction of Humans
Akash Sengupta
Enric Corona
Andrei Zanfir
Proceedings of the IEEE/CVF Conference on Computer Vision and Pattern Recognition (CVPR) (2024)
Preview abstract
We present DiffHuman, a probabilistic method for photorealistic 3D human reconstruction from a single RGB image. Despite the ill-posed nature of this problem, most methods are deterministic and output a single solution, often resulting in a lack of geometric detail and blurriness in unseen or uncertain regions. In contrast, DiffHuman predicts a distribution over 3D reconstructions conditioned on an image, which allows us to sample multiple detailed 3D avatars that are consistent with the input image. DiffHuman is implemented as a conditional diffusion model that denoises partial observations of an underlying pixel-aligned 3D representation. In testing, we can sample a 3D shape by iteratively denoising renderings of the predicted intermediate representation. Further, we introduce an additional generator neural network that approximates rendering with considerably reduced runtime (55x speed up), resulting in a novel dual-branch diffusion framework. We evaluate the effectiveness of our approach through various experiments. Our method can produce diverse, more detailed reconstructions for the parts of the person not observed in the image, and has competitive performance for the surface reconstruction of visible parts.
View details
DreamHuman: Animatable 3D Avatars from Text
Andrei Zanfir
Mihai Fieraru
Advances in Neural Information Processing Systems (2023)
Preview abstract
We present DreamHuman, a method to generate realistic animatable 3D human avatar models solely from textual descriptions. Recent text-to-3D methods have made considerable strides in generation, but are still lacking in important aspects. Control and often spatial resolution remain limited, existing methods produce fixed rather than animated 3D human models, and anthropometric consistency for complex structures like people remains a challenge. DreamHuman connects large text-to-image synthesis models, neural radiance fields, and statistical human body models in a novel modeling and optimization framework. This makes it possible to generate dynamic 3D human avatars with high-quality textures and learned, instance-specific, surface deformations. We demonstrate that our method is capable to generate a wide variety of animatable, realistic 3D human models from text. Our 3D models have diverse appearance, clothing, skin tones and body shapes, and significantly outperform both generic text-to-3D approaches and previous text-based 3D avatar generators in visual fidelity.
View details
No Results Found