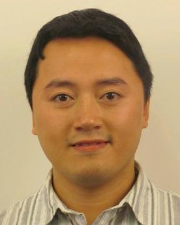
Jing (David) Dai
My interests include:
Spatial/Spatio-temporal Data Management and Analytics, Concurrency Control, Sustainability Management, Intelligent Transportation Systems, Geographic Information Systems.
Authored Publications
Sort By
Google
Multimodal Storytelling via Generative Adversarial Imitation Learning
Zhiqian Chen
Xuchao Zhang
Arnold Boedihardjo
Chang-Tien Lu
The Twenty-Sixth International Joint Conference on Artificial Intelligence (2017), pp. 3967-3973
Trendi: Tracking Stories in News and Microblogs via Emerging, Evolving and Fading Topics
Xuchao Zhang
Liang Zhao
Zhiqian Chen
Arnold Boedihardjo
Chang-Tien Lu
IEEE BigData Conference 2017
Unsupervised Spatial Event Detection in Targeted Domains with Applications to Civil Unrest Modeling
Liang Zhao
Feng Cheng
Ting Hua
Chang-Tien Lu
Naren Ramakrishnan
PLOS ONE, 9 (2014), pp. 1-12
Student-t based Robust Spatio-Temporal Prediction
Yang Chen
Feng Chen
T. Charles Clancy
Yao-Jan Wu
IEEE 12th International Conference on Data Mining, IEEE, Brussels, Belgium (2012), pp. 151-160
An Integrated Framework for Spatio-Temporal-Textual Search and Mining
Bingsheng Wang
Haili Dong
Arnold Boedihardjo
Chang-Tien Lu
Harland Yu
Ing-Ray Chen
20th ACM SIGSPATIAL International Conference on Advances in Geographic Information Systems (ACM SIGSPATIAL GIS 2012), ACM, 2 Penn Plaza, Suite 701, New York, NY 10121, pp. 570-573