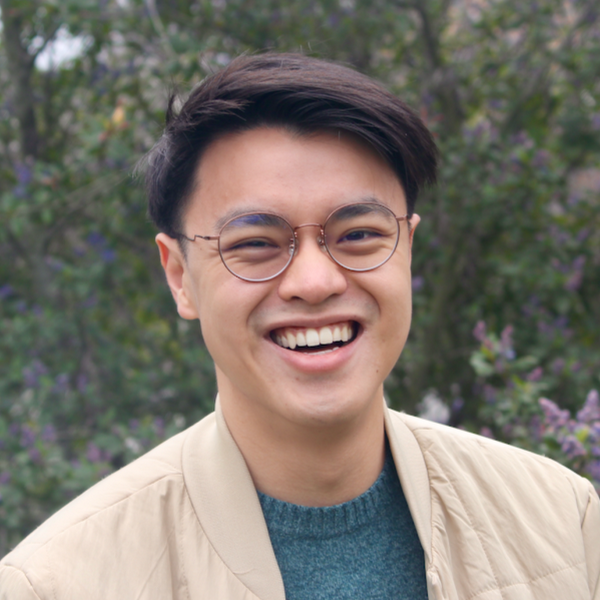
Jack H Ching
Health economics and outcomes researcher with expertise in wearables and digital health interventions, population health management, and cost-effectiveness modeling.
Research Areas
Authored Publications
Sort By
Efficacy of a Multimodal Digital Behavior Change Intervention on Lifestyle Behavior, Cardiometabolic Biomarkers, and Medical Expenditure: Protocol for a Randomized Controlled Trial
Sakeina Howard-Wilson
Sherri Gentile
Martin Ho
Alex Garcia
Didem Ayturk
Peter Lazar
Nova Hammerquist
David McManus
Bruce Barton
Steven B. Bird
John Moore
Apurv Soni
JMIR Research Protocols (2024)
Preview abstract
Background:
The US Preventive Services Task Force recommends providers offer individualized healthy behavior interventions for all adults, independent of their risk of cardiovascular disease. While strong evidence exists to support disease-specific programs designed to improve multiple lifestyle behaviors, approaches to adapting these interventions for a broader population are not well established. Digital behavior change interventions (DBCIs) hold promise as a more generalizable and scalable approach to overcome the resource and time limitations that traditional behavioral intervention programs face, especially within an occupational setting.
Objective:
We aimed to evaluate the efficacy of a multimodal DBCI on (1) self-reported behaviors of physical activity, nutrition, sleep, and mindfulness; (2) cardiometabolic biomarkers; and (3) chronic disease–related medical expenditure.
Methods:
We conducted a 2-arm randomized controlled trial for 12 months among employees of an academic health care facility in the United States. The intervention arm received a scale, a smartphone app, an activity tracker, a video library for healthy behavior recommendations, and an on-demand health coach. The control arm received standard employer-provided health and wellness benefits. The primary outcomes of the study included changes in self-reported lifestyle behaviors, cardiometabolic biomarkers, and chronic disease–related medical expenditure. We collected health behavior data via baseline and quarterly web-based surveys, biometric measures via clinic visits at baseline and 12 months, and identified relevant costs through claims datasets.
Results:
A total of 603 participants were enrolled and randomized to the intervention (n=300, 49.8%) and control arms (n=303, 50.2%). The average age was 46.7 (SD 11.2) years, and the majority of participants were female (80.3%, n=484), White (85.4%, n=504), and non-Hispanic (90.7%, n=547), with no systematic differences in baseline characteristics observed between the study arms. We observed retention rates of 86.1% (n=519) for completing the final survey and 77.9% (n=490) for attending the exit visit.
Conclusions:
This study represents the largest and most comprehensive evaluation of DBCIs among participants who were not selected based on their underlying condition to assess its impact on behavior, cardiometabolic biomarkers, and medical expenditure.
