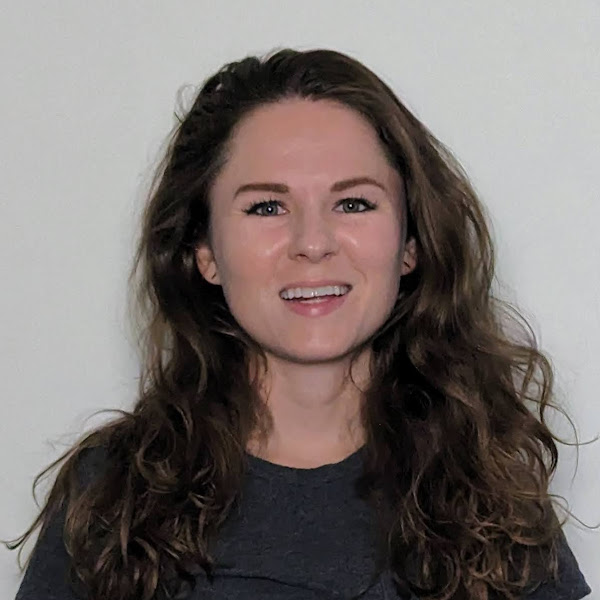
Kara Vaillancourt
Kara Vaillancourt is a researcher at Google working on foundation models, LLMs, and on device ML. More information can be found at my Google Scholar page.
Authored Publications
Sort By
Smartphone-based Hard Braking Events Detection at Scale for Road Safety Services
David Racz
Julie Michelman
Stefan Mellem
Paul C. Eastham
Bradley Green
Charles Robert Armstrong
Shawn O'Banion
Feng Guo
Transportation Research Part C: Emerging Technologies(2022)
Preview abstract
Road crashes are the sixth leading cause of lost disability-adjusted life-years (DALYs) worldwide. One major challenge in traffic safety research is the sparsity of crashes, which makes it difficult to achieve a fine-grain understanding of crash causations and predict future crash risk in a timely manner. Hard-braking events have been widely used as a safety surrogate due to their relatively high prevalence and ease of detection with embedded vehicle sensors. As an alternative to using sensors fixed in vehicles, this paper presents a scalable approach for detecting hard-braking events using the kinematics data collected from smartphone sensors. We train a Transformer-based machine learning model for hard-braking event detection using concurrent sensor readings from smartphones and vehicle sensors from drivers who connect their phone to the vehicle while navigating in Google Maps. The detection model shows superior performance with a 0.83 Area under the Precision-Recall Curve (PR-AUC), which is 3.8×better than a GPS speed-based heuristic model, and 166.6×better than an accelerometer-based heuristic model. The detected hard-braking events are strongly correlated with crashes from publicly available datasets, supporting their use as a safety surrogate. In addition, we conduct model fairness and selection bias evaluation to ensure that the safety benefits are equally shared. The developed methodology can benefit many safety applications such as identifying safety hot spots at road network level, evaluating the safety of new user interfaces, as well as using routing to improve traffic safety.
View details
Residual Adapters for Parameter-Efficient ASR Adaptation to Atypical and Accented Speech
Vicky Zayats
Dirk Padfield
Proceedings of EMNLP 2021(2021)
Preview abstract
Automatic Speech Recognition (ASR) systems are often optimized to work best for speakers with canonical speech patterns. Unfortunately, these systems perform poorly when tested on atypical speech and heavily accented speech. It has previously been shown that personalization through model fine-tuning substantially improves performance. However, maintaining such large models per speaker is costly and difficult to scale. We show that by adding a relatively small number of extra parameters to the encoder layers via so-called residual adapter, we can achieve similar adaptation gains compared to model fine-tuning, while only updating a tiny fraction (less than 0.5%) of the model parameters. We demonstrate this on two speech adaptation tasks (atypical and accented speech) and for two state-of-the-art ASR architectures.
View details