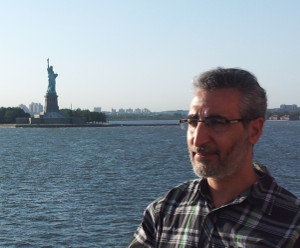
Mohammed Attia
Research Areas
Authored Publications
Sort By
Google
Statistical Measures for Readability Assessment
Yo Ehara
Younes Samih
Proceedings of the Joint 3rd International Conference on Natural Language Processing for Digital Humanities and 8th International Workshop on Computational Linguistics for Uralic Languages (2023)
Effective Multi Dialectal Arabic POS Tagging
Kareem Darwish
Hamdy Mubarak
Younes Samih
Ahmed Abdelali
Lluís Màrquez
Mohamed Eldesouki
Laura Kallmeyer
Natural Language Engineering (NLE) (2020)
QC-GO Submission for MADAR Shared Task: Arabic Fine-Grained Dialect Identification
Ahmed Abdelali
Hamdy Mubarak
Kareem Darwish
Mohamed Eldesouki
Younes Samih
MADAR Shared on Dialect Identification -- ACL 2019 (2019)
POS Tagging for Improving Code-Switching Identification in Arabic
Ahmed Abdelali
Ali Elkahky
Hamdy Mubarak
Kareem Darwish
Younes Samih
Workshop on Arabic Natural Language Processing -- ACL 2019, Florence, Italy (2019)
Segmentation for Domain Adaptation in Arabic
Ali Elkahky
Workshop on Arabic Natural Language Processing -- ACL 2019, Florence, Italy (2019)
Multilingual Multi-class Sentiment Classification Using Convolutional Neural Networks
Younes Samih
Ali Elkahky
Laura Kallmeyer
Proceedings of the Eleventh International Conference on Language Resources and Evaluation (LREC 2018), European Language Resources Association (ELRA), Miyazaki, Japan (2018), pp. 635-640
Multi-Dialect Arabic POS Tagging: A CRF Approach
Kareem Darwish
Hamdy Mubarak
Ahmed Abdelali
Mohamed Eldesouki
Younes Samih
Randah Alharbi
Walid Magdy
Laura Kallmeyer
Proceedings of the Eleventh International Conference on Language Resources and Evaluation (LREC 2018), European Language Resources Association (ELRA), Miyazaki, Japan (2018), pp. 93-98
GHH at SemEval-2018 Task 10: Discovering Discriminative Attributes in Distributional Semantics
Younes Samih
Manaal Faruqui
Wolfgang Maier
SemEval 2018 Task 10 on Capturing Discriminative Attributes (2018)
Diacritization of Moroccan and Tunisian Arabic Dialects: A CRF Approach
Kareem Darwish
Ahmed Abdelali
Hamdy Mubarak
Younes Samih
The 3rd Workshop on Open-Source Arabic Corpora and Processing Tools in the Proceedings of the Eleventh International Conference on Language Resources and Evaluation (LREC 2018), European Language Resources Association (ELRA), Miyazaki, Japan (2018)
GHHT at CALCS 2018: Named Entity Recognition for Dialectal Arabic Using Neural Networks
Younes Samih
Wolfgang Maier
Third Workshop on Computational Approaches to Linguistic Code-switching in ACL 2018 (2018)