View details
Preview abstract
Background: Physical activity levels worldwide have declined over recent decades, with the average number of daily steps decreasing steadily since 1995. Given that physical inactivity is a major modifiable risk factor for chronic disease and mortality, increasing the level of physical activity is a clear opportunity to improve population health on a broad scale. The current study aims to assess the cost-effectiveness and budget impact of a Fitbit-based intervention among healthy, but insufficiently active, adults to quantify the potential clinical and economic value for a commercially insured population in the U.S. Methods: An economic model was developed to compare physical activity, health outcomes, costs, and quality-adjusted life-years (QALYs) associated with usual care and a Fitbit-based intervention that consists of a consumer wearable device alongside goal setting and feedback features provided in a companion software application. Improvement in physical activity was measured in terms of mean daily step count. The effects of increased daily step count were characterized as reduced short-term healthcare costs and decreased incidence of chronic diseases with corresponding improvement in health utility and reduced disease costs. Published literature, standardized costing resources, and data from a National Institutes of Health-funded research program were utilized. Cost-effectiveness and budget impact analyses were performed for a hypothetical cohort of middle-aged adults. Results: The base case cost-effectiveness results found the Fitbit intervention to be dominant (less costly and more effective) compared to usual care. Discounted 15-year incremental costs and QALYs were -$1,257 and 0.011, respectively. In probabilistic analyses, the Fitbit intervention was dominant in 93% of simulations and either dominant or cost-effective (defined as less than $150,000/QALY gained) in 99.4% of simulations. For budget impact analyses conducted from the perspective of a U.S. Commercial payer, the Fitbit intervention was estimated to save approximately $6.5-million dollars over 2 years and $8.5-million dollars over 5 years for a cohort of 8,000 participants. Although the economic analysis results were very robust, the short-term healthcare cost savings were the most uncertain in this population and warrant further research. Conclusions: There is abundant evidence documenting the benefits of wearable activity trackers when used to increase physical activity as measured by daily step counts. Our research provides additional health economic evidence supporting implementation of wearable-based interventions to improve population health and offers compelling support for payers to consider including wearable-based physical activity interventions as part of a comprehensive portfolio of preventive health offerings for their insured populations.
View details
Sleep patterns and risk of chronic disease as measured by long-term monitoring with commercial wearable devices in the All of Us Research Program
Neil S. Zheng
Jeffrey Annis
Hiral Master
Lide Han
Karla Gleichauf
Melody Nasser
Peyton Coleman
Stacy Desine
Douglas M. Ruderfer
John Hernandez
Logan D. Schneider
Evan L. Brittain
Nature Medicine (2024)
Preview abstract
Poor sleep health is associated with increased all-cause mortality and incidence of many chronic conditions. Previous studies have relied on cross-sectional and self-reported survey data or polysomnograms, which have limitations with respect to data granularity, sample size and longitudinal information. Here, using objectively measured, longitudinal sleep data from commercial wearable devices linked to electronic health record data from the All of Us Research Program, we show that sleep patterns, including sleep stages, duration and regularity, are associated with chronic disease incidence. Of the 6,785 participants included in this study, 71% were female, 84% self-identified as white and 71% had a college degree; the median age was 50.2 years (interquartile range = 35.7, 61.5) and the median sleep monitoring period was 4.5 years (2.5, 6.5). We found that rapid eye movement sleep and deep sleep were inversely associated with the odds of incident atrial fibrillation and that increased sleep irregularity was associated with increased odds of incident obesity, hyperlipidemia, hypertension, major depressive disorder and generalized anxiety disorder. Moreover, J-shaped associations were observed between average daily sleep duration and hypertension, major depressive disorder and generalized anxiety disorder. These findings show that sleep stages, duration and regularity are all important factors associated with chronic disease development and may inform evidence-based recommendations on healthy sleeping habits.
View details
Cost-utility analysis of deep learning and trained human graders for diabetic retinopathy screening in a nationwide program
Attasit Srisubat
Kankamon Kittrongsiri
Sermsiri Sangroongruangsri
Chalida Khemvaranan
Jacqueline Shreibati
John Hernandez
Fred Hersch
Prut Hanutsaha
Varis Ruamviboonsuk
Saowalak Turongkaravee
Rajiv Raman
Dr. Paisan Raumviboonsuk
Ophthalmology (2023)
Preview abstract
Introduction
Deep learning (DL) for screening diabetic retinopathy (DR) has the potential to address limited healthcare resources by enabling expanded access to healthcare. However, there is still limited health economic evaluation, particularly in low- and middle-income countries, on this subject to aid decision-making for DL adoption.
Methods
In the context of a middle-income country (MIC), using Thailand as a model, we constructed a decision tree-Markov hybrid model to estimate lifetime costs and outcomes of Thailand’s national DR screening program via DL and trained human graders (HG). We calculated the incremental cost-effectiveness ratio (ICER) between the two strategies. Sensitivity analyses were performed to probe the influence of modeling parameters.
Results
From a societal perspective, screening with DL was associated with a reduction in costs of ~ US$ 2.70, similar quality-adjusted life-years (QALY) of + 0.0043, and an incremental net monetary benefit of ~ US$ 24.10 in the base case. In sensitivity analysis, DL remained cost-effective even with a price increase from US$ 1.00 to US$ 4.00 per patient at a Thai willingness-to-pay threshold of ~ US$ 4.997 per QALY gained. When further incorporating recent findings suggesting improved compliance to treatment referral with DL, our analysis models effectiveness benefits of ~ US$ 20 to US$ 50 depending on compliance.
Conclusion
DR screening using DL in an MIC using Thailand as a model may result in societal cost-savings and similar health outcomes compared with HG. This study may provide an economic rationale to expand DL-based DR screening in MICs as an alternative solution for limited availability of skilled human resources for primary screening, particularly in MICs with similar prevalence of diabetes and low compliance to referrals for treatment.
View details