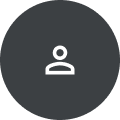
Keith Peters
I'm a roll up your sleeves and get dirty engineer who builds and implements. Sometimes what I build and implement is interesting for others.
Authored Publications
Sort By
Google
F1 Query: Declarative Querying at Scale
Bart Samwel
Ben Handy
Jason Govig
Chanjun Yang
Daniel Tenedorio
Felix Weigel
David G Wilhite
Jiacheng Yang
Jun Xu
Jiexing Li
Zhan Yuan
Qiang Zeng
Ian Rae
Anurag Biyani
Andrew Harn
Yang Xia
Andrey Gubichev
Amr El-Helw
Orri Erling
Allen Yan
Mohan Yang
Yiqun Wei
Thanh Do
Colin Zheng
Somayeh Sardashti
Ahmed Aly
Divy Agrawal
Shivakumar Venkataraman
PVLDB (2018), pp. 1835-1848