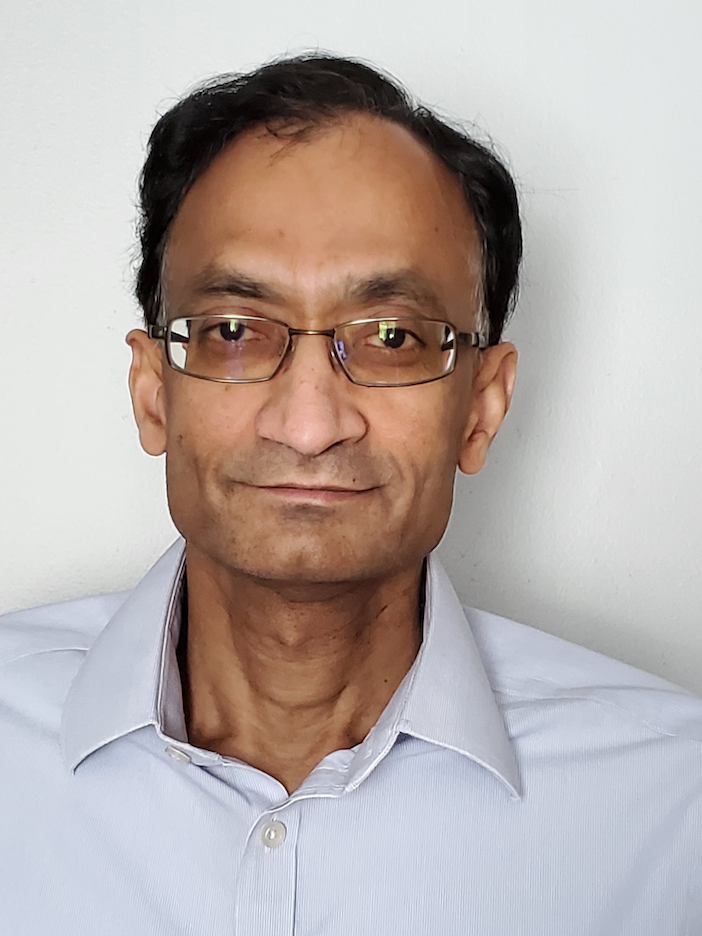
Kishore Papineni
Kishore leads the Coauthor team whose objective is cross-lingual cross-modal access to dynamically organized information. His team hopes to make content consumption or creation a richer experience by surfacing relevant and diverse information from the web, possibly synthesized dynamically from across different sources or types of content such as text, images, charts, and videos. Coauthor team powers the web content suggestions in Google Docs when users are writing a document, and is working on additional content recommendation applications. His work at Google includes veracity of information on the web, depth of discourse on a topic in a document, drift of discourse on a topic on the web, identifying concepts peculiar to a collection of documents and relationships among the concepts, and identifying different perspectives in content. His past work was in the areas of automatic control theory, natural language understanding, dialog management, machine translation, and display advertisements. Prior to joining Google, he led machine learning at Yahoo! Research and machine translation at IBM Research. He is a coauthor of the BLEU metric for automatic evaluation of machine translation quality (awarded 2018 Test-of-Time Paper on Computational Linguistics). He was a founding Editor-in-Chief of ACM Transactions on Speech and Language Processing from 2003-2007.
Authored Publications
Sort By
Google
Promises and Pitfalls of Generative Masked Language Modeling: Theoretical Framework and Practical Guidelines
Yuchen Li
Alexandre Kirchmeyer
Aashay Mehta
Yilong Qin
Andrej Risteski
International Conference on Machine Learning (2024) (to appear)