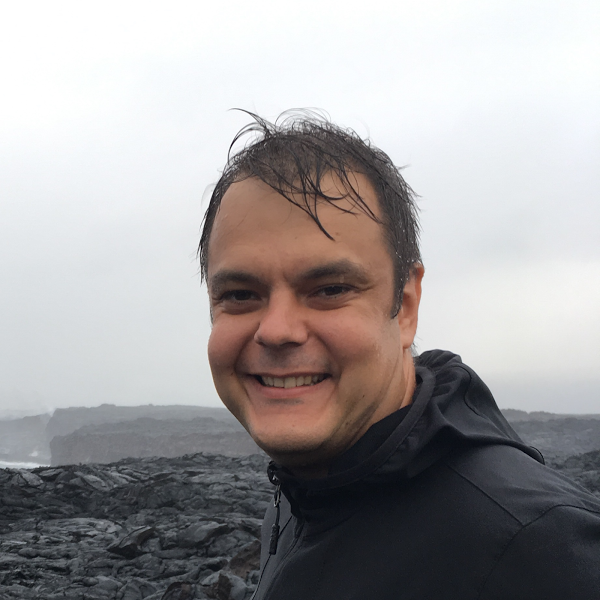
Mykhaylo Andriluka
Mykhaylo Andriluka is a senior research scientist at Google. Previously he has
been a visiting assistant professor at Stanford University and a junior research
group leader at the Max-Planck Institute for Informatics. Mykhaylo holds a master's degree
in mathematics and PhD degree in computer science from TU Darmstadt. His
research interests are in various aspects of visual human analysis such as
articulated pose estimation and tracking, multi-person tracking, people
detection and activity recognition. Mykhaylos' work has received the best paper
awards at AMDO'12 and BMVC'12, and was a winning entry in the MOTChallenge'16
competition on multi-person tracking. Mykhaylo is also a co-creator of several
popular benchmarks for articulated human pose estimation.
Research Areas
Authored Publications